Meta-analysis
Part of a series on |
Research |
---|
Philosophy portal |
Meta-analysis is a method of synthesis of quantitative data from multiple independent studies addressing a common research question. An important part of this method involves computing a combined effect size across all of the studies. As such, this statistical approach involves extracting effect sizes and variance measures from various studies. By combining these effect sizes the statistical power is improved and can resolve uncertainties or discrepancies found in individual studies. Meta-analyses are integral in supporting research grant proposals, shaping treatment guidelines, and influencing health policies. They are also pivotal in summarizing existing research to guide future studies, thereby cementing their role as a fundamental methodology in metascience. Meta-analyses are often, but not always, important components of a systematic review.
History
[edit]The term "meta-analysis" was coined in 1976 by the statistician Gene Glass,[1][2] who stated "Meta-analysis refers to the analysis of analyses".[3] Glass's work aimed at describing aggregated measures of relationships and effects.[4] While Glass is credited with authoring the first modern meta-analysis, a paper published in 1904 by the statistician Karl Pearson in the British Medical Journal[5] collated data from several studies of typhoid inoculation and is seen as the first time a meta-analytic approach was used to aggregate the outcomes of multiple clinical studies.[6][7] Numerous other examples of early meta-analyses can be found including occupational aptitude testing,[8][9] and agriculture.[10]
The first model meta-analysis was published in 1978 on the effectiveness of psychotherapy outcomes by Mary Lee Smith and Gene Glass.[2][11] After publication of their article there was pushback on the usefulness and validity of meta-analysis as a tool for evidence synthesis. The first example of this was by Han Eysenck who in a 1978 article in response to the work done by Mary Lee Smith and Gene Glass called meta-analysis an "exercise in mega-silliness".[12][13] Later Eysenck would refer to meta-analysis as "statistical alchemy".[14] Despite these criticisms the use of meta-analysis has only grown since its modern introduction. By 1991 there were 334 published meta-analyses;[13] this number grew to 9,135 by 2014.[1][15]
The field of meta-analysis expanded greatly since the 1970s and touches multiple disciplines including psychology, medicine, and ecology.[1] Further the more recent creation of evidence synthesis communities has increased the cross pollination of ideas, methods, and the creation of software tools across disciplines.[16][17][18]
Literature Search
[edit]One of the most important steps of a meta-analysis is data collection. For an efficient database search, appropriate keywords and search limits need to be identified.[19] The use of Boolean operators and search limits can assist the literature search.[20][21] A number of databases are available (e.g., PubMed, Embase, PsychInfo), however, it is up to the researcher to choose the most appropriate sources for their research area.[22] Indeed, many scientists use duplicate search terms within two or more databases to cover multiple sources.[23] The reference lists of eligible studies can also be searched for eligible studies (i.e., snowballing).[24] The initial search may return a large volume of studies.[24] Quite often, the abstract or the title of the manuscript reveals that the study is not eligible for inclusion, based on the pre-specified criteria.[22] These studies can be discarded. However, if it appears that the study may be eligible (or even if there is some doubt) the full paper can be retained for closer inspection. The references lists of eligible articles can also be searched for any relevant articles.[23][25] These search results need to be detailed in a PRIMSA flow diagram[26] which details the flow of information through all stages of the review. Thus, it is important to note how many studies were returned after using the specified search terms and how many of these studies were discarded, and for what reason.[22] The search terms and strategy should be specific enough for a reader to reproduce the search.[27] The date range of studies, along with the date (or date period) the search was conducted should also be provided.[28]
A data collection form provides a standardized means of collecting data from eligible studies.[29] For a meta-analysis of correlational data, effect size information is usually collected as Pearson's r statistic.[30][31] Partial correlations are often reported in research, however, these may inflate relationships in comparison to zero-order correlations.[32] Moreover, the partialed out variables will likely vary from study-to-study. As a consequence, many meta-analyses exclude partial correlations from their analysis.[22] As a final resort, plot digitizers can be used to scrape data points from scatterplots (if available) for the calculation of Pearson's r.[33][34] Data reporting important study characteristics that may moderate effects, such as the mean age of participants, should also be collected.[35] A measure of study quality can also be included in these forms to assess the quality of evidence from each study.[36] There are more than 80 tools available to assess the quality and risk of bias in observational studies reflecting the diversity of research approaches between fields.[36][37][38] These tools usually include an assessment of how dependent variables were measured, appropriate selection of participants, and appropriate control for confounding factors. Other quality measures that may be more relevant for correlational studies include sample size, psychometric properties, and reporting of methods.[22]
A final consideration is whether to include studies from the gray literature,[39] which is defined as research that has not been formally published.[40] This type of literature includes conference abstracts,[41] dissertations,[42] and pre-prints.[43] While the inclusion of gray literature reduces the risk of publication bias, the methodological quality of the work is often (but not always) lower than formally published work.[44][45] Reports from conference proceedings, which are the most common source of gray literature,[46] are poorly reported[47] and data in the subsequent publication is often inconsistent, with differences observed in almost 20% of published studies.[48]
Methods and assumptions
[edit]Approaches
[edit]In general, two types of evidence can be distinguished when performing a meta-analysis: individual participant data (IPD), and aggregate data (AD).[49] The aggregate data can be direct or indirect.
AD is more commonly available (e.g. from the literature) and typically represents summary estimates such as odds ratios[50] or relative risks.[51] This can be directly synthesized across conceptually similar studies using several approaches. On the other hand, indirect aggregate data measures the effect of two treatments that were each compared against a similar control group in a meta-analysis. For example, if treatment A and treatment B were directly compared vs placebo in separate meta-analyses, we can use these two pooled results to get an estimate of the effects of A vs B in an indirect comparison as effect A vs Placebo minus effect B vs Placebo.
IPD evidence represents raw data as collected by the study centers. This distinction has raised the need for different meta-analytic methods when evidence synthesis is desired, and has led to the development of one-stage and two-stage methods.[52] In one-stage methods the IPD from all studies are modeled simultaneously whilst accounting for the clustering of participants within studies. Two-stage methods first compute summary statistics for AD from each study and then calculate overall statistics as a weighted average of the study statistics. By reducing IPD to AD, two-stage methods can also be applied when IPD is available; this makes them an appealing choice when performing a meta-analysis. Although it is conventionally believed that one-stage and two-stage methods yield similar results, recent studies have shown that they may occasionally lead to different conclusions.[53][54]
Statistical models for aggregate data
[edit]Fixed effect model
[edit]
The fixed effect model provides a weighted average of a series of study estimates.[55] The inverse of the estimates' variance is commonly used as study weight, so that larger studies tend to contribute more than smaller studies to the weighted average.[56] Consequently, when studies within a meta-analysis are dominated by a very large study, the findings from smaller studies are practically ignored.[57] Most importantly, the fixed effects model assumes that all included studies investigate the same population, use the same variable and outcome definitions, etc.[58] This assumption is typically unrealistic as research is often prone to several sources of heterogeneity.[59][60]
If we start with a collection of independent effect size estimates, each estimate a corresponding effect size we can assume that where denotes the observed effect in the -th study, the corresponding (unknown) true effect, is the sampling error, and . Therefore, the ’s are assumed to be unbiased and normally distributed estimates of their corresponding true effects. The sampling variances (i.e., values) are assumed to be known.[61]
Random effects model
[edit]Most meta-analyses are based on sets of studies that are not exactly identical in their methods and/or the characteristics of the included samples.[61] Differences in the methods and sample characteristics may introduce variability (“heterogeneity”) among the true effects.[61][62] One way to model the heterogeneity is to treat it as purely random. The weight that is applied in this process of weighted averaging with a random effects meta-analysis is achieved in two steps:[63]
- Step 1: Inverse variance weighting
- Step 2: Un-weighting of this inverse variance weighting by applying a random effects variance component (REVC) that is simply derived from the extent of variability of the effect sizes of the underlying studies.
This means that the greater this variability in effect sizes (otherwise known as heterogeneity), the greater the un-weighting and this can reach a point when the random effects meta-analysis result becomes simply the un-weighted average effect size across the studies. At the other extreme, when all effect sizes are similar (or variability does not exceed sampling error), no REVC is applied and the random effects meta-analysis defaults to simply a fixed effect meta-analysis (only inverse variance weighting).
The extent of this reversal is solely dependent on two factors:[64]
- Heterogeneity of precision
- Heterogeneity of effect size
Since neither of these factors automatically indicates a faulty larger study or more reliable smaller studies, the re-distribution of weights under this model will not bear a relationship to what these studies actually might offer. Indeed, it has been demonstrated that redistribution of weights is simply in one direction from larger to smaller studies as heterogeneity increases until eventually all studies have equal weight and no more redistribution is possible.[64] Another issue with the random effects model is that the most commonly used confidence intervals generally do not retain their coverage probability above the specified nominal level and thus substantially underestimate the statistical error and are potentially overconfident in their conclusions.[65][66] Several fixes have been suggested[67][68] but the debate continues on.[66][69] A further concern is that the average treatment effect can sometimes be even less conservative compared to the fixed effect model[70] and therefore misleading in practice. One interpretational fix that has been suggested is to create a prediction interval around the random effects estimate to portray the range of possible effects in practice.[71] However, an assumption behind the calculation of such a prediction interval is that trials are considered more or less homogeneous entities and that included patient populations and comparator treatments should be considered exchangeable[72] and this is usually unattainable in practice.
