Financial economics
Part of a series on |
Economics |
---|
![]() |
Financial economics is the branch of economics characterized by a "concentration on monetary activities", in which "money of one type or another is likely to appear on both sides of a trade".[1] Its concern is thus the interrelation of financial variables, such as share prices, interest rates and exchange rates, as opposed to those concerning the real economy. It has two main areas of focus:[2] asset pricing and corporate finance; the first being the perspective of providers of capital, i.e. investors, and the second of users of capital. It thus provides the theoretical underpinning for much of finance.
The subject is concerned with "the allocation and deployment of economic resources, both spatially and across time, in an uncertain environment".[3][4] It therefore centers on decision making under uncertainty in the context of the financial markets, and the resultant economic and financial models and principles, and is concerned with deriving testable or policy implications from acceptable assumptions. It thus also includes a formal study of the financial markets themselves, especially market microstructure and market regulation. It is built on the foundations of microeconomics and decision theory.
Financial econometrics is the branch of financial economics that uses econometric techniques to parameterise the relationships identified. Mathematical finance is related in that it will derive and extend the mathematical or numerical models suggested by financial economics. Whereas financial economics has a primarily microeconomic focus, monetary economics is primarily macroeconomic in nature.
Underlying economics
[edit]Fundamental valuation equation [5] |
Four equivalent formulations,[6] where:
|
Financial economics studies how rational investors would apply decision theory to investment management. The subject is thus built on the foundations of microeconomics and derives several key results for the application of decision making under uncertainty to the financial markets. The underlying economic logic yields the fundamental theorem of asset pricing, which gives the conditions for arbitrage-free asset pricing.[6][5] The various "fundamental" valuation formulae result directly.
Present value, expectation and utility
[edit]Underlying all of financial economics are the concepts of present value and expectation.[6]
Calculating their present value, in the first formula, allows the decision maker to aggregate the cashflows (or other returns) to be produced by the asset in the future to a single value at the date in question, and to thus more readily compare two opportunities; this concept is then the starting point for financial decision making. [note 1] (Note that here, "" represents a generic (or arbitrary) discount rate applied to the cash flows, whereas in the valuation formulae, the risk-free rate is applied once these have been "adjusted" for their riskiness; see below.)
An immediate extension is to combine probabilities with present value, leading to the expected value criterion which sets asset value as a function of the sizes of the expected payouts and the probabilities of their occurrence, and respectively. [note 2]
This decision method, however, fails to consider risk aversion. In other words, since individuals receive greater utility from an extra dollar when they are poor and less utility when comparatively rich, the approach is therefore to "adjust" the weight assigned to the various outcomes, i.e. "states", correspondingly: . See indifference price. (Some investors may in fact be risk seeking as opposed to risk averse, but the same logic would apply.)
Choice under uncertainty here may then be defined as the maximization of expected utility. More formally, the resulting expected utility hypothesis states that, if certain axioms are satisfied, the subjective value associated with a gamble by an individual is that individual's statistical expectation of the valuations of the outcomes of that gamble.
The impetus for these ideas arises from various inconsistencies observed under the expected value framework, such as the St. Petersburg paradox and the Ellsberg paradox. [note 3]
Arbitrage-free pricing and equilibrium
[edit]JEL classification codes |
In the Journal of Economic Literature classification codes, Financial Economics is one of the 19 primary classifications, at JEL: G. It follows Monetary and International Economics and precedes Public Economics. For detailed subclassifications see JEL classification codes § G. Financial Economics.
The New Palgrave Dictionary of Economics (2008, 2nd ed.) also uses the JEL codes to classify its entries in v. 8, Subject Index, including Financial Economics at pp. 863–64. The below have links to entry abstracts of The New Palgrave Online for each primary or secondary JEL category (10 or fewer per page, similar to Google searches):
Tertiary category entries can also be searched.[10] |
The concepts of arbitrage-free, "rational", pricing and equilibrium are then coupled [11] with the above to derive various of the "classical"[12] (or "neo-classical"[13]) financial economics models.
Rational pricing is the assumption that asset prices (and hence asset pricing models) will reflect the arbitrage-free price of the asset, as any deviation from this price will be "arbitraged away". This assumption is useful in pricing fixed income securities, particularly bonds, and is fundamental to the pricing of derivative instruments.
Economic equilibrium is a state in which economic forces such as supply and demand are balanced, and in the absence of external influences these equilibrium values of economic variables will not change. General equilibrium deals with the behavior of supply, demand, and prices in a whole economy with several or many interacting markets, by seeking to prove that a set of prices exists that will result in an overall equilibrium. (This is in contrast to partial equilibrium, which only analyzes single markets.)
The two concepts are linked as follows: where market prices do not allow profitable arbitrage, i.e. they comprise an arbitrage-free market, then these prices are also said to constitute an "arbitrage equilibrium". Intuitively, this may be seen by considering that where an arbitrage opportunity does exist, then prices can be expected to change, and they are therefore not in equilibrium.[14] An arbitrage equilibrium is thus a precondition for a general economic equilibrium.
"Complete" here means that there is a price for every asset in every possible state of the world, , and that the complete set of possible bets on future states-of-the-world can therefore be constructed with existing assets (assuming no friction): essentially solving simultaneously for n (risk-neutral) probabilities, , given n prices. For a simplified example see Rational pricing § Risk neutral valuation, where the economy has only two possible states – up and down – and where and (=) are the two corresponding probabilities, and in turn, the derived distribution, or "measure".
The formal derivation will proceed by arbitrage arguments.[6][14][11]The analysis here is often undertaken assuming a representative agent,[15] essentially treating all market participants, "agents", as identical (or, at least, assuming that they act in such a way that the sum of their choices is equivalent to the decision of one individual) with the effect that the problems are then mathematically tractable.
With this measure in place, the expected, i.e. required, return of any security (or portfolio) will then equal the risk-free return, plus an "adjustment for risk",[6] i.e. a security-specific risk premium, compensating for the extent to which its cashflows are unpredictable. All pricing models are then essentially variants of this, given specific assumptions or conditions.[6][5][16] This approach is consistent with the above, but with the expectation based on "the market" (i.e. arbitrage-free, and, per the theorem, therefore in equilibrium) as opposed to individual preferences.
Continuing the example, in pricing a derivative instrument, its forecasted cashflows in the above-mentioned up- and down-states and , are multiplied through by and , and are then discounted at the risk-free interest rate; per the second equation above. In pricing a "fundamental", underlying, instrument (in equilibrium), on the other hand, a risk-appropriate premium over risk-free is required in the discounting, essentially employing the first equation with and combined. This premium may be derived by the CAPM (or extensions) as will be seen under § Uncertainty.
The difference is explained as follows: By construction, the value of the derivative will (must) grow at the risk free rate, and, by arbitrage arguments, its value must then be discounted correspondingly; in the case of an option, this is achieved by "manufacturing" the instrument as a combination of the underlying and a risk free "bond"; see Rational pricing § Delta hedging (and § Uncertainty below). Where the underlying is itself being priced, such "manufacturing" is of course not possible – the instrument being "fundamental", i.e. as opposed to "derivative" – and a premium is then required for risk.
(Correspondingly, mathematical finance separates into two analytic regimes: risk and portfolio management (generally) use physical (or actual or actuarial) probability, denoted by "P"; while derivatives pricing uses risk-neutral probability (or arbitrage-pricing probability), denoted by "Q". In specific applications the lower case is used, as in the above equations.)
