Research transparency
Research transparency is a major aspect of scientific research. It covers a variety of scientific principles and practices: reproducibility, data and code sharing, citation standards or verifiability.
The definitions and norms of research transparency significantly differ depending on the disciplines and fields of research. Due to the lack of consistent terminology, research transparency has frequently been defined negatively by addressing non-transparent usages (which are part of questionable research practices).
After 2010, recurrent issues of research methodology have been increasingly acknowledged as structural crisis, that involve deep changes at all stages of the research process. Transparency has become a key value of the open science movement, which evolved from an initial focus on publishing to encompass a large diversity of research outputs. New common standards for research transparency, like the TOP Guidelines, aims to build and strengthen open research culture across disciplines and epistemic cultures.
Definitions
[edit]Confused terminologies
[edit]There is no widespread consensus on the definition of research transparency.
Differences between disciplines and epistemic cultures has largely contributed to different acceptions. The reproduction of past research has been a leading source of dissent. In an experimental setting, reproduction relies on the same set-up and apparatus, while replication only requires the use of the same methodology. Conversely, computational disciplines use reversed definitions of the term replicability and reproducibility.[1] Alternative taxonomies have proposed to make do entirely with the ambiguity of reproducibility/replicability/repeatability. Goodman, Fanelli and Ioannidis recommended instead a distinction between method reproducibility (same experimental/computational setup) and result reproducibility (different setup but same overall principles).[2]
Core institutional actors continue to disagree on the meaning and usage of key concepts. In 2019, the National Academies of Science of the United States retained the experimental definition of replication and reproduction, which remains "at odds with the more flexible way they are used by [other] major organizations".[3] The Association for Computing Machinery opted in 2016, for the computational definition and added also an intermediary notion of repeatability, where a different team of research use exactly the same measurement system and procedure.[4]
Debate over research transparency has also created new convergences between different disciplines and academic circles. In the Problem of science (2021), Rufus Barker Bausell argues that all disciplines, including the social sciences, currently face similar issues to medicine and physical sciences: "The problem, which has come to be known as the reproducibility crisis, affects almost all of science, not one or two individual disciplines."[5]
Negative definitions
[edit]Due to lack of consistent terminology over research transparency, scientists, policy-makers and other major stake-holders have increasingly rely on negative definitions: what are the practices and forms that harm or disrupt any common ideal of research transparency.[citation needed]
The taxonomy of scientific misconducts has been gradually expanded since the 1980s. The concept of questionable research practices (or QRP) was first incepted in a 1992 report of the Committee on Science, Engineering, and Public Policy as a way to address potentially non-intentional research failures (such as inadequacies in the research data management process).[6] Questionable research practices uncover a large grey area of problematic practices, which are frequently associated to deficiencies in research transparency. In 2016, a study identified as much as 34 questionable research practices or "degree of freedom", that can occur at all the steps of the project (the initial hypothesis, the design of the study, collection of the data, the analysis and the reporting).[7]
Surveys of disciplinary practices have shown large differences in the admissibility and spread of questionable research practices. While data fabrication and, to a lesser extent, rounding of statistical indicators like the p value are largely rejected, the non-publication of negative results or the adjonctions of supplementary data are not identified as major issues.[8][9]
In 2009, a meta-analysis of 18 surveys estimated that less than 2% of scientists "admitted to have fabricated, falsified or modified data or results at least once". Real prevalence may be under-estimated due to self-reporting: regarding "the behaviour of colleagues admission rates were 14.12%".[10] Questionable research practices are more widespread as more than one third of the respondents admit to have done it once.[10] A large 2021 survey of 6,813 respondents in the Netherlands found significantly higher estimate, with 4% of the respondents engaging in data fabrication and more than half of the respondents engaging in questionable research practices.[11] Higher rates can be either attributed to a deterioration of ethic norms or to "the increased awareness of research integrity in recent years".[12]
A new dimension of open science?
[edit]Transparency has been increasingly acknowledged as an important component of open science. Until the 2010s, definitions of open science have been mostly focused on technical access and enhanced participation and collaboration between academics and non-academics. In 2016, Liz Lyon identified transparency as a "third dimension" of open science, due to the fact that "the concept of transparency and the associated term ‘reproducibility’, have become increasingly important in the current interdisciplinary research environment."[13] According to Kevin Elliott, the open science movement "encompasses a number of different initiatives aimed at somewhat different forms of transparency."[14]
First drafted in 2014, the TOP guidelines have significantly contributed to bring transparency on the agenda of the open science movements.[15] They aim to promote an "open research culture" and implement "strong incentives to be more transparent".[16] They rely on eight standards, with different levels of compliance. While the standards are modular, they also aim to articulate a consistent ethos of science as "they also complement each other, in that commitment to one standard may facilitate adoption of others.".[15]
This open science framework of transparency has been in turn coopted by leading contributors and institutions on the topic of research transparency. After 2015, contributions from science historians underlined that there have been no significant deterioration of research quality, as past experiments and research design were not significantly better conceived and the rate of false or partially false has likely remained approximately constant for the last decades.[17][18] Consequently, proponents of research transparency have come to embrace more explicitly the discourse of open science: the culture of scientific transparency becomes a new ideal to achieve rather than a fundamental principle to re-establish.
The concept of transparency has contributed to create convergences between open science and other open movements in different areas such as open data or open government. In 2015, the OECD describe transparency as a common "rationale for open science and open data".[19]
History
[edit]Discourse and practices of research transparency (before 1945)
[edit]Transparency has been a fundamental criterion of experimental research for centuries.[20] Successful replications have become an integral part of the institutional discourse of natural sciences (then called natural philosophy) in the 17th century.[21] An early scientific society of Florence the Accademia del Cimento adopted in 1657 the motto provando e riprovando as a call for "repeated (public) performances of experimental trials"[22] A key member of the Accademia, the naturalist Francesco Redi described extensively of the forms and benefits of procedural experimentation, that made it possible to check for random effects, the soundness of the experiment design, or causal relationships through repeated trials[23] Replication and the open documentation of scientific experiments has become a key component of the diffusion of scientific knowledge in society: once they attained a satisfying rate of success, experiments could be performed in a variety of social spaces such as courts, marketplaces or learned salon.[24]
Although transparency has been early on acknowledged as a key component of science, it was not defined consistently. Most concept associated today with research transparency have arisen as terms of the art with no clear and widespread definitions. The concept of reproducibility appeared in an article on the "Methods of illuminations" first published in 1902: one of the methods examined was deemed limited regarding "reproducibility and constancy"[25] In 2019, the National Academies underlined that the distinction between reproduction, repetition and replication has remained largely unclear and unharmonized across disciplines: "What one group means by one word, the other group means by the other word. These terms — and others, such as repeatability — have long been used in relation to the general concept of one experiment or study confirming the results of another."[26]
Beyond this lack of formalization, there was a significant drift between the institutional and disciplinary discourse on research transparency and the reality of research work, that has persisted till the 21st century. Due to the high cost of the apparatus and the lack of incentives, most experiences were not reproduced by contemporary researchers: even a committed proponent of experimentalism like Robert Doyle had to devolve to a form of virtual experimentalism, by describing in detail a research design that has only been run once[27] For Friedrich Steinle, the gap between the postulated virtue of transparency and the material conditions of science has never been solved: "The rare cases in which replication actually is attempted are those that either are central for theory development (e.g., by being incompatible with existing theory) or promise broad attention due to major economical perspectives. Despite the formal ideal of replicability, we do not live in a culture of replication."[28]
Preconditions of the transparency crisis (1945–2000)
[edit]The development of big science after the Second World War has created unprecedented challenges for research transparency. The generalization of statistical methods across a large number of fields, as well as the increasing breadth and complexity of research projects, entailed a series of concerns about the lack of proper documentation of the scientific process.