There are many methods used to estimate between studies variance with restricted maximum likelihood estimator being the least prone to bias and one of the most commonly used.[73] Several advanced iterative techniques for computing the between studies variance exist including both maximum likelihood and restricted maximum likelihood methods and random effects models using these methods can be run with multiple software platforms including Excel,[74] Stata,[75] SPSS,[76] and R.[61]
Most meta-analyses include between 2 and 4 studies and such a sample is more often than not inadequate to accurately estimate heterogeneity. Thus it appears that in small meta-analyses, an incorrect zero between study variance estimate is obtained, leading to a false homogeneity assumption. Overall, it appears that heterogeneity is being consistently underestimated in meta-analyses and sensitivity analyses in which high heterogeneity levels are assumed could be informative.[77] These random effects models and software packages mentioned above relate to study-aggregate meta-analyses and researchers wishing to conduct individual patient data (IPD) meta-analyses need to consider mixed-effects modelling approaches.[78]/
Quality effects model
[edit]Doi and Thalib originally introduced the quality effects model.[79] They[80] introduced a new approach to adjustment for inter-study variability by incorporating the contribution of variance due to a relevant component (quality) in addition to the contribution of variance due to random error that is used in any fixed effects meta-analysis model to generate weights for each study. The strength of the quality effects meta-analysis is that it allows available methodological evidence to be used over subjective random effects, and thereby helps to close the damaging gap which has opened up between methodology and statistics in clinical research. To do this a synthetic bias variance is computed based on quality information to adjust inverse variance weights and the quality adjusted weight of the ith study is introduced.[79] These adjusted weights are then used in meta-analysis. In other words, if study i is of good quality and other studies are of poor quality, a proportion of their quality adjusted weights is mathematically redistributed to study i giving it more weight towards the overall effect size. As studies become increasingly similar in terms of quality, re-distribution becomes progressively less and ceases when all studies are of equal quality (in the case of equal quality, the quality effects model defaults to the IVhet model – see previous section). A recent evaluation of the quality effects model (with some updates) demonstrates that despite the subjectivity of quality assessment, the performance (MSE and true variance under simulation) is superior to that achievable with the random effects model.[81][82] This model thus replaces the untenable interpretations that abound in the literature and a software is available to explore this method further.[83]
Network meta-analysis methods
[edit]
Indirect comparison meta-analysis methods (also called network meta-analyses, in particular when multiple treatments are assessed simultaneously) generally use two main methodologies.[84][85] First, is the Bucher method[86] which is a single or repeated comparison of a closed loop of three-treatments such that one of them is common to the two studies and forms the node where the loop begins and ends. Therefore, multiple two-by-two comparisons (3-treatment loops) are needed to compare multiple treatments. This methodology requires that trials with more than two arms have two arms only selected as independent pair-wise comparisons are required. The alternative methodology uses complex statistical modelling to include the multiple arm trials and comparisons simultaneously between all competing treatments. These have been executed using Bayesian methods, mixed linear models and meta-regression approaches.[citation needed]
Bayesian framework
[edit]Specifying a Bayesian network meta-analysis model involves writing a directed acyclic graph (DAG) model for general-purpose Markov chain Monte Carlo (MCMC) software such as WinBUGS.[87] In addition, prior distributions have to be specified for a number of the parameters, and the data have to be supplied in a specific format.[87] Together, the DAG, priors, and data form a Bayesian hierarchical model. To complicate matters further, because of the nature of MCMC estimation, overdispersed starting values have to be chosen for a number of independent chains so that convergence can be assessed.[88] Recently, multiple R software packages were developed to simplify the model fitting (e.g., metaBMA[89] and RoBMA[90]) and even implemented in statistical software with graphical user interface (GUI): JASP. Although the complexity of the Bayesian approach limits usage of this methodology, recent tutorial papers are trying to increase accessibility of the methods.[91][92] Methodology for automation of this method has been suggested[87] but requires that arm-level outcome data are available, and this is usually unavailable. Great claims are sometimes made for the inherent ability of the Bayesian framework to handle network meta-analysis and its greater flexibility. However, this choice of implementation of framework for inference, Bayesian or frequentist, may be less important than other choices regarding the modeling of effects[93] (see discussion on models above).
Frequentist multivariate framework
[edit]On the other hand, the frequentist multivariate methods involve approximations and assumptions that are not stated explicitly or verified when the methods are applied (see discussion on meta-analysis models above). For example, the mvmeta package for Stata enables network meta-analysis in a frequentist framework.[94] However, if there is no common comparator in the network, then this has to be handled by augmenting the dataset with fictional arms with high variance, which is not very objective and requires a decision as to what constitutes a sufficiently high variance.[87] The other issue is use of the random effects model in both this frequentist framework and the Bayesian framework. Senn advises analysts to be cautious about interpreting the 'random effects' analysis since only one random effect is allowed for but one could envisage many.[93] Senn goes on to say that it is rather naıve, even in the case where only two treatments are being compared to assume that random-effects analysis accounts for all uncertainty about the way effects can vary from trial to trial. Newer models of meta-analysis such as those discussed above would certainly help alleviate this situation and have been implemented in the next framework.
Generalized pairwise modelling framework
[edit]An approach that has been tried since the late 1990s is the implementation of the multiple three-treatment closed-loop analysis. This has not been popular because the process rapidly becomes overwhelming as network complexity increases. Development in this area was then abandoned in favor of the Bayesian and multivariate frequentist methods which emerged as alternatives. Very recently, automation of the three-treatment closed loop method has been developed for complex networks by some researchers[74] as a way to make this methodology available to the mainstream research community. This proposal does restrict each trial to two interventions, but also introduces a workaround for multiple arm trials: a different fixed control node can be selected in different runs. It also utilizes robust meta-analysis methods so that many of the problems highlighted above are avoided. Further research around this framework is required to determine if this is indeed superior to the Bayesian or multivariate frequentist frameworks. Researchers willing to try this out have access to this framework through a free software.[83]
Tailored meta-analysis
[edit]Another form of additional information comes from the intended setting. If the target setting for applying the meta-analysis results is known then it may be possible to use data from the setting to tailor the results thus producing a 'tailored meta-analysis'.,[95][96] This has been used in test accuracy meta-analyses, where empirical knowledge of the test positive rate and the prevalence have been used to derive a region in Receiver Operating Characteristic (ROC) space known as an 'applicable region'. Studies are then selected for the target setting based on comparison with this region and aggregated to produce a summary estimate which is tailored to the target setting.
Aggregating IPD and AD
[edit]Meta-analysis can also be applied to combine IPD and AD. This is convenient when the researchers who conduct the analysis have their own raw data while collecting aggregate or summary data from the literature. The generalized integration model (GIM)[97] is a generalization of the meta-analysis. It allows that the model fitted on the individual participant data (IPD) is different from the ones used to compute the aggregate data (AD). GIM can be viewed as a model calibration method for integrating information with more flexibility.
Validation of meta-analysis results
[edit]The meta-analysis estimate represents a weighted average across studies and when there is heterogeneity this may result in the summary estimate not being representative of individual studies. Qualitative appraisal of the primary studies using established tools can uncover potential biases,[98][99] but does not quantify the aggregate effect of these biases on the summary estimate. Although the meta-analysis result could be compared with an independent prospective primary study, such external validation is often impractical. This has led to the development of methods that exploit a form of leave-one-out cross validation, sometimes referred to as internal-external cross validation (IOCV).[100] Here each of the k included studies in turn is omitted and compared with the summary estimate derived from aggregating the remaining k- 1 studies. A general validation statistic, Vn based on IOCV has been developed to measure the statistical validity of meta-analysis results.[101] For test accuracy and prediction, particularly when there are multivariate effects, other approaches which seek to estimate the prediction error have also been proposed.[102]
Challenges
[edit]A meta-analysis of several small studies does not always predict the results of a single large study.[103] Some have argued that a weakness of the method is that sources of bias are not controlled by the method: a good meta-analysis cannot correct for poor design or bias in the original studies.[104] This would mean that only methodologically sound studies should be included in a meta-analysis, a practice called 'best evidence synthesis'.[104] Other meta-analysts would include weaker studies, and add a study-level predictor variable that reflects the methodological quality of the studies to examine the effect of study quality on the effect size.[105] However, others have argued that a better approach is to preserve information about the variance in the study sample, casting as wide a net as possible, and that methodological selection criteria introduce unwanted subjectivity, defeating the purpose of the approach.[106] More recently, and under the influence of a push for open practices in science, tools to develop "crowd-sourced" living meta-analyses that are updated by communities of scientists [107][108] in hopes of making all the subjective choices more explicit.
Publication bias: the file drawer problem
[edit]
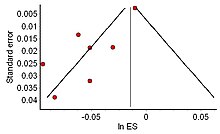
Another potential pitfall is the reliance on the available body of published studies, which may create exaggerated outcomes due to publication bias,[109] as studies which show negative results or insignificant results are less likely to be published.[110] For example, pharmaceutical companies have been known to hide negative studies[111] and researchers may have overlooked unpublished studies such as dissertation studies or conference abstracts that did not reach publication.[112] This is not easily solved, as one cannot know how many studies have gone unreported.[113][114]
This file drawer problem characterized by negative or non-significant results being tucked away in a cabinet, can result in a biased distribution of effect sizes thus creating a serious base rate fallacy, in which the significance of the published studies is overestimated, as other studies were either not submitted for publication or were rejected. This should be seriously considered when interpreting the outcomes of a meta-analysis.[113][115]
The distribution of effect sizes can be visualized with a funnel plot which (in its most common version) is a scatter plot of standard error versus the effect size.[116] It makes use of the fact that the smaller studies (thus larger standard errors) have more scatter of the magnitude of effect (being less precise) while the larger studies have less scatter and form the tip of the funnel. If many negative studies were not published, the remaining positive studies give rise to a funnel plot in which the base is skewed to one side (asymmetry of the funnel plot). In contrast, when there is no publication bias, the effect of the smaller studies has no reason to be skewed to one side and so a symmetric funnel plot results. This also means that if no publication bias is present, there would be no relationship between standard error and effect size.[117] A negative or positive relation between standard error and effect size would imply that smaller studies that found effects in one direction only were more likely to be published and/or to be submitted for publication.