State prices
[edit]With the above relationship established, the further specialized Arrow–Debreu model may be derived. [note 4] This result suggests that, under certain economic conditions, there must be a set of prices such that aggregate supplies will equal aggregate demands for every commodity in the economy. The Arrow–Debreu model applies to economies with maximally complete markets, in which there exists a market for every time period and forward prices for every commodity at all time periods.
A direct extension, then, is the concept of a state price security, also called an Arrow–Debreu security, a contract that agrees to pay one unit of a numeraire (a currency or a commodity) if a particular state occurs ("up" and "down" in the simplified example above) at a particular time in the future and pays zero numeraire in all the other states. The price of this security is the state price of this particular state of the world; also referred to as a "Risk Neutral Density".[20]
In the above example, the state prices, , would equate to the present values of and : i.e. what one would pay today, respectively, for the up- and down-state securities; the state price vector is the vector of state prices for all states. Applied to derivative valuation, the price today would simply be [× + ×]: the fourth formula (see above regarding the absence of a risk premium here). For a continuous random variable indicating a continuum of possible states, the value is found by integrating over the state price "density".
State prices find immediate application as a conceptual tool ("contingent claim analysis");[6] but can also be applied to valuation problems.[21] Given the pricing mechanism described, one can decompose the derivative value – true in fact for "every security"[2] – as a linear combination of its state-prices; i.e. back-solve for the state-prices corresponding to observed derivative prices.[22][21] [20] These recovered state-prices can then be used for valuation of other instruments with exposure to the underlyer, or for other decision making relating to the underlyer itself.
Using the related stochastic discount factor - also called the pricing kernel - the asset price is computed by "discounting" the future cash flow by the stochastic factor , and then taking the expectation;[16] the third equation above. Essentially, this factor divides expected utility at the relevant future period - a function of the possible asset values realized under each state - by the utility due to today's wealth, and is then also referred to as "the intertemporal marginal rate of substitution".
Resultant models
[edit]
Bond valuation formula where Coupons and Face value are discounted at the appropriate rate, "i": typically a spread over the (per period) risk free rate as a function of credit risk; often quoted as a "yield to maturity". See body for discussion re the relationship with the above pricing formulae. |
DCF valuation formula, where the value of the firm, is its forecasted free cash flows discounted to the present using the weighted average cost of capital, i.e. cost of equity and cost of debt, with the former (often) derived using the below CAPM. For share valuation investors use the related dividend discount model. |



The capital asset pricing model (CAPM):
The expected return used when discounting cashflows on an asset , is the risk-free rate plus the market premium multiplied by beta (), the asset's correlated volatility relative to the overall market . |


The Black–Scholes equation:
|
The Black–Scholes formula for the value of a call option:
|
Applying the above economic concepts, we may then derive various economic- and financial models and principles. As above, the two usual areas of focus are Asset Pricing and Corporate Finance, the first being the perspective of providers of capital, the second of users of capital. Here, and for (almost) all other financial economics models, the questions addressed are typically framed in terms of "time, uncertainty, options, and information",[1][15] as will be seen below.
- Time: money now is traded for money in the future.
- Uncertainty (or risk): The amount of money to be transferred in the future is uncertain.
- Options: one party to the transaction can make a decision at a later time that will affect subsequent transfers of money.
- Information: knowledge of the future can reduce, or possibly eliminate, the uncertainty associated with future monetary value (FMV).
Applying this framework, with the above concepts, leads to the required models. This derivation begins with the assumption of "no uncertainty" and is then expanded to incorporate the other considerations.[4] (This division sometimes denoted "deterministic" and "random",[23] or "stochastic".)
Certainty
[edit]The starting point here is "Investment under certainty", and usually framed in the context of a corporation. The Fisher separation theorem, asserts that the objective of the corporation will be the maximization of its present value, regardless of the preferences of its shareholders. Related is the Modigliani–Miller theorem, which shows that, under certain conditions, the value of a firm is unaffected by how that firm is financed, and depends neither on its dividend policy nor its decision to raise capital by issuing stock or selling debt. The proof here proceeds using arbitrage arguments, and acts as a benchmark [11] for evaluating the effects of factors outside the model that do affect value. [note 5]
The mechanism for determining (corporate) value is provided by [26] [27] John Burr Williams' The Theory of Investment Value, which proposes that the value of an asset should be calculated using "evaluation by the rule of present worth". Thus, for a common stock, the "intrinsic", long-term worth is the present value of its future net cashflows, in the form of dividends. What remains to be determined is the appropriate discount rate. Later developments show that, "rationally", i.e. in the formal sense, the appropriate discount rate here will (should) depend on the asset's riskiness relative to the overall market, as opposed to its owners' preferences; see below. Net present value (NPV) is the direct extension of these ideas typically applied to Corporate Finance decisioning. For other results, as well as specific models developed here, see the list of "Equity valuation" topics under Outline of finance § Discounted cash flow valuation. [note 6]
Bond valuation, in that cashflows (coupons and return of principal, or "Face value") are deterministic, may proceed in the same fashion.[23] An immediate extension, Arbitrage-free bond pricing, discounts each cashflow at the market derived rate – i.e. at each coupon's corresponding zero rate, and of equivalent credit worthiness – as opposed to an overall rate. In many treatments bond valuation precedes equity valuation, under which cashflows (dividends) are not "known" per se. Williams and onward allow for forecasting as to these – based on historic ratios or published dividend policy – and cashflows are then treated as essentially deterministic; see below under § Corporate finance theory.
For both stocks and bonds, "under certainty, with the focus on cash flows from securities over time," valuation based on a term structure of interest rates is in fact consistent with arbitrage-free pricing.[28] Indeed, a corollary of the above is that "the law of one price implies the existence of a discount factor"; [29] correspondingly, as formulated, .
Whereas these "certainty" results are all commonly employed under corporate finance, uncertainty is the focus of "asset pricing models" as follows. Fisher's formulation of the theory here - developing an intertemporal equilibrium model - underpins also [26] the below applications to uncertainty; [note 7] see [30] for the development.
Uncertainty
[edit]For "choice under uncertainty" the twin assumptions of rationality and market efficiency, as more closely defined, lead to modern portfolio theory (MPT) with its capital asset pricing model (CAPM) – an equilibrium-based result – and to the Black–Scholes–Merton theory (BSM; often, simply Black–Scholes) for option pricing – an arbitrage-free result. As above, the (intuitive) link between these, is that the latter derivative prices are calculated such that they are arbitrage-free with respect to the more fundamental, equilibrium determined, securities prices; see Asset pricing § Interrelationship.
Briefly, and intuitively – and consistent with § Arbitrage-free pricing and equilibrium above – the relationship between rationality and efficiency is as follows.[31] Given the ability to profit from private information, self-interested traders are motivated to acquire and act on their private information. In doing so, traders contribute to more and more "correct", i.e. efficient, prices: the efficient-market hypothesis, or EMH. Thus, if prices of financial assets are (broadly) efficient, then deviations from these (equilibrium) values could not last for long. (See earnings response coefficient.) The EMH (implicitly) assumes that average expectations constitute an "optimal forecast", i.e. prices using all available information are identical to the best guess of the future: the assumption of rational expectations. The EMH does allow that when faced with new information, some investors may overreact and some may underreact, [32] but what is required, however, is that investors' reactions follow a normal distribution – so that the net effect on market prices cannot be reliably exploited [32] to make an abnormal profit. In the competitive limit, then, market prices will reflect all available information and prices can only move in response to news: [33] the random walk hypothesis. This news, of course, could be "good" or "bad", minor or, less common, major; and these moves are then, correspondingly, normally distributed; with the price therefore following a log-normal distribution. [note 8]
Under these conditions, investors can then be assumed to act rationally: their investment decision must be calculated or a loss is sure to follow; [32] correspondingly, where an arbitrage opportunity presents itself, then arbitrageurs will exploit it, reinforcing this equilibrium. Here, as under the certainty-case above, the specific assumption as to pricing is that prices are calculated as the present value of expected future dividends, [5] [33] [15] as based on currently available information. What is required though, is a theory for determining the appropriate discount rate, i.e. "required return", given this uncertainty: this is provided by the MPT and its CAPM. Relatedly, rationality – in the sense of arbitrage-exploitation – gives rise to Black–Scholes; option values here ultimately consistent with the CAPM.