Due to the expansion of the published research output, new quantitative methods for literature surveys have been developed under the label of meta-analysis or meta-science. These rely on the assumption that quantitative results and the details of the experimental and observational framework are sound (such as the size or the composition of the sample). In 1966, Stanley Schor and Irving Karten published one of the first generic evaluation of statistical methods in 67 leading medical journals. While few outright problematic papers were found, "in almost 73% of the reports read (those needing revision and those which should have been rejected), conclusions were drawn when the justification for these conclusions was invalid"[29]
In the 1970s and the 1980s, scientific misconducts gradually ceased to be presented as individual misconducts and became collective problems that need to be addressed by scientific institutions and communities. Between 1979 and 1981, several major cases of scientific frauds and plagiarism draw a larger focus to the issue from researchers and policy-makers in the United States[30] In a well-publicized investigation, Betrayers of Science, two scientific journalists described scientific fraud as a structural problem: "As more cases of frauds broke into public view (…) we wondered if fraud wasn't a quite regular minor feature of the scientific landscape (…) Logic, replication, peer review — all had been successfully defied by scientific forgers, often for extended periods of time".[31] The codification of research integrity has been the main institutional answer to this increased public scrutiny with "numerous codes of conduct field specific, national, and international alike."[32]
The reproducibility/transparency debate (2000–2015)
[edit]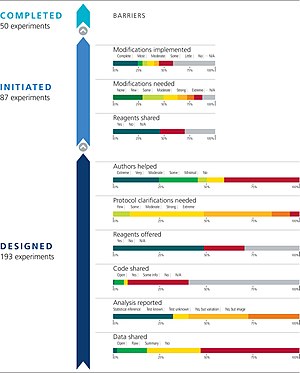
In the 2000s, long-standing issues on the standardization of research methodology have been increasingly presented as a structural crisis which "if not addressed the general public will inevitably lose its trust in science."[33] The early 2010s is commonly considered to be a turning point: "it wasn’t until sometime around 2011–2012 that the scientific community’s consciousness was bombarded with irreproducibility warnings".[34]
An early significant contribution to the debate has been the controversial and influential claim of John Ioannidis from 2005: "most published research findings are false.[35] The main argument was based on the excessively lax experimental standards in place, with numerous weak result being presented as solid research: "the majority of modern biomedical research is operating in areas with very low pre- and post-study probability for true findings"[36]
Due to being published in PLOS Medicine the study of Ioannidis had a considerable echo in psychology, medicine and biology. In the following decades, large range projects attempted to assess experimental reproducibility. In 2015, the Reproducibility Project: Psychology attempted to reproduced 100 studies from three top psychology journals (Journal of Personality and Social Psychology, Journal of Experimental Psychology: Learning, Memory, and Cognition, and Psychological Science): while nearly all paper had reproducible effects, it was found that only 36% of the replications were significant enough (p value above the common threshold of 0.05).[37] In 2021, another Reproducibility Project, Cancer Biology, analyzed 53 top papers about cancer published between 2010 and 2012 and established that the effect sizes were 85% smaller on average than the original findings .[38]
During the 2010s, the concept of reproducibility crisis has been expanded to a wider array of disciplines. The share of citations per year of the seminal paper of John Ioannidis, Why Most Published Research Findings Are False in the main fields of research according to the metadata recorded by the academic search engine Semantic Scholar (6,349 citations as of June 2022) shows how this framing has especially expanded to computing sciences. In Economics, a replication of 18 experimental studies in two major journals, found a failure rate comparable to psychology or medicine (39%).[39]
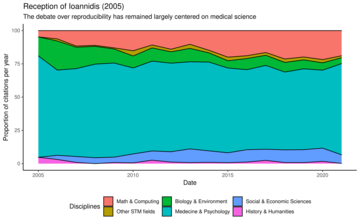
Several global surveys have reported a growing uneasiness of scientific communities over reproducibility and other issues of research transparency. In 2016, Nature highlighted that "more than 70% of researchers have tried and failed to reproduce another scientist's experiments, and more than half have failed to reproduce their own experiments"[40] The survey also found "no consensus on what reproducibility is or should be", in part due to disciplinary differences, which makes it harder to assess what could be the necessary steps to overcome the issue at plays.[40] The Nature survey has also been criticized for its paradoxical lack of research transparency, since it was not based on a representative sample but an online survey: it has "relied on convenience samples and other methodological choices that limit the conclusions that can be made about attitudes among the larger scientific community"[41] Despite mixed results, the Nature survey has been largely disseminated and ahs become a common entry data for any study of research transparency.[42]
Reproducibility crisis and other issues of research transparency have become a public topic addressed in the general press: "Reproducibility conversations are also unique compared to other methodological conversations because they have received sustained attention in both the scientific literature and the popular press".[43]
Research transparency and open science (2015–)
[edit]Since 2000, the open science movement has expanded beyond access to scientific outputs (publication, data or software) to encompass the entire process of scientific production. In 2018, Vicente-Saez and Martinez-Fuentes have attempted to map the common values shared by the standard definitions of open science in the English-speaking scientific literature indexed on Scopus and the Web of Science.[44] Access is no longer the main dimension of open science, as it has been extended by more recent commitments toward transparency, collaborative work and social impact.[45] Through this process, open science has been increasingly structured over a consisting set of ethical principles: "novel open science practices have developed in tandem with novel organising forms of conducting and sharing research through open repositories, open physical labs, and transdisciplinary research platforms. Together, these novel practices and organising forms are expanding the ethos of science at universities."[46]
The global scale of the open science movement and its integration in a large variety of technical tools, standards and regulations makes it possible to overcome the "classic collective action problem" embodied by research transparency: there is a structural discrepancy between the stated objective of scientific institutions and the lack of incentives to implement them at an individual level.[15]
The formalization of open science as a potential framework to ensure research transparency has been initially undertaken by institutional and communities initiatives. The TOP guidelines were elaborated in 2014 by a committee for Transparency and Openness Promotion that included "disciplinary leaders, journal editors, funding agency representatives, and disciplinary experts largely from the social and behavioral sciences".[15] The guidelines rely on eight standards, with different levels of compliance. While the standards are modular, they also aim to articulate a consistent ethos of science as "they also complement each other, in that commitment to one standard may facilitate adoption of others."[15]
After 2015, theses initiatives have partly influenced new regulations and code of ethics. The European Code of Conduct for Research Integrity from 2017 is strongly structured around open science and open data: it "pays data management almost an equal amount of attention as publishing and is also in this sense the most advanced of the four CoCs."[47] First adopted in July 2020, the Hong Kong principles for assessing researchers acknowledge open science as one of the five pillars of scientific integrity: "It seems clear that the various modalities of open science need to be rewarded in the assessment of researchers because these behaviors strongly increase transparency, which is a core principle of research integrity."[48]
Forms of research transparency