Apart from the visual funnel plot, statistical methods for detecting publication bias have also been proposed.[114] These are controversial because they typically have low power for detection of bias, but also may make false positives under some circumstances.[118] For instance small study effects (biased smaller studies), wherein methodological differences between smaller and larger studies exist, may cause asymmetry in effect sizes that resembles publication bias. However, small study effects may be just as problematic for the interpretation of meta-analyses, and the imperative is on meta-analytic authors to investigate potential sources of bias.[119]
The problem of publication bias is not trivial as it is suggested that 25% of meta-analyses in the psychological sciences may have suffered from publication bias.[120] However, low power of existing tests and problems with the visual appearance of the funnel plot remain an issue, and estimates of publication bias may remain lower than what truly exists.
Most discussions of publication bias focus on journal practices favoring publication of statistically significant findings. However, questionable research practices, such as reworking statistical models until significance is achieved, may also favor statistically significant findings in support of researchers' hypotheses.[121][122]
Problems related to studies not reporting non-statistically significant effects
[edit]Studies often do not report the effects when they do not reach statistical significance.[123] For example, they may simply say that the groups did not show statistically significant differences, without reporting any other information (e.g. a statistic or p-value).[124] Exclusion of these studies would lead to a situation similar to publication bias, but their inclusion (assuming null effects) would also bias the meta-analysis.
Problems related to the statistical approach
[edit]Other weaknesses are that it has not been determined if the statistically most accurate method for combining results is the fixed, IVhet, random or quality effect models, though the criticism against the random effects model is mounting because of the perception that the new random effects (used in meta-analysis) are essentially formal devices to facilitate smoothing or shrinkage and prediction may be impossible or ill-advised.[125] The main problem with the random effects approach is that it uses the classic statistical thought of generating a "compromise estimator" that makes the weights close to the naturally weighted estimator if heterogeneity across studies is large but close to the inverse variance weighted estimator if the between study heterogeneity is small. However, what has been ignored is the distinction between the model we choose to analyze a given dataset, and the mechanism by which the data came into being.[126] A random effect can be present in either of these roles, but the two roles are quite distinct. There's no reason to think the analysis model and data-generation mechanism (model) are similar in form, but many sub-fields of statistics have developed the habit of assuming, for theory and simulations, that the data-generation mechanism (model) is identical to the analysis model we choose (or would like others to choose). As a hypothesized mechanisms for producing the data, the random effect model for meta-analysis is silly and it is more appropriate to think of this model as a superficial description and something we choose as an analytical tool – but this choice for meta-analysis may not work because the study effects are a fixed feature of the respective meta-analysis and the probability distribution is only a descriptive tool.[126]
Problems arising from agenda-driven bias
[edit]The most severe fault in meta-analysis often occurs when the person or persons doing the meta-analysis have an economic, social, or political agenda such as the passage or defeat of legislation.[127] People with these types of agendas may be more likely to abuse meta-analysis due to personal bias. For example, researchers favorable to the author's agenda are likely to have their studies cherry-picked while those not favorable will be ignored or labeled as "not credible". In addition, the favored authors may themselves be biased or paid to produce results that support their overall political, social, or economic goals in ways such as selecting small favorable data sets and not incorporating larger unfavorable data sets. The influence of such biases on the results of a meta-analysis is possible because the methodology of meta-analysis is highly malleable.[128]
A 2011 study done to disclose possible conflicts of interests in underlying research studies used for medical meta-analyses reviewed 29 meta-analyses and found that conflicts of interests in the studies underlying the meta-analyses were rarely disclosed. The 29 meta-analyses included 11 from general medicine journals, 15 from specialty medicine journals, and three from the Cochrane Database of Systematic Reviews. The 29 meta-analyses reviewed a total of 509 randomized controlled trials (RCTs). Of these, 318 RCTs reported funding sources, with 219 (69%) receiving funding from industry (i.e. one or more authors having financial ties to the pharmaceutical industry). Of the 509 RCTs, 132 reported author conflict of interest disclosures, with 91 studies (69%) disclosing one or more authors having financial ties to industry. The information was, however, seldom reflected in the meta-analyses. Only two (7%) reported RCT funding sources and none reported RCT author-industry ties. The authors concluded "without acknowledgment of COI due to industry funding or author industry financial ties from RCTs included in meta-analyses, readers' understanding and appraisal of the evidence from the meta-analysis may be compromised."[129]
For example, in 1998, a US federal judge found that the United States Environmental Protection Agency had abused the meta-analysis process to produce a study claiming cancer risks to non-smokers from environmental tobacco smoke (ETS) with the intent to influence policy makers to pass smoke-free–workplace laws.[130][131][132]
Comparability and validity of included studies
[edit]Meta-analysis may often not be a substitute for an adequately powered primary study, particularly in the biological sciences.[133]
Heterogeneity of methods used may lead to faulty conclusions.[134] For instance, differences in the forms of an intervention or the cohorts that are thought to be minor or are unknown to the scientists could lead to substantially different results, including results that distort the meta-analysis' results or are not adequately considered in its data. Vice versa, results from meta-analyses may also make certain hypothesis or interventions seem nonviable and preempt further research or approvals, despite certain modifications – such as intermittent administration, personalized criteria and combination measures – leading to substantially different results, including in cases where such have been successfully identified and applied in small-scale studies that were considered in the meta-analysis.[citation needed] Standardization, reproduction of experiments, open data and open protocols may often not mitigate such problems, for instance as relevant factors and criteria could be unknown or not be recorded.[citation needed]
There is a debate about the appropriate balance between testing with as few animals or humans as possible and the need to obtain robust, reliable findings. It has been argued that unreliable research is inefficient and wasteful and that studies are not just wasteful when they stop too late but also when they stop too early. In large clinical trials, planned, sequential analyses are sometimes used if there is considerable expense or potential harm associated with testing participants.[135] In applied behavioural science, "megastudies" have been proposed to investigate the efficacy of many different interventions designed in an interdisciplinary manner by separate teams.[136] One such study used a fitness chain to recruit a large number participants. It has been suggested that behavioural interventions are often hard to compare [in meta-analyses and reviews], as "different scientists test different intervention ideas in different samples using different outcomes over different time intervals", causing a lack of comparability of such individual investigations which limits "their potential to inform policy".[136]
Weak inclusion standards lead to misleading conclusions
[edit]Meta-analyses in education are often not restrictive enough in regards to the methodological quality of the studies they include. For example, studies that include small samples or researcher-made measures lead to inflated effect size estimates.[137] However, this problem also troubles meta-analysis of clinical trials. The use of different quality assessment tools (QATs) lead to including different studies and obtaining conflicting estimates of average treatment effects.[138][139]
Applications in modern science
[edit]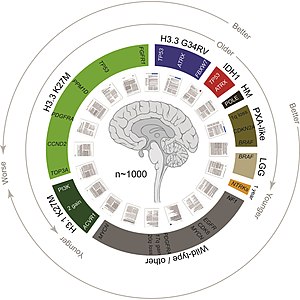
Modern statistical meta-analysis does more than just combine the effect sizes of a set of studies using a weighted average. It can test if the outcomes of studies show more variation than the variation that is expected because of the sampling of different numbers of research participants. Additionally, study characteristics such as measurement instrument used, population sampled, or aspects of the studies' design can be coded and used to reduce variance of the estimator (see statistical models above). Thus some methodological weaknesses in studies can be corrected statistically. Other uses of meta-analytic methods include the development and validation of clinical prediction models, where meta-analysis may be used to combine individual participant data from different research centers and to assess the model's generalisability,[140][141] or even to aggregate existing prediction models.[142]
Meta-analysis can be done with single-subject design as well as group research designs.[143] This is important because much research has been done with single-subject research designs.[144] Considerable dispute exists for the most appropriate meta-analytic technique for single subject research.[145]
Meta-analysis leads to a shift of emphasis from single studies to multiple studies. It emphasizes the practical importance of the effect size instead of the statistical significance of individual studies. This shift in thinking has been termed "meta-analytic thinking". The results of a meta-analysis are often shown in a forest plot.
Results from studies are combined using different approaches. One approach frequently used in meta-analysis in health care research is termed 'inverse variance method'. The average effect size across all studies is computed as a weighted mean, whereby the weights are equal to the inverse variance of each study's effect estimator. Larger studies and studies with less random variation are given greater weight than smaller studies. Other common approaches include the Mantel–Haenszel method[146] and the Peto method.[147]
Seed-based d mapping (formerly signed differential mapping, SDM) is a statistical technique for meta-analyzing studies on differences in brain activity or structure which used neuroimaging techniques such as fMRI, VBM or PET.
Different high throughput techniques such as microarrays have been used to understand Gene expression. MicroRNA expression profiles have been used to identify differentially expressed microRNAs in particular cell or tissue type or disease conditions or to check the effect of a treatment. A meta-analysis of such expression profiles was performed to derive novel conclusions and to validate the known findings.[148]
Meta-analysis of whole genome sequencing studies provides an attractive solution to the problem of collecting large sample sizes for discovering rare variants associated with complex phenotypes. Some methods have been developed to enable functionally informed rare variant association meta-analysis in biobank-scale cohorts using efficient approaches for summary statistic storage.[149]
Sweeping meta-analyses can also be used to estimate a network of effects. This allows researchers to examine patterns in the fuller panorama of more accurately estimated results and draw conclusions that consider the broader context (e.g., how personality-intelligence relations vary by trait family).[150]
See also
[edit]Sources
[edit] This article incorporates text by Daniel S. Quintana available under the CC BY 4.0 license.