In general, then, while portfolio theory studies how investors should balance risk and return when investing in many assets or securities, the CAPM is more focused, describing how, in equilibrium, markets set the prices of assets in relation to how risky they are. [note 9] This result will be independent of the investor's level of risk aversion and assumed utility function, thus providing a readily determined discount rate for corporate finance decision makers as above,[36] and for other investors. The argument proceeds as follows: [37] If one can construct an efficient frontier – i.e. each combination of assets offering the best possible expected level of return for its level of risk, see diagram – then mean-variance efficient portfolios can be formed simply as a combination of holdings of the risk-free asset and the "market portfolio" (the Mutual fund separation theorem), with the combinations here plotting as the capital market line, or CML. Then, given this CML, the required return on a risky security will be independent of the investor's utility function, and solely determined by its covariance ("beta") with aggregate, i.e. market, risk. This is because investors here can then maximize utility through leverage as opposed to pricing; see Separation property (finance), Markowitz model § Choosing the best portfolio and CML diagram aside. As can be seen in the formula aside, this result is consistent with the preceding, equaling the riskless return plus an adjustment for risk.[5] A more modern, direct, derivation is as described at the bottom of this section; which can be generalized to derive other equilibrium-pricing models.
Black–Scholes provides a mathematical model of a financial market containing derivative instruments, and the resultant formula for the price of European-styled options. [note 10] The model is expressed as the Black–Scholes equation, a partial differential equation describing the changing price of the option over time; it is derived assuming log-normal, geometric Brownian motion (see Brownian model of financial markets). The key financial insight behind the model is that one can perfectly hedge the option by buying and selling the underlying asset in just the right way and consequently "eliminate risk", absenting the risk adjustment from the pricing (, the value, or price, of the option, grows at , the risk-free rate).[6][5] This hedge, in turn, implies that there is only one right price – in an arbitrage-free sense – for the option. And this price is returned by the Black–Scholes option pricing formula. (The formula, and hence the price, is consistent with the equation, as the formula is the solution to the equation.) Since the formula is without reference to the share's expected return, Black–Scholes inheres risk neutrality; intuitively consistent with the "elimination of risk" here, and mathematically consistent with § Arbitrage-free pricing and equilibrium above. Relatedly, therefore, the pricing formula may also be derived directly via risk neutral expectation. Itô's lemma provides the underlying mathematics, and, with Itô calculus more generally, remains fundamental in quantitative finance. [note 11]
As implied by the Fundamental Theorem, the two major results are consistent. Here, the Black Scholes equation can alternatively be derived from the CAPM, and the price obtained from the Black–Scholes model is thus consistent with the assumptions of the CAPM.[45][13] The Black–Scholes theory, although built on Arbitrage-free pricing, is therefore consistent with the equilibrium based capital asset pricing. Both models, in turn, are ultimately consistent with the Arrow–Debreu theory, and can be derived via state-pricing – essentially, by expanding the fundamental result above – further explaining, and if required demonstrating, this consistency. [6] Here, the CAPM is derived by linking , risk aversion, to overall market return, and setting the return on security as ; see Stochastic discount factor § Properties. The Black-Scholes formula is found, in the limit, by attaching a binomial probability[11] to each of numerous possible spot-prices (i.e. states) and then rearranging for the terms corresponding to and , per the boxed description; see Binomial options pricing model § Relationship with Black–Scholes.
Extensions
[edit]More recent work further generalizes and extends these models. As regards asset pricing, developments in equilibrium-based pricing are discussed under "Portfolio theory" below, while "Derivative pricing" relates to risk-neutral, i.e. arbitrage-free, pricing. As regards the use of capital, "Corporate finance theory" relates, mainly, to the application of these models.
Portfolio theory
[edit]

The majority of developments here relate to required return, i.e. pricing, extending the basic CAPM. Multi-factor models such as the Fama–French three-factor model and the Carhart four-factor model, propose factors other than market return as relevant in pricing. The intertemporal CAPM and consumption-based CAPM similarly extend the model. With intertemporal portfolio choice, the investor now repeatedly optimizes her portfolio; while the inclusion of consumption (in the economic sense) then incorporates all sources of wealth, and not just market-based investments, into the investor's calculation of required return.
Whereas the above extend the CAPM, the single-index model is a more simple model. It assumes, only, a correlation between security and market returns, without (numerous) other economic assumptions. It is useful in that it simplifies the estimation of correlation between securities, significantly reducing the inputs for building the correlation matrix required for portfolio optimization. The arbitrage pricing theory (APT) similarly differs as regards its assumptions. APT "gives up the notion that there is one right portfolio for everyone in the world, and ...replaces it with an explanatory model of what drives asset returns."[46] It returns the required (expected) return of a financial asset as a linear function of various macro-economic factors, and assumes that arbitrage should bring incorrectly priced assets back into line.[note 12] The linear factor model structure of the APT is used as the basis for many of the commercial risk systems employed by asset managers.
As regards portfolio optimization, the Black–Litterman model[49] departs from the original Markowitz model – i.e. of constructing portfolios via an efficient frontier. Black–Litterman instead starts with an equilibrium assumption, and is then modified to take into account the 'views' (i.e., the specific opinions about asset returns) of the investor in question to arrive at a bespoke [50] asset allocation. Where factors additional to volatility are considered (kurtosis, skew...) then multiple-criteria decision analysis can be applied; here deriving a Pareto efficient portfolio. The universal portfolio algorithm applies machine learning to asset selection, learning adaptively from historical data. Behavioral portfolio theory recognizes that investors have varied aims and create an investment portfolio that meets a broad range of goals. Copulas have lately been applied here; recently this is the case also for genetic algorithms and Machine learning, more generally. (Tail) risk parity focuses on allocation of risk, rather than allocation of capital. [note 13] See Portfolio optimization § Improving portfolio optimization for other techniques and objectives, and Financial risk management § Investment management for discussion.
Derivative pricing
[edit]
PDE for a zero-coupon bond:
Interpretation: Analogous to Black-Scholes, [51] arbitrage arguments describe the instantaneous change in the bond price for changes in the (risk-free) short rate ; the analyst selects the specific short-rate model to be employed. |

In pricing derivatives, the binomial options pricing model provides a discretized version of Black–Scholes, useful for the valuation of American styled options. Discretized models of this type are built – at least implicitly – using state-prices (as above); relatedly, a large number of researchers have used options to extract state-prices for a variety of other applications in financial economics.[6][45][22] For path dependent derivatives, Monte Carlo methods for option pricing are employed; here the modelling is in continuous time, but similarly uses risk neutral expected value. Various other numeric techniques have also been developed. The theoretical framework too has been extended such that martingale pricing is now the standard approach. [note 14]
Drawing on these techniques, models for various other underlyings and applications have also been developed, all based on the same logic (using "contingent claim analysis"). Real options valuation allows that option holders can influence the option's underlying; models for employee stock option valuation explicitly assume non-rationality on the part of option holders; Credit derivatives allow that payment obligations or delivery requirements might not be honored. Exotic derivatives are now routinely valued. Multi-asset underlyers are handled via simulation or copula based analysis.