[edit]Research transparency has a large variety of forms depending on the disciplinary culture, the material condition of research and the interaction between scientists and other social circles (policy-makers, non-academic professionals, general audience). For Lyon, Jeng and Mattern, "the term ‘transparency’ has been applied in a range of contexts by diverse research stakeholders, who have articulated and framed the concept in a number of different ways."[19] In 2020, Kevin Elliott introduced a taxonomy of eight dimensions of research transparency: purpose, audience, content, timeframe, actors, mechanism, venues and dangers.[49] For Elliott not all forms of transparency are achievable and desirable, so that a proper terminology can help to make the more appropriate decisions: "While these are important objections, the taxonomy of transparency considered here suggests that the best response to them is typically not to abandon the goal of transparency entirely to consider what forms of transparency are best able to minimize them.".[50]
Method reproducibility
[edit]Goodman, Fanelli and Ioannidis define method reproducibility as "the provision of enough detail about study procedures and data so the same procedures could, in theory or in actuality, be exactly repeated."[2] This acception is largely synonymous with replicability in a computational context or reproducibility in an experimental context. In the report of the National Academies of Science, that opted for an experimental terminology, the counterpart of method reproducibility was described as "obtaining consistent results using the same input data; computational steps, methods, and code; and conditions of analysis".[26]
Method reproducibility is more attainable in computational sciences: as long as it behaves as expected, the same code should produce the same output. Open code, open data and more recently, research notebook are common recommendations to enhance method reproducibility. In principle, the wider availability of research output makes it possible to assess and audit the process of analysis. In practice, Roger Peng already underlined in 2011, that many projects require "computing power that may not be available to all researchers".[51] This issue has worsened in some areas such as Artificial Intelligence or Computer vision, as the development of very large deep learning models makes it nearly impossible to recreate them (or at a prohibitive cost), even when the original code and data are open. Method reproducibility can also be affected by library dependency, as the open code can rely on external programs which may not always be available or compatible. Two studies in 2018 and 2019 have shown that a large share of research notebook hosted on GitHub are no longer usable, either due to the of required extensions no longer being available or issues in the code.[52][53]
In experimental sciences, there is no commonly agreed criterium of method reproducibility: "in practice, the level of procedural detail needed to describe a study as "methodologically reproducible" does not have consensus."[2]
Result reproducibility
[edit]Goodman, Fanelli and Ioannidis define result reproducibility as "obtaining the same results from the conduct of an independent study whose procedures are as closely matched".[2] Result reproducibility is comparable to replication in an experimental context and reproducibility in a computational context. The definition of replicability retained in the National Academies of Science, largely applies to it: "obtaining consistent results across studies aimed at answering the same scientific question, each of which has obtained its own data.".[26] The reproducibility crisis met in experimental disciplines like psychology or medicine is mostly a crisis of "result reproducibility", since it concerns research that cannot been simply re-executed, but involve the independent recreation of the experimental design.[26] As such it is arguably the most debated form of research transparency in the recent years.[14]
Result reproducibility is harder to achieve than other forms of research transparency. It involve a variety of issues that may include computational reproducibility, accuracy of scientific measurement[26] and diversity of methodological approaches.[54] There are no universal standard to determine how close are the original procedures matched and criterium may vary depending on the disciplines or, even on the field of research. Consequently, meta-analysis of reproducibility have faced significant challenges. A 2015 study of 100 psychology papers conducted by Open Science Collaboration has been confronted with the "lack of a single accepted definition" which "opened the door to controversy about their methodological approach and conclusions" and made it necessary to fall back on "subjective assessments" of result reproducibility.[55]
Observation reproducibility and verifiability
[edit]In 2018 Sabina Leonelli defines observation reproducibility as the "expectation being that any skilled researcher placed in the same time and place would pick out, if not the same data, at least similar patterns".[56] This expectation recovers a large range scientific and scholarly practices in non-experimental disciplines:[57] "A tremendous amount of research in the medical, historical and social sciences does not rest on experimentation, but rather on observational techniques such as surveys, descriptions and case reports documenting unique circumstances"[56]
The development of open scientific infrastructure has radically transformed the status and the availability of scientific data and other primary sources. Access to theses resources has been thoroughly transformed by digitization and the attribution of unique identifiers. Permanent digital object identifiers (or DOI) have been first allocated to dataset since the early 2000s [58] which solved a long-standing debate on the citability of scientific data.[59]
Increased transparency of citations to primary sources or research materials has been framed by Andrew Moravcsik as a "revolution in qualitative research".[60] Access to theses resources has been thoroughly transformed by digitization and the attribution of unique identifiers. Permanent digital object identifiers (or DOI) have been first allocated to dataset since the early 2000s [58] which solved a long-standing debate on the citability of scientific data.[59]
Value transparency
[edit]Transparency of research values has been a major focus of disciplines with strong involvements in policy-making such as environment studies or social sciences. In 2009, Heather Douglas underlined that the public discourse on science has been largely dominated by normative ideals of objective research: if the procedures have been correctly applied, science results should be "value-free".[61] For Douglas, this ideal remains largely at loss with the effective process of research and scientific advising as pre-defined values may largely predate choices about the concepts, the protocols and the data used.[62] Douglas argued instead in favor of a disclosure of the values held by researchers: "the values should be made as explicit as possible in this indirect role, whether in policy documents or in the research papers of scientists."[63]
In the 2010s, several philosopher of sciences attempted to systematize value transparency in the context of open science. In 2017, Kevin Elliott emphasized three conditions for value transparency in research, the first one involved "being as transparent as possible about (…) data, methods, models and assumptions so that value influence can be scrutinized".[64]
Review and editorial transparency
[edit]Until the 2010s, the editorial practices of scholarly publishing have remained largely unformal and little studied: "Despite 350 years of scholarly publishing (…) research on ItAs [Instruction to authors], and on their evolution and change, is scarce."[65]
Editorial transparency has been recently acknowledged as a natural expansion of the debate over research reproducibility.[66][67] Several principles laid in the 2015 TOP guidelines already implied the existence of explicit editorial standards.[68] Unprecedented attention given to editorial transparency has also been motivated by the diversification and the complexification of the open science publishing landscape: "Triggered by a wide variety of expectations for journals’ editorial processes, journals have started to experiment with new ways of organizing their editorial assessment and peer review systems (...) The arrival of these innovations in an already diverse set of practices of peer review and editorial selection means we can no longer assume that authors, readers, and reviewers simply know how editorial assessment operates."[66]
Transparent by design: developing open workflow
[edit]The TOPs Guidelines have set up an influential transdisciplinary standard to establish result reproducibility in an open science context. While experimental and computational disciplines remains a primary focus, the standards have strived to integrate concerns and formats more specific to other disciplinary practices (such as research materials).