This article incorporates text by Wolfgang Viechtbauer available under the CC BY 3.0 license.
References
[edit]- ^ a b c Shadish WR, Lecy JD (September 2015). "The meta-analytic big bang". Research Synthesis Methods. 6 (3): 246–264. doi:10.1002/jrsm.1132. PMID 26212600. S2CID 5416879.
- ^ a b Glass GV (September 2015). "Meta-analysis at middle age: a personal history". Research Synthesis Methods. 6 (3): 221–231. doi:10.1002/jrsm.1133. PMID 26355796. S2CID 30083129.
- ^ Glass GV (1976). "Primary, secondary, and meta-analysis of research". Educational Researcher. 5 (10): 3–8. doi:10.3102/0013189X005010003. S2CID 3185455.
- ^ Hunt, Morton (1997). How science takes stock: the story of meta-analysis (1st ed.). New York, New York, United States of America: Russell Sage Foundation.
- ^ "Report on Certain Enteric Fever Inoculation Statistics". British Medical Journal. 2 (2288): 1243–1246. November 1904. doi:10.1136/bmj.2.2288.1243. PMC 2355479. PMID 20761760.
- ^ Nordmann AJ, Kasenda B, Briel M (9 March 2012). "Meta-analyses: what they can and cannot do". Swiss Medical Weekly. 142: w13518. doi:10.4414/smw.2012.13518. PMID 22407741.
- ^ O'Rourke K (December 2007). "An historical perspective on meta-analysis: dealing quantitatively with varying study results". Journal of the Royal Society of Medicine. 100 (12): 579–582. doi:10.1177/0141076807100012020. PMC 2121629. PMID 18065712.
- ^ Ghiselli, E. E. (1955). The measurement of occupational aptitude. University of California Publications in Psychology, 8, 101–216.
- ^ Ghiselli, Edwin E. (1973). "The Validity of Aptitude Tests in Personnel Selection". Personnel Psychology. 26 (4): 461–477. doi:10.1111/j.1744-6570.1973.tb01150.x. ISSN 0031-5826.
- ^ Yates, F.; Cochran, W. G. (1938). "The analysis of groups of experiments". The Journal of Agricultural Science. 28 (4): 556–580. doi:10.1017/S0021859600050978. ISSN 0021-8596. S2CID 86619593.
- ^ Smith, Mary L.; Glass, Gene V. (1977). "Meta-analysis of psychotherapy outcome studies". American Psychologist. 32 (9): 752–760. doi:10.1037/0003-066X.32.9.752. ISSN 1935-990X. PMID 921048. S2CID 43326263.
- ^ Eysenck, H. J. (1978). "An exercise in mega-silliness". American Psychologist. 33 (5): 517. doi:10.1037/0003-066X.33.5.517.a. ISSN 1935-990X.
- ^ a b Sharpe, Donald; Poets, Sarena (2020). "Meta-analysis as a response to the replication crisis". Canadian Psychology / Psychologie Canadienne. 61 (4): 377–387. doi:10.1037/cap0000215. ISSN 1878-7304. S2CID 225384392.
- ^ Eysenck, H.J. (1995). "Meta-analysis or best-evidence synthesis?". Journal of Evaluation in Clinical Practice. 1 (1): 29–36. doi:10.1111/j.1365-2753.1995.tb00005.x. ISSN 1356-1294. PMID 9238555.
- ^ Ioannidis, John P.A. (2016). "The Mass Production of Redundant, Misleading, and Conflicted Systematic Reviews and Meta-analyses". The Milbank Quarterly. 94 (3): 485–514. doi:10.1111/1468-0009.12210. ISSN 0887-378X. PMC 5020151. PMID 27620683.
- ^ Vandvik PO, Brandt L (July 2020). "Future of Evidence Ecosystem Series: Evidence ecosystems and learning health systems: why bother?". Journal of Clinical Epidemiology. 123: 166–170. doi:10.1016/j.jclinepi.2020.02.008. PMID 32145365. S2CID 212629387.
- ^ Cartabellotta A, Tilson JK (June 2019). "The ecosystem of evidence cannot thrive without efficiency of knowledge generation, synthesis, and translation". Journal of Clinical Epidemiology. 110: 90–95. doi:10.1016/j.jclinepi.2019.01.008. PMID 30708174. S2CID 73415319.
- ^ Haddaway NR, Bannach-Brown A, Grainger MJ, Hamilton WK, Hennessy EA, Keenan C, et al. (June 2022). "The evidence synthesis and meta-analysis in R conference (ESMARConf): levelling the playing field of conference accessibility and equitability". Systematic Reviews. 11 (1): 113. doi:10.1186/s13643-022-01985-6. PMC 9164457. PMID 35659294.
- ^ Grames, Eliza M.; Stillman, Andrew N.; Tingley, Morgan W.; Elphick, Chris S. (2019). Freckleton, Robert (ed.). "An automated approach to identifying search terms for systematic reviews using keyword co-occurrence networks". Methods in Ecology and Evolution. 10 (10): 1645–1654. Bibcode:2019MEcEv..10.1645G. doi:10.1111/2041-210X.13268. ISSN 2041-210X.
- ^ Sood, Amit; Erwin, Patricia J.; Ebbert, Jon O. (2004). "Using Advanced Search Tools on PubMed for Citation Retrieval". Mayo Clinic Proceedings. 79 (10): 1295–1300. doi:10.4065/79.10.1295. PMID 15473412.
- ^ Vincent, Beatriz; Vincent, Maurice; Ferreira, Carlos Gil (1 March 2006). "Making PubMed Searching Simple: Learning to Retrieve Medical Literature Through Interactive Problem Solving". The Oncologist. 11 (3): 243–251. doi:10.1634/theoncologist.11-3-243. ISSN 1083-7159. PMID 16549808.
- ^ a b c d e Quintana, Daniel S. (8 October 2015). "From pre-registration to publication: a non-technical primer for conducting a meta-analysis to synthesize correlational data". Frontiers in Psychology. 6: 1549. doi:10.3389/fpsyg.2015.01549. ISSN 1664-1078. PMC 4597034. PMID 26500598.
- ^ a b Bramer, Wichor M.; Giustini, Dean; de Jonge, Gerdien B.; Holland, Leslie; Bekhuis, Tanja (2016). "De-duplication of database search results for systematic reviews in EndNote". Journal of the Medical Library Association. 104 (3): 240–243. doi:10.3163/1536-5050.104.3.014. ISSN 1536-5050. PMC 4915647. PMID 27366130.
- ^ a b Horsley, Tanya; Dingwall, Orvie; Sampson, Margaret (10 August 2011). Cochrane Methodology Review Group (ed.). "Checking reference lists to find additional studies for systematic reviews". Cochrane Database of Systematic Reviews. 2011 (8): MR000026. doi:10.1002/14651858.MR000026.pub2. PMC 7388740. PMID 21833989.
- ^ Bramer, Wichor M.; De Jonge, Gerdien B.; Rethlefsen, Melissa L.; Mast, Frans; Kleijnen, Jos (4 October 2018). "A systematic approach to searching: an efficient and complete method to develop literature searches". Journal of the Medical Library Association. 106 (4): 531–541. doi:10.5195/jmla.2018.283. ISSN 1558-9439. PMC 6148622. PMID 30271302.
- ^ Moher, David; Tetzlaff, Jennifer; Tricco, Andrea C; Sampson, Margaret; Altman, Douglas G (27 March 2007). Clarke, Mike (ed.). "Epidemiology and Reporting Characteristics of Systematic Reviews". PLOS Medicine. 4 (3): e78. doi:10.1371/journal.pmed.0040078. ISSN 1549-1676. PMC 1831728. PMID 17388659.
- ^ Lakens, Daniël; Hilgard, Joe; Staaks, Janneke (2016). "On the reproducibility of meta-analyses: six practical recommendations". BMC Psychology. 4 (1): 24. doi:10.1186/s40359-016-0126-3. ISSN 2050-7283. PMC 4886411. PMID 27241618.
- ^ Nguyen, Phi-Yen; McKenzie, Joanne E.; Hamilton, Daniel G.; Moher, David; Tugwell, Peter; Fidler, Fiona M.; Haddaway, Neal R.; Higgins, Julian P. T.; Kanukula, Raju; Karunananthan, Sathya; Maxwell, Lara J.; McDonald, Steve; Nakagawa, Shinichi; Nunan, David; Welch, Vivian A. (2023). "Systematic reviewers' perspectives on sharing review data, analytic code, and other materials: A survey". Cochrane Evidence Synthesis and Methods. 1 (2). doi:10.1002/cesm.12008. ISSN 2832-9023.
- ^ Page, Matthew J.; Altman, Douglas G.; Shamseer, Larissa; McKenzie, Joanne E.; Ahmadzai, Nadera; Wolfe, Dianna; Yazdi, Fatemeh; Catalá-López, Ferrán; Tricco, Andrea C.; Moher, David (2018). "Reproducible research practices are underused in systematic reviews of biomedical interventions". Journal of Clinical Epidemiology. 94: 8–18. doi:10.1016/j.jclinepi.2017.10.017. PMID 29113936.
- ^ Aloe, Ariel M. (2014). "An Empirical Investigation of Partial Effect Sizes in Meta-Analysis of Correlational Data". The Journal of General Psychology. 141 (1): 47–64. doi:10.1080/00221309.2013.853021. ISSN 0022-1309. PMID 24838020.
- ^ Tracz, Susan M.; Elmore, Patricia B.; Pohlmann, John T. (1992). "Correlational Meta-Analysis: Independent and Nonindependent Cases". Educational and Psychological Measurement. 52 (4): 879–888. doi:10.1177/0013164492052004007. ISSN 0013-1644.