Similarly, the various short-rate models allow for an extension of these techniques to fixed income- and interest rate derivatives. (The Vasicek and CIR models are equilibrium-based, while Ho–Lee and subsequent models are based on arbitrage-free pricing.) The more general HJM Framework describes the dynamics of the full forward-rate curve – as opposed to working with short rates – and is then more widely applied. The valuation of the underlying instrument – additional to its derivatives – is relatedly extended, particularly for hybrid securities, where credit risk is combined with uncertainty re future rates; see Bond valuation § Stochastic calculus approach and Lattice model (finance) § Hybrid securities. [note 15]
Following the Crash of 1987, equity options traded in American markets began to exhibit what is known as a "volatility smile"; that is, for a given expiration, options whose strike price differs substantially from the underlying asset's price command higher prices, and thus implied volatilities, than what is suggested by BSM. (The pattern differs across various markets.) Modelling the volatility smile is an active area of research, and developments here – as well as implications re the standard theory – are discussed in the next section.
After the 2007–2008 financial crisis, a further development:[60] as outlined, (over the counter) derivative pricing had relied on the BSM risk neutral pricing framework, under the assumptions of funding at the risk free rate and the ability to perfectly replicate cashflows so as to fully hedge. This, in turn, is built on the assumption of a credit-risk-free environment – called into question during the crisis. Addressing this, therefore, issues such as counterparty credit risk, funding costs and costs of capital are now additionally considered when pricing,[61] and a credit valuation adjustment, or CVA – and potentially other valuation adjustments, collectively xVA – is generally added to the risk-neutral derivative value. The standard economic arguments can be extended to incorporate these various adjustments.[62]
A related, and perhaps more fundamental change, is that discounting is now on the Overnight Index Swap (OIS) curve, as opposed to LIBOR as used previously.[60] This is because post-crisis, the overnight rate is considered a better proxy for the "risk-free rate".[63] (Also, practically, the interest paid on cash collateral is usually the overnight rate; OIS discounting is then, sometimes, referred to as "CSA discounting".) Swap pricing – and, therefore, yield curve construction – is further modified: previously, swaps were valued off a single "self discounting" interest rate curve; whereas post crisis, to accommodate OIS discounting, valuation is now under a "multi-curve framework" where "forecast curves" are constructed for each floating-leg LIBOR tenor, with discounting on the common OIS curve.
Corporate finance theory
[edit]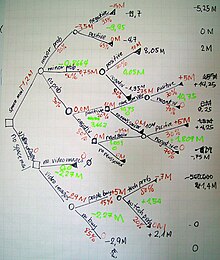
Mirroring the above developments, asset-valuation and decisioning no longer need assume "certainty". Monte Carlo methods in finance allow financial analysts to construct "stochastic" or probabilistic corporate finance models, as opposed to the traditional static and deterministic models;[64] see Corporate finance § Quantifying uncertainty. Relatedly, Real Options theory allows for owner – i.e. managerial – actions that impact underlying value: by incorporating option pricing logic, these actions are then applied to a distribution of future outcomes, changing with time, which then determine the "project's" valuation today.[65] More traditionally, decision trees – which are complementary – have been used to evaluate projects, by incorporating in the valuation (all) possible events (or states) and consequent management decisions;[66][64] the correct discount rate here reflecting each decision-point's "non-diversifiable risk looking forward."[64] [note 16]
Related to this, is the treatment of forecasted cashflows in equity valuation. In many cases, following Williams above, the average (or most likely) cash-flows were discounted,[68] as opposed to a theoretically correct state-by-state treatment under uncertainty; see comments under Financial modeling § Accounting. In more modern treatments, then, it is the expected cashflows (in the mathematical sense: ) combined into an overall value per forecast period which are discounted. [69] [70] [71] [64] And using the CAPM – or extensions – the discounting here is at the risk-free rate plus a premium linked to the uncertainty of the entity or project cash flows [64] (essentially, and combined).
Other developments here include[72] agency theory, which analyses the difficulties in motivating corporate management (the "agent"; in a different sense to the above) to act in the best interests of shareholders (the "principal"), rather than in their own interests; here emphasizing the issues interrelated with capital structure. [73] Clean surplus accounting and the related residual income valuation provide a model that returns price as a function of earnings, expected returns, and change in book value, as opposed to dividends. This approach, to some extent, arises due to the implicit contradiction of seeing value as a function of dividends, while also holding that dividend policy cannot influence value per Modigliani and Miller's "Irrelevance principle"; see Dividend policy § Relevance of dividend policy.
"Corporate finance" as a discipline more generally, per Fisher above, relates to the long term objective of maximizing the value of the firm - and its return to shareholders - and thus also incorporates the areas of capital structure and dividend policy. [74] Extensions of the theory here then also consider these latter, as follows: (i) optimization re capitalization structure, and theories here as to corporate choices and behavior: Capital structure substitution theory, Pecking order theory, Market timing hypothesis, Trade-off theory; (ii) considerations and analysis re dividend policy, additional to - and sometimes contrasting with - Modigliani-Miller, include: the Walter model, Lintner model, Residuals theory and signaling hypothesis, as well as discussion re the observed clientele effect and dividend puzzle.
As described, the typical application of real options is to capital budgeting type problems. However, here, they are also applied to problems of capital structure and dividend policy, and to the related design of corporate securities; [75] and since stockholder and bondholders have different objective functions, in the analysis of the related agency problems. [65] In all of these cases, state-prices can provide the market-implied information relating to the corporate, as above, which is then applied to the analysis. For example, convertible bonds can (must) be priced consistent with the (recovered) state-prices of the corporate's equity.[21][69]
Financial markets
[edit]The discipline, as outlined, also includes a formal study of financial markets. Of interest especially are market regulation and market microstructure, and their relationship to price efficiency.
Regulatory economics studies, in general, the economics of regulation. In the context of finance, it will address the impact of financial regulation on the functioning of markets and the efficiency of prices, while also weighing the corresponding increases in market confidence and financial stability. Research here considers how, and to what extent, regulations relating to disclosure (earnings guidance, annual reports), insider trading, and short-selling will impact price efficiency, the cost of equity, and market liquidity.[76]
Market microstructure is concerned with the details of how exchange occurs in markets (with Walrasian-, matching-, Fisher-, and Arrow-Debreu markets as prototypes), and "analyzes how specific trading mechanisms affect the price formation process",[77] examining the ways in which the processes of a market affect determinants of transaction costs, prices, quotes, volume, and trading behavior. It has been used, for example, in providing explanations for long-standing exchange rate puzzles,[78] and for the equity premium puzzle.[79] In contrast to the above classical approach, models here explicitly allow for (testing the impact of) market frictions and other imperfections; see also market design.
For both regulation [80] and microstructure,[81] and generally,[82] agent-based models can be developed [83] to examine any impact due to a change in structure or policy - or to make inferences re market dynamics - by testing these in an artificial financial market, or AFM. [note 17] This approach, essentially simulated trade between numerous agents, "typically uses artificial intelligence technologies [often genetic algorithms and neural nets] to represent the adaptive behaviour of market participants".[83]
These 'bottom-up' models "start from first principals of agent behavior",[84] with participants modifying their trading strategies having learned over time, and "are able to describe macro features [i.e. stylized facts] emerging from a soup of individual interacting strategies".[84] Agent-based models depart further from the classical approach — the representative agent, as outlined — in that they introduce heterogeneity into the environment (thereby addressing, also, the aggregation problem).