Informal incentives like badges or indexes have been initially advocated as a way to support the adoption of harmonized policies in regard to research transparency. Due to the development of open science, regulation and standardized infrastructures or processes are increasingly favored.
Sharing of research outputs
[edit]Data sharing has been early on identified as major potential solution to the reproducibility crisis and the lack of solid guidelines for statistical indicators. In 2005, John Ioannidis hypothesized that "some kind of registration or networking of data collections or investigators within fields may be more feasible than registration of each and every hypothesis-generating experiment."[69]
The sharing of research outputs is covered by three standards of the TOPs guidelines: on Data transparency (2), Analytic/code methods transparency (3) and Research materials transparency (4). All the relevant data, code and research materials are to be stored on a "trusted repository" and all analysis being already reproduced independently prior to publication.[70]
Extended citation standards
[edit]While citation standards are commonly applied to academic reference, there is much less formalization for all the other research output, such as data, code, primary sources or qualitative assessments.
In 2012, the American Political Science Association adopted new policies for open qualitative research.[71] They covered three dimensions of transparency: data transparency (in the sense of precise bibliographic data to the original sources), analytic transparency (in regards to claims extrapolated from the cited sources) and production transparency (in reference to the editorial choices made in the selection of the sources).[72] In 2014, Andrew Moravcsik advocated the implementation of transparency appendix, containing detailed quotes of original sources as well as annotations "explaining how the source supports the claim being made".[73]
According to the TOP Guidelines, "appropriate citation for data and materials" should be provided each publication.[70] Consequently, scientific outputs like code or dataset are fully acknowledged as citable contributions: "Regular and rigorous citation of these materials credit them as original intellectual contributions."[70]
Pre-registrations
[edit]Pre-registrations are covered by two TOP guidelines: Preregistration of studies (6) and Preregistration of analysis plans (7). In both cases, for the highest level of compliance journal should provide "link and badge in article to meeting requirements".[70]
Pre-registrations aims to preventively address a variety of questionable research practices. It takes usually the form of "a timestamped uneditable research plan to a public archive [that] states the hypotheses to be tested, target sample sizes".[74] Preregistration acts as an ethical contract as it theoretically constrains "the researcher degrees of freedom that make QRPs and p-hacking work".[74]
Preregistration do not solve all the range of questionable research practices. Selective reporting of the results would especially still be compatible with a predefined research plan: "preregistration does not fully counter publication bias as it does not guarantee that findings will be reported."[75] It has been argued that preregistration may also in some cases harm the quality of the research output by creating artificial constraints that do not fit with the reality of the research field: "Preregistration may interfere with valid inference because nothing prevents a researcher from preregistering a poor analytical plan."[76]
While advocated as a relatively cost-free solution, preregistration may be in reality harder to implement as it relies on a significant commitment on the part of the researchers. An empiric study of the adoption of open science experiments in a psychology journals has shown that "Adoption of pre-registration lags relative to other open science practices (…) from 2015 to 2020".[77] Consequently "even within researchers who see field-wide benefits of pre-registration, there is uncertainty surrounding the costs and benefits to individuals."[77]
Replication studies
[edit]Replication studies or assessments of replicability aims to re-do one or several original studies. Although the concept has only appeared in the 2010s, replication studies have been existing for decades but were not acknowledged as such.[78] The 2019 report of the National academies include a meta-analysis of 25 replications published between 1986 and 2019. It finds that the majority of the replication concern the medical and social sciences (especially, psychology and behavioral economics) and that there is for now no standardized evaluation criteria: "methods of assessing replicability are inconsistent and the replicability percentages depend strongly on the methods used."[78] Consequently, at least as for 2019, replication studies cannot be aggregated to extrapolate a replicability rate: they "are not necessarily indicative of the actual rate of non-replicability across science for a number"[78]
The TOPs guidelines have called for an enhanced recognition and valorization of replication studies. The eighth standards state that compliant journals should use "registered Reports as a submission option for replication studies with peer review".[70]
Open editorial policies
[edit]In July 2018, several publishers, librarians, journal editors and researchers drafted a Leiden Declaration for Transparent Editorial Policies.[79] The declaration underlined that journals "often do not contain information about reviewer selection, review criteria, blinding, the use of digital tools such as text similarity scanners, as well as policies on corrections and retractions" and this lack of transparency.[79] The declaration identifies four main publication and peer review phases that should be better documented:
- At submission: details on the governance of the journal, its scope, the editorial board or the rejection rates.[79]
- During review: criteria for selection, timing of the review and model of peer review (double bind, single bind, open).[79]
- Publication: disclosure of the "roles in the review process".[79]
- Post-publication: "criteria and procedures for corrections, expressions of concern, retraction" and other changes.[79]
In 2020, the Leiden Declaration has been expanded and supplemented by a Platform for Responsible Editorial Policies (PREP).[80] This initiative also aims to solve the structural scarcity of data and empirical information on editorial policies and peer review practices.[66][81] As of 2022, this database contains partially crowdsourced information on the editorial procedures of 490 journals,[82] from an initial base of 353 journals.[83] The procedures evaluated include especially "the level of anonymity afforded to authors and reviewers; the use of digital tools such as plagiarism scanners; and the timing of peer review in the research and publication process".[84] Despite this developments, research on editorial research still highlight the need for the "a comprehensive database that would allow authors or other stakeholders to compare journals based on their (…) requirements or recommendations"[85]
References
[edit]- ^ Plesser 2018, p. 1-2.
- ^ a b c d Goodman, Fanelli & Ioannidis 2016, p. 2.
- ^ Nelson et al. 2021, p. 45.
- ^ Plesser 2018, p. 2.
- ^ Bausell 2021, p. 1.
- ^ Pimple 2002, p. 202.
- ^ Wicherts et al. 2016.
- ^ John, Loewenstein & Prelec 2012.
- ^ Fraser et al. 2018.
- ^ a b Fanelli 2009.
- ^ Gopalakrishna et al. 2021.
- ^ Gopalakrishna et al. 2021, p. 5.
- ^ Lyon 2016, p. 160.
- ^ a b Elliott 2020, p. 2.
- ^ a b c d e Nosek et al. 2015, p. 1423.
- ^ Nosek et al. 2015, p. 1422-1423.
- ^ Steinle 2016.
- ^ Fanelli 2018.
- ^ a b Lyon, Jeng & Mattern 2017, p. 47.
- ^ Steinle 2016, p. 44.
- ^ Schickore 2011.
- ^ Schickore 2011, p. 330.
- ^ Schickore 2011, p. 332.
- ^ Steinle 2016, p. 45.
- ^ Bell 1902, p. 15.
- ^ a b c d e National Academies 2019, p. 46.
- ^ Shapin & Schaffer 2011, p. 60 sq..
- ^ Steinle 2016, p. 56.
- ^ Schor & Karten 1966, p. 148.
- ^ Löppönen & Vuorio 2013, p. 3.
- ^ Broad & Wade 1983, p. 8
- ^ Laine 2018, p. 49.
- ^ Drummond 2018, p. 2.
- ^ Bausell 2021, p. 10.
- ^ Ioannidis 2005.
- ^ Ioannidis 2005, p. 700.