- ^ Cramer, Duncan (2003). "A Cautionary Tale of Two Statistics: Partial Correlation and Standardized Partial Regression". The Journal of Psychology. 137 (5): 507–511. doi:10.1080/00223980309600632. ISSN 0022-3980. PMID 14629080. S2CID 37557674.
- ^ Gross, Arnd; Schirm, Sibylle; Scholz, Markus (2014). "Ycasd– a tool for capturing and scaling data from graphical representations". BMC Bioinformatics. 15 (1): 219. doi:10.1186/1471-2105-15-219. ISSN 1471-2105. PMC 4085079. PMID 24965054.
- ^ Cliche, Mathieu; Rosenberg, David; Madeka, Dhruv; Yee, Connie (2017), Ceci, Michelangelo; Hollmén, Jaakko; Todorovski, Ljupčo; Vens, Celine (eds.), "Scatteract: Automated Extraction of Data from Scatter Plots", Machine Learning and Knowledge Discovery in Databases, vol. 10534, Cham: Springer International Publishing, pp. 135–150, arXiv:1704.06687, doi:10.1007/978-3-319-71249-9_9, ISBN 978-3-319-71248-2, S2CID 9543956, retrieved 26 December 2023
- ^ Moreau, David; Gamble, Beau (2022). "Conducting a meta-analysis in the age of open science: Tools, tips, and practical recommendations". Psychological Methods. 27 (3): 426–432. doi:10.1037/met0000351. ISSN 1939-1463. PMID 32914999. S2CID 221619510.
- ^ a b McGuinness, Luke A.; Higgins, Julian P. T. (2021). "Risk-of-bias VISualization (robvis): An R package and Shiny web app for visualizing risk-of-bias assessments". Research Synthesis Methods. 12 (1): 55–61. doi:10.1002/jrsm.1411. hdl:1983/e59b578e-1534-43d9-a438-8bc27b363a9a. ISSN 1759-2879. PMID 32336025.
- ^ Sanderson, S.; Tatt, I. D; Higgins, J. P. (1 June 2007). "Tools for assessing quality and susceptibility to bias in observational studies in epidemiology: a systematic review and annotated bibliography". International Journal of Epidemiology. 36 (3): 666–676. doi:10.1093/ije/dym018. ISSN 0300-5771. PMID 17470488.
- ^ Haddaway, Neal R.; Macura, Biljana; Whaley, Paul; Pullin, Andrew S. (2018). "ROSES RepOrting standards for Systematic Evidence Syntheses: pro forma, flow-diagram and descriptive summary of the plan and conduct of environmental systematic reviews and systematic maps". Environmental Evidence. 7 (1). Bibcode:2018EnvEv...7....7H. doi:10.1186/s13750-018-0121-7. ISSN 2047-2382.
- ^ Paez, Arsenio (2017). "Gray literature: An important resource in systematic reviews". Journal of Evidence-Based Medicine. 10 (3): 233–240. doi:10.1111/jebm.12266. ISSN 1756-5383. PMID 28857505.
- ^ Conn, Vicki S.; Valentine, Jeffrey C.; Cooper, Harris M.; Rantz, Marilyn J. (2003). "Grey Literature in Meta-Analyses". Nursing Research. 52 (4): 256–261. doi:10.1097/00006199-200307000-00008. ISSN 0029-6562. PMID 12867783. S2CID 27109643.
- ^ Scherer, Roberta W.; Saldanha, Ian J. (2019). "How should systematic reviewers handle conference abstracts? A view from the trenches". Systematic Reviews. 8 (1): 264. doi:10.1186/s13643-019-1188-0. ISSN 2046-4053. PMC 6836535. PMID 31699124.
- ^ Hartling, Lisa; Featherstone, Robin; Nuspl, Megan; Shave, Kassi; Dryden, Donna M.; Vandermeer, Ben (2017). "Grey literature in systematic reviews: a cross-sectional study of the contribution of non-English reports, unpublished studies and dissertations to the results of meta-analyses in child-relevant reviews". BMC Medical Research Methodology. 17 (1): 64. doi:10.1186/s12874-017-0347-z. ISSN 1471-2288. PMC 5395863. PMID 28420349.
- ^ Haddaway, N.R.; Woodcock, P.; Macura, B.; Collins, A. (2015). "Making literature reviews more reliable through application of lessons from systematic reviews". Conservation Biology. 29 (6): 1596–1605. Bibcode:2015ConBi..29.1596H. doi:10.1111/cobi.12541. ISSN 0888-8892. JSTOR 24761072. PMID 26032263. S2CID 20624428.
- ^ Egger, M; Jüni, P; Bartlett, C; Holenstein, F; Sterne, J (2003). "How important are comprehensive literature searches and the assessment of trial quality in systematic reviews? Empirical study". Health Technology Assessment. 7 (1): 1–82. doi:10.3310/hta7010. ISSN 1366-5278. PMID 12583822.
- ^ Lefebvre, Carol; Glanville, Julie; Briscoe, Simon; Littlewood, Anne; Marshall, Chris; Metzendorf, Maria-Inti; Noel-Storr, Anna; Rader, Tamara; Shokraneh, Farhad (23 September 2019), Higgins, Julian P.T.; Thomas, James; Chandler, Jacqueline; Cumpston, Miranda (eds.), "Searching for and selecting studies", Cochrane Handbook for Systematic Reviews of Interventions (1 ed.), Wiley, pp. 67–107, doi:10.1002/9781119536604.ch4, ISBN 978-1-119-53662-8, S2CID 204603849, retrieved 26 December 2023
- ^ McAuley, Laura; Pham, Ba'; Tugwell, Peter; Moher, David (2000). "Does the inclusion of grey literature influence estimates of intervention effectiveness reported in meta-analyses?". The Lancet. 356 (9237): 1228–1231. doi:10.1016/S0140-6736(00)02786-0. PMID 11072941. S2CID 33777183.
- ^ Hopewell, Sally; Clarke, Mike (2005). "Abstracts presented at the American Society of Clinical Oncology conference: how completely are trials reported?". Clinical Trials. 2 (3): 265–268. doi:10.1191/1740774505cn091oa. ISSN 1740-7745. PMID 16279150. S2CID 3601317.
- ^ Bhandari, Mohit; Devereaux, P. J.; Guyatt, Gordon H.; Cook, Deborah J.; Swiontkowski, Marc F.; Sprague, Sheila; Schemitsch, Emil H. (2002). "An Observational Study of Orthopaedic Abstracts and Subsequent Full-Text Publications". The Journal of Bone and Joint Surgery-American Volume. 84 (4): 615–621. doi:10.2106/00004623-200204000-00017. ISSN 0021-9355. PMID 11940624. S2CID 8807106.
- ^ Tierney, Jayne F.; Fisher, David J.; Burdett, Sarah; Stewart, Lesley A.; Parmar, Mahesh K. B. (31 January 2020). Shapiro, Steven D. (ed.). "Comparison of aggregate and individual participant data approaches to meta-analysis of randomised trials: An observational study". PLOS Medicine. 17 (1): e1003019. doi:10.1371/journal.pmed.1003019. ISSN 1549-1676. PMC 6993967. PMID 32004320.
- ^ Chang, Bei-Hung; Hoaglin, David C. (2017). "Meta-Analysis of Odds Ratios: Current Good Practices". Medical Care. 55 (4): 328–335. doi:10.1097/MLR.0000000000000696. ISSN 0025-7079. PMC 5352535. PMID 28169977.
- ^ Bakbergenuly, Ilyas; Hoaglin, David C.; Kulinskaya, Elena (2019). "Pitfalls of using the risk ratio in meta-analysis". Research Synthesis Methods. 10 (3): 398–419. doi:10.1002/jrsm.1347. ISSN 1759-2879. PMC 6767076. PMID 30854785.
- ^ Debray TP, Moons KG, van Valkenhoef G, Efthimiou O, Hummel N, Groenwold RH, Reitsma JB (December 2015). "Get real in individual participant data (IPD) meta-analysis: a review of the methodology". Research Synthesis Methods. 6 (4): 293–309. doi:10.1002/jrsm.1160. PMC 5042043. PMID 26287812.
- ^ Debray TP, Moons KG, Abo-Zaid GM, Koffijberg H, Riley RD (2013). "Individual participant data meta-analysis for a binary outcome: one-stage or two-stage?". PLOS ONE. 8 (4): e60650. Bibcode:2013PLoSO...860650D. doi:10.1371/journal.pone.0060650. PMC 3621872. PMID 23585842.
- ^ Burke DL, Ensor J, Riley RD (February 2017). "Meta-analysis using individual participant data: one-stage and two-stage approaches, and why they may differ". Statistics in Medicine. 36 (5): 855–875. doi:10.1002/sim.7141. PMC 5297998. PMID 27747915.
- ^ Nikolakopoulou, Adriani; Mavridis, Dimitris; Salanti, Georgia (2014). "How to interpret meta-analysis models: fixed effect and random effects meta-analyses". Evidence Based Mental Health. 17 (2): 64. doi:10.1136/eb-2014-101794. ISSN 1362-0347. PMID 24778439.
- ^ Dekkers, Olaf M. (2018). "Meta-analysis: Key features, potentials and misunderstandings". Research and Practice in Thrombosis and Haemostasis. 2 (4): 658–663. doi:10.1002/rth2.12153. PMC 6178740. PMID 30349883.
- ^ Helfenstein U (March 2002). "Data and models determine treatment proposals--an illustration from meta-analysis". Postgraduate Medical Journal. 78 (917): 131–134. doi:10.1136/pmj.78.917.131. PMC 1742301. PMID 11884693.