Challenges and criticism
[edit]As above, there is a very close link between: the random walk hypothesis, with the associated belief that price changes should follow a normal distribution, on the one hand; and market efficiency and rational expectations, on the other. Wide departures from these are commonly observed, and there are thus, respectively, two main sets of challenges.
Departures from normality
[edit]
As discussed, the assumptions that market prices follow a random walk and that asset returns are normally distributed are fundamental. Empirical evidence, however, suggests that these assumptions may not hold, and that in practice, traders, analysts and risk managers frequently modify the "standard models" (see Kurtosis risk, Skewness risk, Long tail, Model risk). In fact, Benoit Mandelbrot had discovered already in the 1960s [85] that changes in financial prices do not follow a normal distribution, the basis for much option pricing theory, although this observation was slow to find its way into mainstream financial economics. [86]
Financial models with long-tailed distributions and volatility clustering have been introduced to overcome problems with the realism of the above "classical" financial models; while jump diffusion models allow for (option) pricing incorporating "jumps" in the spot price.[87] Risk managers, similarly, complement (or substitute) the standard value at risk models with historical simulations, mixture models, principal component analysis, extreme value theory, as well as models for volatility clustering.[88] For further discussion see Fat-tailed distribution § Applications in economics, and Value at risk § Criticism. Portfolio managers, likewise, have modified their optimization criteria and algorithms; see § Portfolio theory above.
Closely related is the volatility smile, where, as above, implied volatility – the volatility corresponding to the BSM price – is observed to differ as a function of strike price (i.e. moneyness), true only if the price-change distribution is non-normal, unlike that assumed by BSM. The term structure of volatility describes how (implied) volatility differs for related options with different maturities. An implied volatility surface is then a three-dimensional surface plot of volatility smile and term structure. These empirical phenomena negate the assumption of constant volatility – and log-normality – upon which Black–Scholes is built.[40][87] Within institutions, the function of Black-Scholes is now, largely, to communicate prices via implied volatilities, much like bond prices are communicated via YTM; see Black–Scholes model § The volatility smile.
In consequence traders (and risk managers) now, instead, use "smile-consistent" models, firstly, when valuing derivatives not directly mapped to the surface, facilitating the pricing of other, i.e. non-quoted, strike/maturity combinations, or of non-European derivatives, and generally for hedging purposes. The two main approaches are local volatility and stochastic volatility. The first returns the volatility which is "local" to each spot-time point of the finite difference- or simulation-based valuation; i.e. as opposed to implied volatility, which holds overall. In this way calculated prices – and numeric structures – are market-consistent in an arbitrage-free sense. The second approach assumes that the volatility of the underlying price is a stochastic process rather than a constant. Models here are first calibrated to observed prices, and are then applied to the valuation or hedging in question; the most common are Heston, SABR and CEV. This approach addresses certain problems identified with hedging under local volatility.[89]
Related to local volatility are the lattice-based implied-binomial and -trinomial trees – essentially a discretization of the approach – which are similarly, but less commonly,[20] used for pricing; these are built on state-prices recovered from the surface. Edgeworth binomial trees allow for a specified (i.e. non-Gaussian) skew and kurtosis in the spot price; priced here, options with differing strikes will return differing implied volatilities, and the tree can be calibrated to the smile as required.[90] Similarly purposed (and derived) closed-form models were also developed. [91]
As discussed, additional to assuming log-normality in returns, "classical" BSM-type models also (implicitly) assume the existence of a credit-risk-free environment, where one can perfectly replicate cashflows so as to fully hedge, and then discount at "the" risk-free-rate. And therefore, post crisis, the various x-value adjustments must be employed, effectively correcting the risk-neutral value for counterparty- and funding-related risk. These xVA are additional to any smile or surface effect. This is valid as the surface is built on price data relating to fully collateralized positions, and there is therefore no "double counting" of credit risk (etc.) when appending xVA. (Were this not the case, then each counterparty would have its own surface...)
As mentioned at top, mathematical finance (and particularly financial engineering) is more concerned with mathematical consistency (and market realities) than compatibility with economic theory, and the above "extreme event" approaches, smile-consistent modeling, and valuation adjustments should then be seen in this light. Recognizing this, critics of financial economics - especially vocal since the 2007–2008 financial crisis - suggest that instead, the theory needs revisiting almost entirely: [note 18]
- "The current system, based on the idea that risk is distributed in the shape of a bell curve, is flawed... The problem is [that economists and practitioners] never abandon the bell curve. They are like medieval astronomers who believe the sun revolves around the earth and are furiously tweaking their geo-centric math in the face of contrary evidence. They will never get this right; they need their Copernicus."[92]
Departures from rationality
[edit]Market anomalies and economic puzzles |
As seen, a common assumption is that financial decision makers act rationally; see Homo economicus. Recently, however, researchers in experimental economics and experimental finance have challenged this assumption empirically. These assumptions are also challenged theoretically, by behavioral finance, a discipline primarily concerned with the limits to rationality of economic agents. [note 19] For related criticisms re corporate finance theory vs its practice see:.[93] Various persistent market anomalies have also been documented as consistent with and complementary to price or return distortions – e.g. size premiums – which appear to contradict the efficient-market hypothesis. Within these market anomalies, calendar effects are the most commonly referenced group.
Related to these are various of the economic puzzles, concerning phenomena similarly contradicting the theory. The equity premium puzzle, as one example, arises in that the difference between the observed returns on stocks as compared to government bonds is consistently higher than the risk premium rational equity investors should demand, an "abnormal return". For further context see Random walk hypothesis § A non-random walk hypothesis, and sidebar for specific instances.
More generally, and, again, particularly following the 2007–2008 financial crisis, financial economics and mathematical finance have been subjected to deeper criticism; notable here is Nassim Nicholas Taleb, who claims that the prices of financial assets cannot be characterized by the simple models currently in use, rendering much of current practice at best irrelevant, and, at worst, dangerously misleading; see Black swan theory, Taleb distribution. A topic of general interest has thus been financial crises, [94] and the failure of (financial) economics to model (and predict) these.
A related problem is systemic risk: where companies hold securities in each other then this interconnectedness may entail a "valuation chain" – and the performance of one company, or security, here will impact all, a phenomenon not easily modeled, regardless of whether the individual models are correct. See: Systemic risk § Inadequacy of classic valuation models; Cascades in financial networks; Flight-to-quality.
Areas of research attempting to explain (or at least model) these phenomena, and crises, include[15] noise trading, market microstructure (as above), and Heterogeneous agent models. The latter is extended to agent-based computational models, as mentioned; here [82] price is treated as an emergent phenomenon, resulting from the interaction of the various market participants (agents). The noisy market hypothesis argues that prices can be influenced by speculators and momentum traders, as well as by insiders and institutions that often buy and sell stocks for reasons unrelated to fundamental value; see Noise (economic). The adaptive market hypothesis is an attempt to reconcile the efficient market hypothesis with behavioral economics, by applying the principles of evolution to financial interactions. An information cascade, alternatively, shows market participants engaging in the same acts as others ("herd behavior"), despite contradictions with their private information. Copula-based modelling has similarly been applied. See also Hyman Minsky's "financial instability hypothesis", as well as George Soros' application of "reflexivity".