- ^ Open Science Collaboration 2015.
- ^ "Reproducibility Project: Cancer Biology". www.cos.io. Center for Open Science. Retrieved 19 January 2022.
- ^ Camerer et al. 2016.
- ^ a b Baker 2016.
- ^ National Academies 2019, p. 83.
- ^ Bausell 2021, p. 128.
- ^ Nelson et al. 2021, p. 1-2.
- ^ Vicente-Saez & Martinez-Fuentes 2018.
- ^ Vicente-Saez & Martinez-Fuentes 2018, p. 2.
- ^ Vicente-Saez, Gustafsson & Van den Brande 2020, p. 1.
- ^ Laine 2018, p. 65.
- ^ Moher et al. 2020, p. 6
- ^ Elliott 2020, p. 6.
- ^ Elliott 2020, p. 8-9.
- ^ Peng 2011.
- ^ Rule, Tabard & Hollan 2018.
- ^ Pimentel et al. 2019.
- ^ National Academies 2019, p. 51.
- ^ Goodman, Fanelli & Ioannidis 2016, p. 4.
- ^ a b Leonelli 2018, p. 137.
- ^ Devezer et al. 2021.
- ^ a b Brase 2004.
- ^ a b Crosas 2014, p. 63.
- ^ Moravcsik 2014, p. 48.
- ^ Douglas 2009, p. 175.
- ^ Douglas 2009, p. 87 sq..
- ^ Douglas 2009, p. 176.
- ^ Elliott 2017, p. X.
- ^ Malički et al. 2021, p. 2.
- ^ a b c Horbach, Hepkema & Halffman 2020a, p. 2.
- ^ Justman 2019.
- ^ Nosek et al. 2015.
- ^ Ioannidis 2005, p. 701.
- ^ a b c d e Nosek et al. 2015, p. 1424.
- ^ Lupia & Elman 2014.
- ^ Moravcsik 2014, p. 48-49.
- ^ Moravcsik 2014, p. 50.
- ^ a b Romero 2019, p. 8.
- ^ Romero 2019, p. 9.
- ^ Devezer et al. 2021, p. 16.
- ^ a b Logg & Dorison 2021, p. 26.
- ^ a b c National Academies 2019, p. 77.
- ^ a b c d e f Radboud and Leiden transparency declaration 2019.
- ^ Horbach, Hepkema & Halffman 2020a, p. 1.
- ^ Squazzoni et al. 2020.
- ^ ResponsibleJournals.org
- ^ Horbach, Hepkema & Halffman 2020a, p. 4.
- ^ Horbach, Hepkema & Halffman 2020b.
- ^ Malički et al. 2021, p. 9.
Bibliography
[edit]Standards and declarations
[edit]- Nosek, B. A.; Alter, G.; Banks, G. C.; Borsboom, D.; Bowman, S. D.; Breckler, S. J.; Buck, S.; Chambers, C. D.; Chin, G.; Christensen, G.; Contestabile, M.; Dafoe, A.; Eich, E.; Freese, J.; Glennerster, R.; Goroff, D.; Green, D. P.; Hesse, B.; Humphreys, M.; Ishiyama, J.; Karlanflup, D.; Kraut, A.; Lupia, A.; Mabry, P.; Madon, T.; Malhotra, N.; Mayo-Wilson, E.; McNutt, M.; Miguel, E.; Paluck, E. Levy; Simonsohn, U.; Soderberg, C.; Spellman, B. A.; Turitto, J.; VandenBos, G.; Vazire, S.; Wagenmakers, E. J.; Wilson, R.; Yarkoni, T. (2015-06-26). "Promoting an open research culture [TOP Guidelines]". Science. 348 (6242): 1422–1425. Bibcode:2015Sci...348.1422N. doi:10.1126/science.aab2374. ISSN 0036-8075. PMC 4550299. PMID 26113702.
- Radboud and Leiden transparency declaration. Leiden University. 2019. Retrieved 2022-09-10.
Reports
[edit]- Reproducibility and Replicability in Science (Report). National Academies Press. 2019-09-20. ISBN 978-0-309-48619-4.
Books and theses
[edit]- Broad, William J.; Wade, Nicholas (1983). Betrayers of the Truth. Simon and Schuster. ISBN 978-0-671-44769-4.
- Douglas, Heather (2009-07-15). Science, Policy, and the Value-Free Ideal. University of Pittsburgh Pre. ISBN 978-0-8229-7357-7.
- Shapin, Steven; Schaffer, Simon (2011-08-15). Leviathan and the Air-Pump: Hobbes, Boyle, and the Experimental Life. Princeton University Press. ISBN 978-1-4008-3849-3.
- Elliott, Kevin C.; Steel, Daniel (2017-03-27). Current Controversies in Values and Science. Taylor & Francis. ISBN 978-1-317-27399-8.
- Elliott, Kevin Christopher (2017). A Tapestry of Values: An Introduction to Values in Science. Oxford University Press. ISBN 978-0-19-026081-1.
- Pimple, Kenneth D. (2017-05-15). Research Ethics. Routledge. ISBN 978-1-351-90400-1.
- Bausell, R. Barker (2021-01-26). The Problem with Science: The Reproducibility Crisis and What to do About It. Oxford University Press. ISBN 978-0-19-753654-4.
- Grahe, Jon (2021-08-30). A Journey into Open Science and Research Transparency in Psychology. Routledge. ISBN 978-1-00-043049-3.
Academic articles
[edit]- Bell, Louis (January 1902). "Methods of Illumination". Transactions of the American Institute of Electrical Engineers. XIX: 1–27. doi:10.1109/T-AIEE.1902.4763952. ISSN 2330-9431. S2CID 51639145.
- Schor, Stanley; Karten, Irving (1966-03-28). "Statistical Evaluation of Medical Journal Manuscripts". JAMA. 195 (13): 1123–1128. doi:10.1001/jama.1966.03100130097026. ISSN 0098-7484. PMID 5952081. Retrieved 2020-02-11.
- Pimple, Kenneth D. (2002-06-01). "Six domains of research ethics". Science and Engineering Ethics. 8 (2): 191–205. doi:10.1007/s11948-002-0018-1. ISSN 1471-5546. PMID 12092490. S2CID 25084326. Retrieved 2022-02-19.
- Brase, Jan (2004). "Using Digital Library Techniques – Registration of Scientific Primary Data". In Rachel Heery, Liz Lyon (ed.). Research and Advanced Technology for Digital Libraries. Lecture Notes in Computer Science. Berlin, Heidelberg: Springer. pp. 488–494. doi:10.1007/978-3-540-30230-8_44. ISBN 978-3-540-30230-8.
- Ioannidis, John P. A. (2005). "Why Most Published Research Findings Are False". PLOS Medicine. 2 (8): –124. doi:10.1371/journal.pmed.0020124. ISSN 1549-1676. PMC 1182327. PMID 16060722.
- Hubbard, Raymond; Lindsay, R. Murray (2008-02-01). "Why P Values Are Not a Useful Measure of Evidence in Statistical Significance Testing". Theory & Psychology. 18: 69–88. doi:10.1177/0959354307086923. S2CID 143487211. Retrieved 2020-02-11.