- ^ Dettori, Joseph R.; Norvell, Daniel C.; Chapman, Jens R. (2022). "Fixed-Effect vs Random-Effects Models for Meta-Analysis: 3 Points to Consider". Global Spine Journal. 12 (7): 1624–1626. doi:10.1177/21925682221110527. ISSN 2192-5682. PMC 9393987. PMID 35723546.
- ^ Hedges, Larry V.; Vevea, Jack L. (1998). "Fixed- and random-effects models in meta-analysis". Psychological Methods. 3 (4): 486–504. doi:10.1037/1082-989X.3.4.486. ISSN 1939-1463. S2CID 119814256.
- ^ Rice, Kenneth; Higgins, Julian P. T.; Lumley, Thomas (2018). "A re-evaluation of fixed effect(s) meta-analysis". Journal of the Royal Statistical Society. Series A (Statistics in Society). 181 (1): 205–227. doi:10.1111/rssa.12275. ISSN 0964-1998. JSTOR 44682165.
- ^ a b c d Viechtbauer, Wolfgang (2010). "Conducting Meta-Analyses in R with the metafor Package". Journal of Statistical Software. 36 (3). doi:10.18637/jss.v036.i03. ISSN 1548-7660. S2CID 15798713.
- ^ Holzmeister, Felix; Johannesson, Magnus; Böhm, Robert; Dreber, Anna; Huber, Jürgen; Kirchler, Michael (6 August 2024). "Heterogeneity in effect size estimates". Proceedings of the National Academy of Sciences. 121 (32): e2403490121. doi:10.1073/pnas.2403490121. ISSN 0027-8424. PMC 11317577. PMID 39078672.
- ^ Senn S (March 2007). "Trying to be precise about vagueness". Statistics in Medicine. 26 (7): 1417–1430. doi:10.1002/sim.2639. PMID 16906552. S2CID 17764847.
- ^ a b Al Khalaf MM, Thalib L, Doi SA (February 2011). "Combining heterogenous studies using the random-effects model is a mistake and leads to inconclusive meta-analyses". Journal of Clinical Epidemiology. 64 (2): 119–123. doi:10.1016/j.jclinepi.2010.01.009. PMID 20409685.
- ^ Brockwell SE, Gordon IR (March 2001). "A comparison of statistical methods for meta-analysis". Statistics in Medicine. 20 (6): 825–840. doi:10.1002/sim.650. PMID 11252006. S2CID 16932514.
- ^ a b Noma H (December 2011). "Confidence intervals for a random-effects meta-analysis based on Bartlett-type corrections". Statistics in Medicine. 30 (28): 3304–3312. doi:10.1002/sim.4350. hdl:2433/152046. PMID 21964669. S2CID 6556986.
- ^ Brockwell SE, Gordon IR (November 2007). "A simple method for inference on an overall effect in meta-analysis". Statistics in Medicine. 26 (25): 4531–4543. doi:10.1002/sim.2883. PMID 17397112. S2CID 887098.
- ^ Sidik K, Jonkman JN (November 2002). "A simple confidence interval for meta-analysis". Statistics in Medicine. 21 (21): 3153–3159. doi:10.1002/sim.1262. PMID 12375296. S2CID 21384942.
- ^ Jackson D, Bowden J (January 2009). "A re-evaluation of the 'quantile approximation method' for random effects meta-analysis". Statistics in Medicine. 28 (2): 338–348. doi:10.1002/sim.3487. PMC 2991773. PMID 19016302.
- ^ Poole C, Greenland S (September 1999). "Random-effects meta-analyses are not always conservative". American Journal of Epidemiology. 150 (5): 469–475. doi:10.1093/oxfordjournals.aje.a010035. PMID 10472946.
- ^ Riley RD, Higgins JP, Deeks JJ (February 2011). "Interpretation of random effects meta-analyses". BMJ. 342: d549. doi:10.1136/bmj.d549. PMID 21310794. S2CID 32994689.
- ^ Kriston L (March 2013). "Dealing with clinical heterogeneity in meta-analysis. Assumptions, methods, interpretation". International Journal of Methods in Psychiatric Research. 22 (1): 1–15. doi:10.1002/mpr.1377. PMC 6878481. PMID 23494781.
- ^ Langan, Dean; Higgins, Julian P.T.; Jackson, Dan; Bowden, Jack; Veroniki, Areti Angeliki; Kontopantelis, Evangelos; Viechtbauer, Wolfgang; Simmonds, Mark (2019). "A comparison of heterogeneity variance estimators in simulated random-effects meta-analyses". Research Synthesis Methods. 10 (1): 83–98. doi:10.1002/jrsm.1316. hdl:1983/c911791c-c687-4f12-bc0b-ffdbe42ca874. ISSN 1759-2879. PMID 30067315. S2CID 51890354.
- ^ a b "MetaXL User Guide" (PDF). Retrieved 18 September 2018.
- ^ Kontopantelis E, Reeves D (1 August 2010). "Metaan: Random-effects meta-analysis". Stata Journal. 10 (3): 395–407. doi:10.1177/1536867X1001000307 – via ResearchGate.
- ^ Field, Andy P.; Gillett, Raphael (2010). "How to do a meta-analysis". British Journal of Mathematical and Statistical Psychology. 63 (3): 665–694. doi:10.1348/000711010X502733. PMID 20497626. S2CID 22688261.
- ^ Kontopantelis E, Springate DA, Reeves D (2013). Friede T (ed.). "A re-analysis of the Cochrane Library data: the dangers of unobserved heterogeneity in meta-analyses". PLOS ONE. 8 (7): e69930. Bibcode:2013PLoSO...869930K. doi:10.1371/journal.pone.0069930. PMC 3724681. PMID 23922860.
- ^ Kontopantelis E, Reeves D (27 September 2013). "A short guide and a forest plot command (ipdforest) for one-stage meta-analysis". Stata Journal. 13 (3): 574–587. doi:10.1177/1536867X1301300308 – via ResearchGate.
- ^ a b Doi SA, Thalib L (January 2008). "A quality-effects model for meta-analysis". Epidemiology. 19 (1): 94–100. doi:10.1097/EDE.0b013e31815c24e7. PMID 18090860. S2CID 29723291.
- ^ Doi SA, Barendregt JJ, Mozurkewich EL (March 2011). "Meta-analysis of heterogeneous clinical trials: an empirical example". Contemporary Clinical Trials. 32 (2): 288–298. doi:10.1016/j.cct.2010.12.006. PMID 21147265.
- ^ Doi SA, Barendregt JJ, Khan S, Thalib L, Williams GM (July 2015). "Simulation Comparison of the Quality Effects and Random Effects Methods of Meta-analysis". Epidemiology. 26 (4): e42–e44. doi:10.1097/EDE.0000000000000289. PMID 25872162.
- ^ Doi SA, Barendregt JJ, Khan S, Thalib L, Williams GM (November 2015). "Advances in the meta-analysis of heterogeneous clinical trials II: The quality effects model". Contemporary Clinical Trials. 45 (Pt A): 123–129. doi:10.1016/j.cct.2015.05.010. PMID 26003432.
- ^ a b "MetaXL software page". Epigear.com. 3 June 2017. Retrieved 18 September 2018.
- ^ Rouse, Benjamin; Chaimani, Anna; Li, Tianjing (2017). "Network meta-analysis: an introduction for clinicians". Internal and Emergency Medicine. 12 (1): 103–111. doi:10.1007/s11739-016-1583-7. ISSN 1828-0447. PMC 5247317. PMID 27913917.
- ^ Phillips, Mark R.; Steel, David H.; Wykoff, Charles C.; Busse, Jason W.; Bannuru, Raveendhara R.; Thabane, Lehana; Bhandari, Mohit; Chaudhary, Varun; for the Retina Evidence Trials InterNational Alliance (R.E.T.I.N.A.) Study Group; Sivaprasad, Sobha; Kaiser, Peter; Sarraf, David; Bakri, Sophie J.; Garg, Sunir J.; Singh, Rishi P. (2022). "A clinician's guide to network meta-analysis". Eye. 36 (8): 1523–1526. doi:10.1038/s41433-022-01943-5. ISSN 0950-222X. PMC 9307840. PMID 35145277.
- ^ Bucher HC, Guyatt GH, Griffith LE, Walter SD (June 1997). "The results of direct and indirect treatment comparisons in meta-analysis of randomized controlled trials". Journal of Clinical Epidemiology. 50 (6): 683–691. doi:10.1016/s0895-4356(97)00049-8. PMID 9250266.
- ^ a b c d van Valkenhoef G, Lu G, de Brock B, Hillege H, Ades AE, Welton NJ (December 2012). "Automating network meta-analysis". Research Synthesis Methods. 3 (4): 285–299. doi:10.1002/jrsm.1054. PMID 26053422. S2CID 33613631.
- ^ Brooks SP, Gelman A (1998). "General methods for monitoring convergence of iterative simulations" (PDF). Journal of Computational and Graphical Statistics. 7 (4): 434–455. doi:10.1080/10618600.1998.10474787. S2CID 7300890.
- ^ Heck DW, Gronau QF, Wagenmakers EJ, Patil I (17 March 2021). "metaBMA: Bayesian model averaging for random and fixed effects meta-analysis". CRAN. Retrieved 9 May 2022.
- ^ Bartoš F, Maier M, Wagenmakers EJ, Goosen J, Denwood M, Plummer M (20 April 2022). "RoBMA: An R Package for Robust Bayesian Meta-Analyses". Retrieved 9 May 2022.
- ^ Gronau QF, Heck DW, Berkhout SW, Haaf JM, Wagenmakers EJ (July 2021). "A Primer on Bayesian Model-Averaged Meta-Analysis". Advances in Methods and Practices in Psychological Science. 4 (3). doi:10.1177/25152459211031256. hdl:11245.1/ec2c07d1-5ff0-431b-b53a-10f9c5d9541d. ISSN 2515-2459. S2CID 237699937.