However, studies show that despite inefficiencies, asset prices generally follow a random walk, making it difficult to consistently outperform market averages and achieve "alpha".[95] As an explanation for these inefficiencies, institutional limits to arbitrage are sometimes referenced (as opposed to factors directly contradictory to the theory). The practical implication is that passive investing (e.g. via low-cost index funds) should, on average, serve better than any other active strategy.[96] [note 20]
See also
[edit]- Category:Finance theories
- Category:Financial models
- Deutsche Bank Prize in Financial Economics – scholarly award
- Finance § Financial theory
- Fischer Black Prize – economics award
- List of financial economics articles – Overview of finance and finance-related topics
- List of financial economists
- List of unsolved problems in economics § Financial economics
- Master of Financial Economics – graduate degree with a major in financial economics
- Monetary economics – Branch of economics covering theories of money
- Outline of economics
- Outline of corporate finance – Overview of corporate finance and corporate finance-related topics
- Outline of finance – Overview of finance and finance-related topics
Historical notes
[edit]- ^ Its history is correspondingly early: Fibonacci developed the concept of present value already in 1202 in his Liber Abaci. Compound interest was discussed in depth by Richard Witt in 1613, in his Arithmeticall Questions,[7] and was further developed by Johan de Witt in 1671 [8] and by Edmond Halley in 1705.[9]
- ^ These ideas originate with Blaise Pascal and Pierre de Fermat in 1654.
- ^ The development here is originally due to Daniel Bernoulli in 1738; it was later formalized by John von Neumann and Oskar Morgenstern in 1947.
- ^ State prices originate with Kenneth Arrow and Gérard Debreu in 1954.[17] Lionel W. McKenzie is also cited for his independent proof of equilibrium existence in 1954.[18] Breeden and Litzenberger's work in 1978[19] established the use of state prices in financial economics.
- ^ The theorem of Franco Modigliani and Merton Miller is often called the "capital structure irrelevance principle"; it is presented in two key papers of 1958,[24] and 1963.[25]
- ^ John Burr Williams published his "Theory" in 1938; NPV was recommended to corporate managers by Joel Dean in 1951.
- ^ In fact, "Fisher (1930, [The Theory of Interest]) is the seminal work for most of the financial theory of investments during the twentieth century… Fisher develops the first formal equilibrium model of an economy with both intertemporal exchange and production. In so doing, at one swoop, he not only derives present value calculations as a natural economic outcome in calculating wealth, he also justifies the maximization of present value as the goal of production and derives determinants of the interest rates that are used to calculate present value."[12]: 55
- ^ The EMH was presented by Eugene Fama in a 1970 review paper,[34] consolidating previous works re random walks in stock prices: Jules Regnault (1863); Louis Bachelier (1900); Maurice Kendall (1953); Paul Cootner (1964); and Paul Samuelson (1965), among others.
- ^ The efficient frontier was introduced by Harry Markowitz in 1952. The CAPM was derived by Jack Treynor (1961, 1962), William F. Sharpe (1964), John Lintner (1965), and Jan Mossin (1966) independently. Already in 1940, Bruno de Finetti[35] had described the mean-variance method, in the context of reinsurance.
- ^ "BSM" – two seminal 1973 papers by Fischer Black and Myron Scholes,[38] and Robert C. Merton[39] – is consistent with "previous versions of the formula" of Louis Bachelier (1900) and Edward O. Thorp (1967);[40] although these were more "actuarial" in flavor, and had not established risk-neutral discounting.[13] Vinzenz Bronzin (1908) produced very early results, also. Case Sprenkle (1961) [41] had published a formula for the price of a call-option which, with adjustments, satisfied the BSM partial differential equation.[42]
- ^ Kiyosi Itô published his Lemma in 1944. Paul Samuelson[43] introduced this area of mathematics into finance in 1965; Robert Merton promoted continuous stochastic calculus and continuous-time processes from 1969. [44]
- ^ The single-index model was developed by William Sharpe in 1963. [47] APT was developed by Stephen Ross in 1976. [48]
- ^ The universal portfolio algorithm was published by Thomas M. Cover in 1991. The Black–Litterman model was developed in 1990 at Goldman Sachs by Fischer Black and Robert Litterman, and published in 1991.
- ^ The binomial model was first proposed by William Sharpe in the 1978 edition of Investments (ISBN 013504605X), and in 1979 formalized by Cox, Ross and Rubinstein [52] and by Rendleman and Bartter. [53] Finite difference methods for option pricing were due to Eduardo Schwartz in 1977.[54] Monte Carlo methods for option pricing were originated by Phelim Boyle in 1977; [55] In 1996, methods were developed for American [56] and Asian options. [57]
- ^ Oldrich Vasicek developed his pioneering short-rate model in 1977. [58] The HJM framework originates from the work of David Heath, Robert A. Jarrow, and Andrew Morton in 1987. [59]
- ^ Simulation was first applied to (corporate) finance by David B. Hertz in 1964. Decision trees, a standard operations research tool, were applied to corporate finance also in the 1960s.[67] Real options in corporate finance were first discussed by Stewart Myers in 1977.
- ^ The Benchmark here is the pioneering AFM of the Santa Fe Institute developed in the early 1990s. See [84] for discussion of other early models.
- ^ This quote, from banker and author James Rickards, is representative. Prominent and earlier criticism [86] is from Benoit Mandelbrot Emanuel Derman, Paul Wilmott, Nassim Taleb, and others. Well known popularizations include Taleb's Fooled by Randomness and The Black Swan, Mandelbrot's The Misbehavior of Markets, and Derman's Models.Behaving.Badly and, with Wimott, the Financial Modelers' Manifesto.
- ^ An early anecdotal treatment is Benjamin Graham's "Mr. Market", discussed in his The Intelligent Investor in 1949. See also John Maynard Keynes' 1936 discussion of "Animal spirits", and the related Keynesian beauty contest, in his General Theory, Ch. 12. Extraordinary Popular Delusions and the Madness of Crowds is a study of crowd psychology by Scottish journalist Charles Mackay, first published in 1841, with Volume I discussing economic bubbles.
- ^ Burton Malkiel's A Random Walk Down Wall Street – first published in 1973, and in its 13th edition as of 2023 – is a widely read popularization of these arguments. See also John C. Bogle's Common Sense on Mutual Funds; but compare Warren Buffett's The Superinvestors of Graham-and-Doddsville.
References
[edit]- ^ a b William F. Sharpe, "Financial Economics" Archived 2004-06-04 at the Wayback Machine, in "Macro-Investment Analysis". Stanford University (manuscript). Archived from the original on 2014-07-14. Retrieved 2009-08-06.
- ^ a b Merton H. Miller, (1999). The History of Finance: An Eyewitness Account, Journal of Portfolio Management. Summer 1999.
- ^ Robert C. Merton "Nobel Lecture" (PDF). Archived (PDF) from the original on 2009-03-19. Retrieved 2009-08-06.
- ^ a b See Fama and Miller (1972), The Theory of Finance, in Bibliography.
- ^ a b c d e f Christopher L. Culp and John H. Cochrane. (2003). ""Equilibrium Asset Pricing and Discount Factors: Overview and Implications for Derivatives Valuation and Risk Management" Archived 2016-03-04 at the Wayback Machine, in Modern Risk Management: A History. Peter Field, ed. London: Risk Books, 2003. ISBN 1904339050
- ^ a b c d e f g h i j Rubinstein, Mark. (2005). "Great Moments in Financial Economics: IV. The Fundamental Theorem (Part I)", Journal of Investment Management, Vol. 3, No. 4, Fourth Quarter 2005;
~ (2006). Part II, Vol. 4, No. 1, First Quarter 2006. (See under "External links".) - ^ C. Lewin (1970). An early book on compound interest Archived 2016-12-21 at the Wayback Machine, Institute and Faculty of Actuaries
- ^ James E. Ciecka. 2008. "The First Mathematically Correct Life Annuity". Journal of Legal Economics 15(1): pp. 59-63
- ^ James E. Ciecka (2008). "Edmond Halley’s Life Table and Its Uses". Journal of Legal Economics 15(1): pp. 65-74.