- Fanelli, Daniele (2009). "How Many Scientists Fabricate and Falsify Research? A Systematic Review and Meta-Analysis of Survey Data". PLOS ONE. 4 (5): –5738. Bibcode:2009PLoSO...4.5738F. doi:10.1371/journal.pone.0005738. ISSN 1932-6203. PMC 2685008. PMID 19478950.
- Schickore, Jutta (2011). "The Significance of Re-Doing Experiments: A Contribution to Historically Informed Methodology". Erkenntnis (1975-). 75 (3): 325–347. doi:10.1007/s10670-011-9332-9. ISSN 0165-0106. JSTOR 41476727. S2CID 146243575. Retrieved 2022-06-12.
- Peng, Roger D. (2011-12-02). "Reproducible research in computational science". Science. 334 (6060): 1226–1227. Bibcode:2011Sci...334.1226P. doi:10.1126/science.1213847. ISSN 1095-9203. PMC 3383002. PMID 22144613.
- John, Leslie K.; Loewenstein, George; Prelec, Drazen (2012-04-16). "Measuring the Prevalence of Questionable Research Practices With Incentives for Truth Telling". Psychological Science. 23 (5): 524–532. doi:10.1177/0956797611430953. PMID 22508865. S2CID 8400625. Retrieved 2020-02-12.
- Löppönen, Paavo; Vuorio, Eero (2013-02-21). "Tutkimusetiikka Suomessa 1980-luvulta tähän päivään". Tieteessä tapahtuu. 31 (1). ISSN 1239-6540. Retrieved 2022-02-12.
- Moravcsik, Andrew (2014). "Transparency: The Revolution in Qualitative Research". PS: Political Science & Politics. 47 (1): 48–53. doi:10.1017/S1049096513001789 (inactive 1 November 2024). ISSN 1049-0965. S2CID 14202765. Retrieved 2022-06-13.
{{cite journal}}
: CS1 maint: DOI inactive as of November 2024 (link) - Lupia, Arthur; Elman, Colin (2014). "Openness in Political Science: Data Access and Research Transparency: Introduction". PS: Political Science & Politics. 47 (1): 19–42. doi:10.1017/S1049096513001716 (inactive 1 November 2024). ISSN 1049-0965. S2CID 154301281. Retrieved 2022-06-13.
{{cite journal}}
: CS1 maint: DOI inactive as of November 2024 (link) - Crosas, Mercè (2014-05-26). "The Evolution of Data Citation: From Principles to Implementation". IASSIST Quarterly. 37 (1–4): 62. doi:10.29173/iq504. ISSN 0739-1137. Retrieved 2022-05-15.
- Head, Megan L.; Holman, Luke; Lanfear, Rob; Kahn, Andrew T.; Jennions, Michael D. (2015-03-13). "The Extent and Consequences of P-Hacking in Science". PLOS Biology. 13 (3): –1002106. doi:10.1371/journal.pbio.1002106. ISSN 1545-7885. PMC 4359000. PMID 25768323.
- Elliott, Kevin C.; Resnik, David B. (2015-01-01). "Scientific Reproducibility, Human Error, and Public Policy". BioScience. 65 (1): 5–6. doi:10.1093/biosci/biu197. ISSN 0006-3568. PMC 4776714. PMID 26955072. Retrieved 2022-06-12.
- Open Science Collaboration (August 2015). "PSYCHOLOGY. Estimating the reproducibility of psychological science". Science. 349 (6251): aac4716. doi:10.1126/science.aac4716. hdl:10722/230596. PMID 26315443. S2CID 218065162.
- Ioannidis, John P. A.; Fanelli, Daniele; Dunne, Debbie Drake; Goodman, Steven N. (2015-10-02). "Meta-research: Evaluation and Improvement of Research Methods and Practices". PLOS Biology. 13 (10): e1002264. doi:10.1371/journal.pbio.1002264. ISSN 1544-9173. PMC 4592065. PMID 26431313.
- Wicherts, Jelte M.; Veldkamp, Coosje L. S.; Augusteijn, Hilde E. M.; Bakker, Marjan; van Aert, Robbie C. M.; van Assen, Marcel A. L. M. (2016). "Degrees of Freedom in Planning, Running, Analyzing, and Reporting Psychological Studies: A Checklist to Avoid p-Hacking". Frontiers in Psychology. 7: 1832. doi:10.3389/fpsyg.2016.01832. ISSN 1664-1078. PMC 5122713. PMID 27933012.
- Camerer CF, Dreber A, Forsell E, Ho TH, Huber J, Johannesson M, et al. (March 2016). "Evaluating replicability of laboratory experiments in economics". Science. 351 (6280): 1433–1436. Bibcode:2016Sci...351.1433C. doi:10.1126/science.aaf0918. PMID 26940865.
- Wicherts, Jelte M.; Veldkamp, Coosje L. S.; Augusteijn, Hilde E. M.; Bakker, Marjan; van Aert, Robbie C. M.; van Assen, Marcel A. L. M. (2016). "Degrees of Freedom in Planning, Running, Analyzing, and Reporting Psychological Studies: A Checklist to Avoid p-Hacking". Frontiers in Psychology. 7: 1832. doi:10.3389/fpsyg.2016.01832. ISSN 1664-1078. PMC 5122713. PMID 27933012.
- Lyon, Liz (2016-06-23). "Transparency: the emerging third dimension of Open Science and Open Data". LIBER Quarterly: The Journal of the Association of European Research Libraries. 25 (4): 153–171. doi:10.18352/lq.10113. ISSN 2213-056X. S2CID 155715556. Retrieved 2022-02-18.
- Goodman, Steven N.; Fanelli, Daniele; Ioannidis, John P. A. (2016-06-01). "What does research reproducibility mean?". Science Translational Medicine. 8 (341): 341–12. doi:10.1126/scitranslmed.aaf5027. ISSN 1946-6242. PMID 27252173. S2CID 848096.
- Aguinis, Herman; Cascio, Wayne F.; Ramani, Ravi S. (2017-08-01). "Science's reproducibility and replicability crisis: International business is not immune". Journal of International Business Studies. 48 (6): 653–663. doi:10.1057/s41267-017-0081-0. ISSN 1478-6990. S2CID 49226080. Retrieved 2020-02-10.
- Lyon, Liz; Jeng, Wei; Mattern, Eleanor (2017-09-16). "Research Transparency: A Preliminary Study of Disciplinary Conceptualisation, Drivers, Tools and Support Services". International Journal of Digital Curation. 12 (1): 46–64. doi:10.2218/ijdc.v12i1.530 (inactive 2024-11-21). ISSN 1746-8256. Retrieved 2022-06-10.
{{cite journal}}
: CS1 maint: DOI inactive as of November 2024 (link) - Munafò, Marcus; Nosek, Brian; Bishop, Dorothy; Button, Katherine; Chambers, Christopher; Percie du Sert, Nathalie; Simonsohn, Uri; Wagenmakers, Eric-Jan; Ware, Jennifer; Ioannidis, John (2017-01-10). "A manifesto for reproducible science". Nature Human Behaviour. 1 (1): 0021. doi:10.1038/s41562-016-0021. PMC 7610724. PMID 33954258.