- ^ Bartoš F, Maier M, Quintana D, Wagenmakers EJ (16 October 2020). "Adjusting for Publication Bias in JASP & R - Selection Models, PET-PEESE, and Robust Bayesian Meta-Analysis". Advances in Methods and Practices in Psychological Science. doi:10.31234/osf.io/75bqn. hdl:11245.1/5540e87c-0883-45e6-87de-48d2bf4c1e1d. S2CID 236826939.
- ^ a b Senn S, Gavini F, Magrez D, Scheen A (April 2013). "Issues in performing a network meta-analysis". Statistical Methods in Medical Research. 22 (2): 169–189. doi:10.1177/0962280211432220. PMID 22218368. S2CID 10860031.
- ^ White IR (2011). "Multivariate random-effects meta-regression: updates to mvmeta". The Stata Journal. 11 (2): 255–270. doi:10.1177/1536867X1101100206.
- ^ Willis BH, Hyde CJ (May 2014). "Estimating a test's accuracy using tailored meta-analysis-How setting-specific data may aid study selection". Journal of Clinical Epidemiology. 67 (5): 538–546. doi:10.1016/j.jclinepi.2013.10.016. PMID 24447592.
- ^ Willis BH, Hyde CJ (August 2015). "What is the test's accuracy in my practice population? Tailored meta-analysis provides a plausible estimate". Journal of Clinical Epidemiology. 68 (8): 847–854. doi:10.1016/j.jclinepi.2014.10.002. PMID 25479685. S2CID 205844216.
- ^ Zhang H, Deng L, Schiffman M, Qin J, Yu K (2020). "Generalized integration model for improved statistical inference by leveraging external summary data". Biometrika. 107 (3): 689–703. doi:10.1093/biomet/asaa014.
- ^ Higgins JP, Altman DG, Gøtzsche PC, Jüni P, Moher D, Oxman AD, et al. (October 2011). "The Cochrane Collaboration's tool for assessing risk of bias in randomised trials". BMJ. 343: d5928. doi:10.1136/bmj.d5928. PMC 3196245. PMID 22008217.
- ^ Whiting PF, Rutjes AW, Westwood ME, Mallett S, Deeks JJ, Reitsma JB, et al. (October 2011). "QUADAS-2: a revised tool for the quality assessment of diagnostic accuracy studies". Annals of Internal Medicine. 155 (8): 529–536. doi:10.7326/0003-4819-155-8-201110180-00009. PMID 22007046.
- ^ Royston P, Parmar MK, Sylvester R (March 2004). "Construction and validation of a prognostic model across several studies, with an application in superficial bladder cancer". Statistics in Medicine. 23 (6): 907–926. doi:10.1002/sim.1691. PMID 15027080. S2CID 23397142.
- ^ Willis BH, Riley RD (September 2017). "Measuring the statistical validity of summary meta-analysis and meta-regression results for use in clinical practice". Statistics in Medicine. 36 (21): 3283–3301. doi:10.1002/sim.7372. PMC 5575530. PMID 28620945.
- ^ Riley RD, Ahmed I, Debray TP, Willis BH, Noordzij JP, Higgins JP, Deeks JJ (June 2015). "Summarising and validating test accuracy results across multiple studies for use in clinical practice". Statistics in Medicine. 34 (13): 2081–2103. doi:10.1002/sim.6471. PMC 4973708. PMID 25800943.
- ^ LeLorier J, Grégoire G, Benhaddad A, Lapierre J, Derderian F (August 1997). "Discrepancies between meta-analyses and subsequent large randomized, controlled trials". The New England Journal of Medicine. 337 (8): 536–542. doi:10.1056/NEJM199708213370806. PMID 9262498.
- ^ a b Slavin RE (1986). "Best-Evidence Synthesis: An Alternative to Meta-Analytic and Traditional Reviews". Educational Researcher. 15 (9): 5–9. doi:10.3102/0013189X015009005. S2CID 146457142.
- ^ Hunter JE, Schmidt FL, Jackson GB, et al. (American Psychological Association. Division of Industrial-Organizational Psychology) (1982). Meta-analysis: cumulating research findings across studies. Beverly Hills, California: Sage. ISBN 978-0-8039-1864-1.
- ^ Glass GV, McGaw B, Smith ML (1981). Meta-analysis in social research. Beverly Hills, California: Sage Publications. ISBN 978-0-8039-1633-3.
- ^ Wolf, Vinzent; Kühnel, Anne; Teckentrup, Vanessa; Koenig, Julian; Kroemer, Nils B. (2021). "Does transcutaneous auricular vagus nerve stimulation affect vagally mediated heart rate variability? A living and interactive Bayesian meta-analysis". Psychophysiology. 58 (11): e13933. doi:10.1111/psyp.13933. ISSN 0048-5772. PMID 34473846.
- ^ Allbritton, David; Gómez, Pablo; Angele, Bernhard; Vasilev, Martin; Perea, Manuel (22 July 2024). "Breathing Life Into Meta-Analytic Methods". Journal of Cognition. 7 (1): 61. doi:10.5334/joc.389. ISSN 2514-4820. PMC 11276543. PMID 39072210.
- ^ Wagner, John A (3 September 2022). "The influence of unpublished studies on results of recent meta-analyses: publication bias, the file drawer problem, and implications for the replication crisis". International Journal of Social Research Methodology. 25 (5): 639–644. doi:10.1080/13645579.2021.1922805. ISSN 1364-5579.
- ^ Polanin JR, Tanner-Smith EE, Hennessy EA (2016). "Estimating the Difference Between Published and Unpublished Effect Sizes: A Meta-Review". Review of Educational Research. 86 (1): 207–236. doi:10.3102/0034654315582067. ISSN 0034-6543. S2CID 145513046.
- ^ Nassir Ghaemi, S.; Shirzadi, Arshia A.; Filkowski, Megan (10 September 2008). "Publication Bias and the Pharmaceutical Industry: The Case of Lamotrigine in Bipolar Disorder". The Medscape Journal of Medicine. 10 (9): 211. ISSN 1934-1997. PMC 2580079. PMID 19008973.
- ^ Martin, José Luis R.; Pérez, Víctor; Sacristán, Montse; Álvarez, Enric (2005). "Is grey literature essential for a better control of publication bias in psychiatry? An example from three meta-analyses of schizophrenia". European Psychiatry. 20 (8): 550–553. doi:10.1016/j.eurpsy.2005.03.011. ISSN 0924-9338. PMID 15994063.
- ^ a b Rosenthal R (1979). "The "File Drawer Problem" and the Tolerance for Null Results". Psychological Bulletin. 86 (3): 638–641. doi:10.1037/0033-2909.86.3.638. S2CID 36070395.
- ^ a b Vevea JL, Woods CM (December 2005). "Publication bias in research synthesis: sensitivity analysis using a priori weight functions". Psychological Methods. 10 (4): 428–443. doi:10.1037/1082-989X.10.4.428. PMID 16392998.
- ^ Hunter JE, Schmidt FL (1990). Methods of Meta-Analysis: Correcting Error and Bias in Research Findings. Newbury Park, California; London; New Delhi: SAGE Publications.
- ^ Nakagawa, Shinichi; Lagisz, Malgorzata; Jennions, Michael D.; Koricheva, Julia; Noble, Daniel W. A.; Parker, Timothy H.; Sánchez-Tójar, Alfredo; Yang, Yefeng; O'Dea, Rose E. (2022). "Methods for testing publication bias in ecological and evolutionary meta-analyses". Methods in Ecology and Evolution. 13 (1): 4–21. Bibcode:2022MEcEv..13....4N. doi:10.1111/2041-210X.13724. hdl:1885/294436. ISSN 2041-210X. S2CID 241159497.
- ^ Light RJ, Pillemer DB (1984). Summing up: the science of reviewing research. Cambridge, Massachusetts: Harvard University Press. ISBN 978-0-674-85431-4.
- ^ Ioannidis JP, Trikalinos TA (April 2007). "The appropriateness of asymmetry tests for publication bias in meta-analyses: a large survey". CMAJ. 176 (8): 1091–1096. doi:10.1503/cmaj.060410. PMC 1839799. PMID 17420491.
- ^ Hedges LV, Vevea JL (1996). "Estimating Effect Size Under Publication Bias: Small Sample Properties and Robustness of a Random Effects Selection Model". Journal of Educational and Behavioral Statistics. 21 (4): 299–332. doi:10.3102/10769986021004299. ISSN 1076-9986. S2CID 123680599.
- ^ Ferguson CJ, Brannick MT (March 2012). "Publication bias in psychological science: prevalence, methods for identifying and controlling, and implications for the use of meta-analyses". Psychological Methods. 17 (1): 120–128. doi:10.1037/a0024445. PMID 21787082.
- ^ Simmons JP, Nelson LD, Simonsohn U (November 2011). "False-positive psychology: undisclosed flexibility in data collection and analysis allows presenting anything as significant". Psychological Science. 22 (11): 1359–1366. doi:10.1177/0956797611417632. PMID 22006061.
- ^ LeBel E, Peters K (2011). "Fearing the future of empirical psychology: Bem's (2011) evidence of psi as a case study of deficiencies in modal research practice" (PDF). Review of General Psychology. 15 (4): 371–379. doi:10.1037/a0025172. S2CID 51686730. Archived from the original (PDF) on 24 November 2012.
- ^ Schober, Patrick; Bossers, Sebastiaan M.; Schwarte, Lothar A. (2018). "Statistical Significance Versus Clinical Importance of Observed Effect Sizes: What Do P Values and Confidence Intervals Really Represent?". Anesthesia & Analgesia. 126 (3): 1068–1072. doi:10.1213/ANE.0000000000002798. ISSN 0003-2999. PMC 5811238. PMID 29337724.