- ^ For example, http://www.dictionaryofeconomics.com/search_results?q=&field=content&edition=all&topicid=G00 Archived 2013-05-29 at the Wayback Machine.
- ^ a b c d Varian, Hal R. (1987). "The Arbitrage Principle in Financial Economics". Economic Perspectives. 1 (2): 55–72. doi:10.1257/jep.1.2.55. JSTOR 1942981.
- ^ a b See Rubinstein (2006), under "Bibliography".
- ^ a b c Emanuel Derman, A Scientific Approach to CAPM and Options Valuation Archived 2016-03-30 at the Wayback Machine
- ^ a b Freddy Delbaen and Walter Schachermayer. (2004). "What is... a Free Lunch?" Archived 2016-03-04 at the Wayback Machine (pdf). Notices of the AMS 51 (5): 526–528
- ^ a b c d Farmer J. Doyne, Geanakoplos John (2009). "The virtues and vices of equilibrium and the future of financial economics" (PDF). Complexity. 14 (3): 11–38. arXiv:0803.2996. Bibcode:2009Cmplx..14c..11F. doi:10.1002/cplx.20261. S2CID 4506630.
- ^ a b See: David K. Backus (2015). Fundamentals of Asset Pricing, Stern NYU
- ^ Arrow, K. J.; Debreu, G. (1954). "Existence of an equilibrium for a competitive economy". Econometrica. 22 (3): 265–290. doi:10.2307/1907353. JSTOR 1907353.
- ^ McKenzie, Lionel W. (1954). "On Equilibrium in Graham's Model of World Trade and Other Competitive Systems". Econometrica. 22 (2): 147–161. doi:10.2307/1907539. JSTOR 1907539.
- ^ Breeden, Douglas T.; Litzenberger, Robert H. (1978). "Prices of State-Contingent Claims Implicit in Option Prices". Journal of Business. 51 (4): 621–651. doi:10.1086/296025. JSTOR 2352653. S2CID 153841737.
- ^ a b c Figlewski, Stephen (2018). "Risk-Neutral Densities: A Review Annual Review of Financial Economics". Annual Review of Financial Economics. 10: 329–359. doi:10.1146/annurev-financial-110217-022944. S2CID 158075926. SSRN 3120028.
- ^ a b c See de Matos, as well as Bossaerts and Ødegaard, under bibliography.
- ^ a b Don M. Chance (2008). "Option Prices and State Prices" Archived 2012-02-09 at the Wayback Machine
- ^ a b See Luenberger's Investment Science, under Bibliography.
- ^ Modigliani, F.; Miller, M. (1958). "The Cost of Capital, Corporation Finance and the Theory of Investment". American Economic Review. 48 (3): 261–297. JSTOR 1809766.
- ^ Modigliani, F.; Miller, M. (1963). "Corporate income taxes and the cost of capital: a correction". American Economic Review. 53 (3): 433–443. JSTOR 1809167.
- ^ a b The New School. "Finance Theory". Archived from the original on 2006-07-02. Retrieved 2006-06-28.
- ^ Mark Rubinstein (2002). "Great Moments in Financial Economics: I. Present Value". Archived from the original on 2007-07-13. Retrieved 2007-06-28.
- ^ See footnote 3 under Rubinstein (2005). "The Fundamental Theorem (Part I)", refenced below.
- ^ § 4.1 "Law of one price and existence of a discount factor" in Cochrane (2005).
- ^ Gonçalo L. Fonseca (N.D.). Irving Fisher's Theory of Investment. History of Economic Thought series, The New School.
- ^ For a more formal treatment, see, for example: Eugene F. Fama. (1965). "Random Walks in Stock Market Prices". Financial Analysts Journal, September/October 1965, Vol. 21, No. 5: 55–59.
- ^ a b c Mark Rubinstein (2001). "Rational Markets: Yes or No? The Affirmative Case". Financial Analysts Journal, May - Jun., 2001, Vol. 57, No. 3: 15-29
- ^ a b Shiller, Robert J. (2003). "From Efficient Markets Theory to Behavioral Finance" (PDF). Journal of Economic Perspectives. 17 (1 (Winter 2003)): 83–104. doi:10.1257/089533003321164967. Archived (PDF) from the original on 2015-04-12.
- ^ Fama, Eugene (1970). "Efficient Capital Markets: A Review of Theory and Empirical Work". Journal of Finance. Vol. 25, No. 2.
- ^ de Finetti, B. (1940): Il problema dei “Pieni”. Giornale dell’ Istituto Italiano degli Attuari 11, 1–88; translation (Barone, L. (2006)): The problem of full-risk insurances. Chapter I. The risk within a single accounting period. Journal of Investment Management 4(3), 19–43
- ^ Jensen, Michael C. and Smith, Clifford W., "The Theory of Corporate Finance: A Historical Overview". In: The Modern Theory of Corporate Finance, New York: McGraw-Hill Inc., pp. 2–20, 1984.
- ^ See, e.g., Tim Bollerslev (2019). "Risk and Return in Equilibrium: The Capital Asset Pricing Model (CAPM)"
- ^ Black, Fischer; Myron Scholes (1973). "The Pricing of Options and Corporate Liabilities". Journal of Political Economy. 81 (3): 637–654. doi:10.1086/260062. S2CID 154552078. [1]
- ^ Merton, Robert C. (1973). "Theory of Rational Option Pricing" (PDF). Bell Journal of Economics and Management Science. 4 (1): 141–183. doi:10.2307/3003143. hdl:1721.1/49331. JSTOR 3003143. [2]
- ^ a b Haug, E. G. and Taleb, N. N. (2008). Why We Have Never Used the Black-Scholes-Merton Option Pricing Formula, Wilmott Magazine January 2008
- ^ Sprenkle, Case M. (1961). "Warrant prices as indicators of expectations and preferences". Yale Economic Essays. 1 (2): 179–231.
- ^ Black, Fischer MacTutor History of Mathematics Archive
- ^ Samuelson Paul (1965). "A Rational Theory of Warrant Pricing" (PDF). Industrial Management Review. 6: 2. Archived (PDF) from the original on 2017-03-01. Retrieved 2017-02-28.
- ^ Merton, Robert C. "Lifetime Portfolio Selection under Uncertainty: The Continuous-Time Case." The Review of Economics and Statistics 51 (August 1969): 247-257.
- ^ a b Don M. Chance (2008). "Option Prices and Expected Returns" Archived 2015-09-23 at the Wayback Machine
- ^ The Arbitrage Pricing Theory, Chapter VI in Goetzmann, under External links.
- ^ Sharpe, William F. (1963). "A Simplified Model for Portfolio Analysis". Management Science. 9 (2): 277–93. doi:10.1287/mnsc.9.2.277. S2CID 55778045.
- ^ Ross, Stephen A (1976-12-01). "The arbitrage theory of capital asset pricing". Journal of Economic Theory. 13 (3): 341–360. doi:10.1016/0022-0531(76)90046-6. ISSN 0022-0531.