- Drummond, Chris (2018-01-02). "Reproducible research: a minority opinion". Journal of Experimental & Theoretical Artificial Intelligence. 30 (1): 1–11. Bibcode:2018JETAI..30....1D. doi:10.1080/0952813X.2017.1413140. ISSN 0952-813X. S2CID 46838834. Retrieved 2020-02-12.
- Vicente-Saez, Ruben; Martinez-Fuentes, Clara (2018-07-01). "Open Science now: A systematic literature review for an integrated definition". Journal of Business Research. 88: 428–436. doi:10.1016/j.jbusres.2017.12.043. ISSN 0148-2963. S2CID 158229869. Retrieved 2021-11-11.
- Redish, A. David; Kummerfeld, Erich; Morris, Rebecca Lea; Love, Alan C. (2018-05-15). "Reproducibility failures are essential to scientific inquiry". Proceedings of the National Academy of Sciences. 115 (20): 5042–5046. Bibcode:2018PNAS..115.5042R. doi:10.1073/pnas.1806370115. PMC 5960342. PMID 29765001.
- Fanelli, Daniele (2018-03-13). "Opinion: Is science really facing a reproducibility crisis, and do we need it to?". Proceedings of the National Academy of Sciences. 115 (11): 2628–2631. doi:10.1073/pnas.1708272114. ISSN 0027-8424. PMC 5856498. PMID 29531051.
- Plesser, Hans E. (2018-01-18). "Reproducibility vs. Replicability: A Brief History of a Confused Terminology". Frontiers in Neuroinformatics. 11: 76. doi:10.3389/fninf.2017.00076. ISSN 1662-5196. PMC 5778115. PMID 29403370.
- Fraser, Hannah; Parker, Tim; Nakagawa, Shinichi; Barnett, Ashley; Fidler, Fiona (2018). "Questionable research practices in ecology and evolution". PLOS ONE. 13 (7): –0200303. Bibcode:2018PLoSO..1300303F. doi:10.1371/journal.pone.0200303. ISSN 1932-6203. PMC 6047784. PMID 30011289.
- Laine, Heidi (2018-12-31). "Open science and codes of conduct on research integrity". Informaatiotutkimus. 37 (4). doi:10.23978/inf.77414. ISSN 1797-9129. S2CID 115161422. Retrieved 2021-11-11.
- Peels, Rik; Bouter, Lex (2018-08-07). "The possibility and desirability of replication in the humanities". Palgrave Communications. 4 (1): 1–4. doi:10.1057/s41599-018-0149-x. hdl:1871.1/1286b1ed-f663-4f24-886e-f91520856464. ISSN 2055-1045. S2CID 51935690. Retrieved 2022-09-10.
- Fraser, Hannah; Parker, Tim; Nakagawa, Shinichi; Barnett, Ashley; Fidler, Fiona (2018). "Questionable research practices in ecology and evolution". PLOS ONE. 13 (7): –0200303. Bibcode:2018PLoSO..1300303F. doi:10.1371/journal.pone.0200303. ISSN 1932-6203. PMC 6047784. PMID 30011289.
- MacCoun, Robert (2019-08-06). "p-Hacking: A Strategic Analysis". Rochester, NY: Social Science Research Network. SSRN 3433221. Retrieved 2020-02-10.
{{cite journal}}
: Cite journal requires|journal=
(help) - Romero, Felipe (2019). "Philosophy of science and the replicability crisis". Philosophy Compass. 14 (11): –12633. doi:10.1111/phc3.12633. ISSN 1747-9991. S2CID 202261836. Retrieved 2022-09-10.
- Justman, Quincey (2019). "A Necessary Complement to Transparent Peer Review: Editorial Transparency". Cell Systems. 9 (1): 1–2. doi:10.1016/j.cels.2019.07.002. ISSN 2405-4712. PMID 31344358. S2CID 198912667.
- Murphy, P. Karen; Dowd, Alicia C.; Lloyd, Gwendolyn M.; List, Alexandra (2020-02-01). "Transparency in Literature Syntheses and Editorial Review: Introducing the Methodological Guidance Paper Series". Review of Educational Research. 90 (1): 3–5. doi:10.3102/0034654319901128. ISSN 0034-6543. S2CID 214414648. Retrieved 2022-09-10.
- Horbach, Serge P.J.M.; Hepkema, Wytske M.; Halffman, Willem (2020). "The Platform for Responsible Editorial Policies: An initiative to foster editorial transparency in scholarly publishing". Learned Publishing. 33 (3): 340–344. doi:10.1002/leap.1312. hdl:2066/222238. ISSN 0953-1513. S2CID 219740617. Retrieved 2022-09-10.
{{cite journal}}
: CS1 maint: date and year (link) - Vicente-Saez, Ruben; Gustafsson, Robin; Van den Brande, Lieve (2020-07-01). "The dawn of an open exploration era: Emergent principles and practices of open science and innovation of university research teams in a digital world". Technological Forecasting and Social Change. 156: 120037. doi:10.1016/j.techfore.2020.120037. ISSN 0040-1625. S2CID 216442567.
- Horbach, Serge; Hepkema, Wytske; Halffman, Willem (2020-06-02). "Hundreds of journals' editorial practices captured in database". Nature. 582 (7810): 32. Bibcode:2020Natur.582...32H. doi:10.1038/d41586-020-01628-7. hdl:2066/221028. PMID 32488161. S2CID 219175762. Retrieved 2022-09-10.
- Moher, David; Bouter, Lex; Kleinert, Sabine; Glasziou, Paul; Sham, Mai Har; Barbour, Virginia; Coriat, Anne-Marie; Foeger, Nicole; Dirnagl, Ulrich (2020). "The Hong Kong Principles for assessing researchers: Fostering research integrity". PLOS Biology. 18 (7): –3000737. doi:10.1371/journal.pbio.3000737. ISSN 1545-7885. PMC 7365391. PMID 32673304. S2CID 220609403.
- Elliott, Kevin C. (2020-06-16). "A Taxonomy of Transparency in Science". Canadian Journal of Philosophy. 52 (3): 342–355. doi:10.1017/can.2020.21. ISSN 0045-5091. S2CID 225695820. Retrieved 2022-06-12.
- Squazzoni, Flaminio; Ahrweiler, Petra; Barros, Tiago; Bianchi, Federico; Birukou, Aliaksandr; Blom, Harry J. J.; Bravo, Giangiacomo; Cowley, Stephen; Dignum, Virginia; Dondio, Pierpaolo; Grimaldo, Francisco; Haire, Lynsey; Hoyt, Jason; Hurst, Phil; Lammey, Rachael; MacCallum, Catriona; Marušić, Ana; Mehmani, Bahar; Murray, Hollydawn; Nicholas, Duncan; Pedrazzi, Giorgio; Puebla, Iratxe; Rodgers, Peter; Ross-Hellauer, Tony; Seeber, Marco; Shankar, Kalpana; Van Rossum, Joris; Willis, Michael (2020). "Unlock ways to share data on peer review". Nature. 578 (7796): 512–514. Bibcode:2020Natur.578..512S. doi:10.1038/d41586-020-00500-y. hdl:2434/717112. PMID 32099126. S2CID 211265856. Retrieved 2022-09-10.