- ^ Gates, Simon; Ealing, Elizabeth (2019). "Reporting and interpretation of results from clinical trials that did not claim a treatment difference: survey of four general medical journals". BMJ Open. 9 (9): e024785. doi:10.1136/bmjopen-2018-024785. ISSN 2044-6055. PMC 6738699. PMID 31501094.
- ^ Hodges JS, Clayton MK (February 2011). "Random effects old and new". pp. 1–23. CiteSeerX 10.1.1.225.2685.
{{cite web}}
: Missing or empty|url=
(help) - ^ a b Hodges JS (2014). "Random effects old and new.". Richly parameterized linear models: additive, time series, and spatial models using random effects. Boca Raton: CRC Press. pp. 285–302. ISBN 978-1-4398-6683-2.
- ^ "Research into trans medicine has been manipulated". The Economist. ISSN 0013-0613. Retrieved 28 September 2024.
- ^ Stegenga J (December 2011). "Is meta-analysis the platinum standard of evidence?". Studies in History and Philosophy of Biological and Biomedical Sciences. 42 (4): 497–507. doi:10.1016/j.shpsc.2011.07.003. PMID 22035723.
- ^ Roseman M, Milette K, Bero LA, Coyne JC, Lexchin J, Turner EH, Thombs BD (2011), "Reporting of Conflicts of Interest in Meta-analyses of Trials of Pharmacological Treatments", Journal of the American Medical Association, 305 (10): 1008–1017, doi:10.1001/jama.2011.257, hdl:11370/d4a95ee2-429f-45a4-a917-d794ee954797, PMID 21386079, S2CID 11270323
- ^ Spink, Paul (1999). "Challenging Environmental Tobacco Smoke in the Workplace". Environmental Law Review. 1 (4): 243–265. Bibcode:1999EnvLR...1..243S. doi:10.1177/146145299900100402. ISSN 1461-4529.
- ^ Will, George (30 July 1998). "Polluted by the anti-tobacco crusade". Tampa Bay Times. Retrieved 28 September 2024.
- ^ Nelson, Jon P.; Kennedy, Peter E. (2009). "The Use (and Abuse) of Meta-Analysis in Environmental and Natural Resource Economics: An Assessment". Environmental and Resource Economics. 42 (3): 345–377. Bibcode:2009EnREc..42..345N. doi:10.1007/s10640-008-9253-5. ISSN 0924-6460.
- ^ Munafò MR, Flint J (September 2004). "Meta-analysis of genetic association studies". Trends in Genetics. 20 (9): 439–444. doi:10.1016/j.tig.2004.06.014. PMID 15313553.
- ^ Stone DL, Rosopa PJ (1 March 2017). "The Advantages and Limitations of Using Meta-analysis in Human Resource Management Research". Human Resource Management Review. 27 (1): 1–7. doi:10.1016/j.hrmr.2016.09.001. ISSN 1053-4822.
- ^ Button KS, Ioannidis JP, Mokrysz C, Nosek BA, Flint J, Robinson ES, Munafò MR (May 2013). "Power failure: why small sample size undermines the reliability of neuroscience". Nature Reviews. Neuroscience. 14 (5): 365–376. doi:10.1038/nrn3475. PMID 23571845. S2CID 455476.
- ^ a b Milkman KL, Gromet D, Ho H, Kay JS, Lee TW, Pandiloski P, et al. (December 2021). "Megastudies improve the impact of applied behavioural science". Nature. 600 (7889): 478–483. Bibcode:2021Natur.600..478M. doi:10.1038/s41586-021-04128-4. PMC 8822539. PMID 34880497. S2CID 245047340.
- ^ Cheung AC, Slavin RE (1 June 2016). "How Methodological Features Affect Effect Sizes in Education". Educational Researcher. 45 (5): 283–292. doi:10.3102/0013189X16656615. ISSN 0013-189X. S2CID 148531062.
- ^ Jüni P, Witschi A, Bloch R, Egger M (September 1999). "The hazards of scoring the quality of clinical trials for meta-analysis". JAMA. 282 (11): 1054–1060. doi:10.1001/jama.282.11.1054. PMID 10493204.
- ^ Armijo-Olivo S, Fuentes J, Ospina M, Saltaji H, Hartling L (September 2013). "Inconsistency in the items included in tools used in general health research and physical therapy to evaluate the methodological quality of randomized controlled trials: a descriptive analysis". BMC Medical Research Methodology. 13 (1): 116. doi:10.1186/1471-2288-13-116. PMC 3848693. PMID 24044807.
- ^ Debray TP, Riley RD, Rovers MM, Reitsma JB, Moons KG (October 2015). "Individual participant data (IPD) meta-analyses of diagnostic and prognostic modeling studies: guidance on their use". PLOS Medicine. 12 (10): e1001886. doi:10.1371/journal.pmed.1001886. PMC 4603958. PMID 26461078.
- ^ Debray TP, Moons KG, Ahmed I, Koffijberg H, Riley RD (August 2013). "A framework for developing, implementing, and evaluating clinical prediction models in an individual participant data meta-analysis" (PDF). Statistics in Medicine. 32 (18): 3158–3180. doi:10.1002/sim.5732. PMID 23307585. S2CID 25308961.
- ^ Debray TP, Koffijberg H, Vergouwe Y, Moons KG, Steyerberg EW (October 2012). "Aggregating published prediction models with individual participant data: a comparison of different approaches" (PDF). Statistics in Medicine. 31 (23): 2697–2712. doi:10.1002/sim.5412. PMID 22733546. S2CID 39439611.
- ^ Shadish, William R. (2014). "Analysis and meta-analysis of single-case designs: An introduction". Journal of School Psychology. 52 (2): 109–122. doi:10.1016/j.jsp.2013.11.009. PMID 24606971.
- ^ Zelinsky, Nicole A. M.; Shadish, William (19 May 2018). "A demonstration of how to do a meta-analysis that combines single-case designs with between-groups experiments: The effects of choice making on challenging behaviors performed by people with disabilities". Developmental Neurorehabilitation. 21 (4): 266–278. doi:10.3109/17518423.2015.1100690. ISSN 1751-8423. PMID 26809945. S2CID 20442353.
- ^ Van den Noortgate W, Onghena P (2007). "Aggregating Single-Case Results". The Behavior Analyst Today. 8 (2): 196–209. doi:10.1037/h0100613.
- ^ Mantel N, Haenszel W (April 1959). "Statistical aspects of the analysis of data from retrospective studies of disease". Journal of the National Cancer Institute. 22 (4): 719–748. doi:10.1093/jnci/22.4.719. PMID 13655060. S2CID 17698270.
- ^ Deeks JJ, Higgins JP, Altman DG, et al. (Cochrane Statistical Methods Group) (2021). "Chapter 10: Analysing data and undertaking meta-analyses: 10.4.2 Peto odds ratio method". In Higgins J, Thomas J, Chandler J, Cumpston M, Li T, Page M, Welch V (eds.). Cochrane Handbook for Systematic Reviews of Interventions (Version 6.2 ed.). The Cochrane Collaboration.
- ^ Bargaje R, Hariharan M, Scaria V, Pillai B (January 2010). "Consensus miRNA expression profiles derived from interplatform normalization of microarray data". RNA. 16 (1): 16–25. doi:10.1261/rna.1688110. PMC 2802026. PMID 19948767.
- ^ Li, Xihao; Quick, Corbin; Zhou, Hufeng; Gaynor, Sheila M.; Liu, Yaowu; Chen, Han; Selvaraj, Margaret Sunitha; Sun, Ryan; Dey, Rounak; Arnett, Donna K.; Bielak, Lawrence F.; Bis, Joshua C.; Blangero, John; Boerwinkle, Eric; Bowden, Donald W.; Brody, Jennifer A.; Cade, Brian E.; Correa, Adolfo; Cupples, L. Adrienne; Curran, Joanne E.; de Vries, Paul S.; Duggirala, Ravindranath; Freedman, Barry I.; Göring, Harald H. H.; Guo, Xiuqing; Haessler, Jeffrey; Kalyani, Rita R.; Kooperberg, Charles; Kral, Brian G.; Lange, Leslie A.; Manichaikul, Ani; Martin, Lisa W.; McGarvey, Stephen T.; Mitchell, Braxton D.; Montasser, May E.; Morrison, Alanna C.; Naseri, Take; O’Connell, Jeffrey R.; Palmer, Nicholette D.; Peyser, Patricia A.; Psaty, Bruce M.; Raffield, Laura M.; Redline, Susan; Reiner, Alexander P.; Reupena, Muagututi’a Sefuiva; Rice, Kenneth M.; Rich, Stephen S.; Sitlani, Colleen M.; Smith, Jennifer A.; Taylor, Kent D.; Vasan, Ramachandran S.; Willer, Cristen J.; Wilson, James G.; Yanek, Lisa R.; Zhao, Wei; NHLBI Trans-Omics for Precision Medicine (TOPMed) Consortium; TOPMed Lipids Working Group; Rotter, Jerome I.; Natarajan, Pradeep; Peloso, Gina M.; Li, Zilin; Lin, Xihong (January 2023). "Powerful, scalable and resource-efficient meta-analysis of rare variant associations in large whole genome sequencing studies". Nature Genetics. 55 (1): 154–164. doi:10.1038/s41588-022-01225-6. PMC 10084891. PMID 36564505. S2CID 255084231.
- ^ Stanek, Kevin C.; Ones, Deniz S. (2023). Of Anchors & Sails: Personality-ability trait constellations. Minneapolis, Minnesota, United States: University of Minnesota Libraries Publishing. pp. Chapters 4–7. doi:10.24926/9781946135988. ISBN 978-1-946135-98-8. S2CID 265335858.