- Malički, Mario; Jerončić, Ana; Aalbersberg, IJsbrand Jan; Bouter, Lex; ter Riet, Gerben (2021-10-05). "Systematic review and meta-analyses of studies analysing instructions to authors from 1987 to 2017". Nature Communications. 12 (1): 5840. Bibcode:2021NatCo..12.5840M. doi:10.1038/s41467-021-26027-y. ISSN 2041-1723. PMC 8492806. PMID 34611157.
- Brock, Theo C. M.; Elliott, Kevin C.; Gladbach, Anja; Moermond, Caroline; Romeis, Jörg; Seiler, Thomas-Benjamin; Solomon, Keith; Peter Dohmen, G. (November 2021). "Open Science in regulatory environmental risk assessment". Integrated Environmental Assessment and Management. 17 (6): 1229–1242. Bibcode:2021IEAM...17.1229B. doi:10.1002/ieam.4433. ISSN 1551-3777. PMC 8596791. PMID 33913617.
- Logg, Jennifer M.; Dorison, Charles A. (2021). "Pre-registration: Weighing costs and benefits for researchers". Organizational Behavior and Human Decision Processes. 167: 18–27. doi:10.1016/j.obhdp.2021.05.006. ISSN 0749-5978. Retrieved 2022-09-10.
- Nelson, Nicole C.; Ichikawa, Kelsey; Chung, Julie; Malik, Momin M. (2021). "Mapping the discursive dimensions of the reproducibility crisis: A mixed methods analysis". PLOS ONE. 16 (7): –0254090. Bibcode:2021PLoSO..1654090N. doi:10.1371/journal.pone.0254090. ISSN 1932-6203. PMC 8270481. PMID 34242331.
- Gopalakrishna, Gowri; Riet, Gerben ter; Vink, Gerko; Stoop, Ineke; Wicherts, Jelte; Bouter, Lex (2021-07-06). Prevalence of questionable research practices, research misconduct and their potential explanatory factors: a survey among academic researchers in The Netherlands. MetaArXiv. Retrieved 2022-02-18.
- Devezer, Berna; Navarro, Danielle J.; Vandekerckhove, Joachim; Ozge Buzbas, Erkan (2021-03-31). "The case for formal methodology in scientific reform". Royal Society Open Science. 8 (3): 200805. Bibcode:2021RSOS....800805D. doi:10.1098/rsos.200805. ISSN 2054-5703. PMC 8101540. PMID 34035933.
- Hudson, Robert (2021-09-01). "Should We Strive to Make Science Bias-Free? A Philosophical Assessment of the Reproducibility Crisis". Journal for General Philosophy of Science. 52 (3): 389–405. doi:10.1007/s10838-020-09548-w. ISSN 1572-8587. PMC 8550477. PMID 34720421. Retrieved 2022-06-12.
- Nelson, Nicole C.; Ichikawa, Kelsey; Chung, Julie; Malik, Momin M. (2021). "Mapping the discursive dimensions of the reproducibility crisis: A mixed methods analysis". PLOS ONE. 16 (7): –0254090. Bibcode:2021PLoSO..1654090N. doi:10.1371/journal.pone.0254090. ISSN 1932-6203. PMC 8270481. PMID 34242331.
- Elliott, Kevin (2022). "Open Science for Non-Specialists: Making Open Science Meaningful Outside the Scientific Community". Philosophy of Science. 89 (5): 21. doi:10.1017/psa.2022.36.
- Haig, Brian D. (2022-06-01). "Understanding Replication in a Way That Is True to Science". Review of General Psychology. 26 (2): 224–240. doi:10.1177/10892680211046514. ISSN 1089-2680. S2CID 236815515. Retrieved 2022-06-12.
- Douglas, Heather; Elliott, Kevin C. (2022-05-11). "Addressing the Reproducibility Crisis: A Response to Hudson". Journal for General Philosophy of Science. 53 (2): 201–209. doi:10.1007/s10838-022-09606-5. ISSN 1572-8587. S2CID 248733109. Retrieved 2022-06-12.
Chapters
[edit]- Steinle, Friedrich (2016). "Stability and Replication of Experimental Results: A Historical Perspective". Reproducibility. John Wiley & Sons, Ltd. pp. 39–63. doi:10.1002/9781118865064.ch3. ISBN 978-1-118-86506-4. Retrieved 2020-02-10.
- Leonelli, Sabina (2018-10-24). "Rethinking Reproducibility as a Criterion for Research Quality". In Luca Fiorito; Scott Scheall; Carlos Eduardo Suprinyak (eds.). Research in the History of Economic Thought and Methodology. Vol. 36. Emerald Publishing Limited. pp. 129–146. doi:10.1108/S0743-41542018000036B009. hdl:10871/31336. ISBN 978-1-78756-424-4. S2CID 55353995. Retrieved 2022-09-10.
- Fidler, Fiona; Wilcox, John (2018). "Reproducibility of Scientific Results". In Zalta, Edward N. (ed.). The Stanford Encyclopedia of Philosophy (Winter 2018 ed.). Metaphysics Research Lab, Stanford University. Retrieved 2020-02-10.
Conferences
[edit]- Gómez, Omar S.; Juristo, Natalia; Vegas, Sira (2010-09-16). "Replications types in experimental disciplines". Proceedings of the 2010 ACM-IEEE International Symposium on Empirical Software Engineering and Measurement. ESEM '10. Bolzano-Bozen, Italy: Association for Computing Machinery. pp. 1–10. doi:10.1145/1852786.1852790. ISBN 978-1-4503-0039-1.
- Rule, Adam; Tabard, Aurélien; Hollan, James D. (2018-04-19). "Exploration and Explanation in Computational Notebooks". Proceedings of the 2018 CHI Conference on Human Factors in Computing Systems. CHI '18. Montreal QC, Canada: Association for Computing Machinery. pp. 1–12. doi:10.1145/3173574.3173606. ISBN 978-1-4503-5620-6.
- Pimentel, João Felipe; Murta, Leonardo; Braganholo, Vanessa; Freire, Juliana (2019-05-26). "A large-scale study about quality and reproducibility of jupyter notebooks". Proceedings of the 16th International Conference on Mining Software Repositories. MSR '19. Montreal, Quebec, Canada. pp. 507–517. doi:10.1109/MSR.2019.00077.
- OECD (2015-10-15). Making Open Science a Reality (Report). Retrieved 2022-06-13.
- Pellizzari, Edo D.; Lohr, Kathleen N.; Blatecky, Alan; Creel, Darryl V. (2017-08-29). Reproducibility: A Primer on Semantics and Implications for Research. RTI Press.
Other sources
[edit]- Baker, Monya (2016). "Dutch agency launches first grants programme dedicated to replication". Nature News. doi:10.1038/nature.2016.20287. S2CID 114978507. Retrieved 2020-02-10.
- Psomopoulos, Fotis E. Reproducible analysis and Research Transparency — Reproducible Research workshop 1.0 documentation. Retrieved 2020-02-10.