- ^ Black F. and Litterman R. (1991). "Asset Allocation Combining Investor Views with Market Equilibrium". Journal of Fixed Income. September 1991, Vol. 1, No. 2: pp. 7-18
- ^ Guangliang He and Robert Litterman (1999). "The Intuition Behind Black-Litterman Model Portfolios". Goldman Sachs Quantitative Resources Group
- ^ For a derivation see, for example, "Understanding Market Price of Risk" (David Mandel, Florida State University, 2015)
- ^ Cox, J. C.; Ross, S. A.; Rubinstein, M. (1979). "Option pricing: A simplified approach". Journal of Financial Economics. 7 (3): 229. CiteSeerX 10.1.1.379.7582. doi:10.1016/0304-405X(79)90015-1.
- ^ Richard J. Rendleman, Jr. and Brit J. Bartter. 1979. "Two-State Option Pricing". Journal of Finance 24: 1093-1110. doi:10.2307/2327237
- ^ Schwartz, E. (January 1977). "The Valuation of Warrants: Implementing a New Approach". Journal of Financial Economics. 4: 79–94. doi:10.1016/0304-405X(77)90037-X.
- ^ Boyle, Phelim P. (1977). "Options: A Monte Carlo Approach". Journal of Financial Economics. 4 (3): 323–338. doi:10.1016/0304-405x(77)90005-8. Retrieved June 28, 2012.
- ^ Carriere, Jacques (1996). "Valuation of the early-exercise price for options using simulations and nonparametric regression". Insurance: Mathematics and Economics. 19: 19–30. doi:10.1016/S0167-6687(96)00004-2.
- ^ Broadie, M.; Glasserman, P. (1996). "Estimating Security Price Derivatives Using Simulation" (PDF). Management Science. 42 (2): 269–285. CiteSeerX 10.1.1.196.1128. doi:10.1287/mnsc.42.2.269. Retrieved June 28, 2012.
- ^ Vasicek, O. (1977). "An equilibrium characterization of the term structure". Journal of Financial Economics. 5 (2): 177–188. CiteSeerX 10.1.1.164.447. doi:10.1016/0304-405X(77)90016-2.
- ^ David Heath, Robert A. Jarrow, and Andrew Morton (1987). Bond pricing and the term structure of interest rates: a new methodology – working paper, Cornell University
- ^ a b Didier Kouokap Youmbi (2017). "Derivatives Pricing after the 2007-2008 Crisis: How the Crisis Changed the Pricing Approach". Bank of England – Prudential Regulation Authority
- ^ "Post-Crisis Pricing of Swaps using xVAs" Archived 2016-09-17 at the Wayback Machine, Christian Kjølhede & Anders Bech, Master thesis, Aarhus University
- ^ John C. Hull and Alan White (2014). Collateral and Credit Issues in Derivatives Pricing. Rotman School of Management Working Paper No. 2212953
- ^ Hull, John; White, Alan (2013). "LIBOR vs. OIS: The Derivatives Discounting Dilemma". Journal of Investment Management. 11 (3): 14–27.
- ^ a b c d e Aswath Damodaran (2007). "Probabilistic Approaches: Scenario Analysis, Decision Trees and Simulations". In Strategic Risk Taking: A Framework for Risk Management. Prentice Hall. ISBN 0137043775
- ^ a b Damodaran, Aswath (2005). "The Promise and Peril of Real Options" (PDF). NYU Working Paper (S-DRP-05-02). Archived (PDF) from the original on 2001-06-13. Retrieved 2016-12-14.
- ^ Smith, James E.; Nau, Robert F. (1995). "Valuing Risky Projects: Option Pricing Theory and Decision Analysis" (PDF). Management Science. 41 (5): 795–816. doi:10.1287/mnsc.41.5.795. Archived (PDF) from the original on 2010-06-12. Retrieved 2017-08-17.
- ^ See for example: Magee, John F. (1964). "Decision Trees for Decision Making". Harvard Business Review. July 1964: 795–816. Archived from the original on 2017-08-16. Retrieved 2017-08-16.
- ^ Kritzman, Mark (2017). "An Interview with Nobel Laureate Harry M. Markowitz". Financial Analysts Journal. 73 (4): 16–21. doi:10.2469/faj.v73.n4.3. S2CID 158093964.
- ^ a b See Kruschwitz and Löffler under Bibliography.
- ^ "Capital Budgeting Applications and Pitfalls" Archived 2017-08-15 at the Wayback Machine. Ch 13 in Ivo Welch (2017). Corporate Finance: 4th Edition
- ^ George Chacko and Carolyn Evans (2014). Valuation: Methods and Models in Applied Corporate Finance. FT Press. ISBN 0132905221
- ^ See Jensen and Smith under "External links", as well as Rubinstein under "Bibliography".
- ^ Jensen, Michael; Meckling, William (1976). "Theory of the firm: Managerial behavior, agency costs and ownership structure". Journal of Financial Economics. 3 (4): 305–360. doi:10.1016/0304-405X(76)90026-X.
- ^ Corporate Finance: First Principles, from Aswath Damodaran (2022). Applied Corporate Finance: A User's Manual. Wiley. ISBN 978-1118808931
- ^ Kenneth D. Garbade (2001). Pricing Corporate Securities as Contingent Claims. MIT Press. ISBN 9780262072236
- ^ See for example: Hazem Daouk, Charles M.C. Lee, David Ng. (2006). "Capital Market Governance: How Do Security Laws Affect Market Performance?". Journal of Corporate Finance, Volume 12, Issue 3; Emilios Avgouleas (2010). "The Regulation of Short Sales and its Reform" DICE Report, Vol. 8, Iss. 1.
- ^ O'Hara, Maureen, Market Microstructure Theory, Blackwell, Oxford, 1995, ISBN 1-55786-443-8, p.1.
- ^ King, Michael, Osler, Carol and Rime, Dagfinn (2013). "The market microstructure approach to foreign exchange: Looking back and looking forward", Journal of International Money and Finance. Volume 38, November 2013, Pages 95-119
- ^ Randi Næs, Johannes Skjeltorp (2006). "Is the market microstructure of stock markets important?". Norges Bank Economic Bulletin 3/06 (Vol. 77)
- ^ See, e.g., Westerhoff, Frank H. (2008). "The Use of Agent-Based Financial Market Models to Test the Effectiveness of Regulatory Policies", Journal of Economics and Statistics
- ^ See, e.g., Mizuta, Takanobu (2019). "An agent-based model for designing a financial market that works well". 2020 IEEE Symposium Series on Computational Intelligence (SSCI).
- ^ a b For a survey see: LeBaron, Blake (2006). "Agent-based Computational Finance". Handbook of Computational Economics. Elsevier
- ^ a b Katalin Boer, Arie De Bruin, Uzay Kaymak (2005). "On the Design of Artificial Stock Markets". Research In Management ERIM Report Series
- ^ a b c LeBaron, B. (2002). "Building the Santa Fe artificial stock market". Physica A, 1, 20.
- ^ Mandelbrot, Benoit (1963). "The Variation of Certain Speculative Prices" (PDF). The Journal of Business. 36 (Oct): 394–419. doi:10.1086/294632.
- ^ a b Nassim Taleb and Benoit Mandelbrot. "How the Finance Gurus Get Risk All Wrong" (PDF). Archived from the original (PDF) on 2010-12-07. Retrieved 2010-06-15.
- ^ a b Black, Fischer (1989). "How to use the holes in Black-Scholes". Journal of Applied Corporate Finance. 1 (Jan): 67–73. doi:10.1111/j.1745-6622.1989.tb00175.x.
- ^ See for example III.A.3, in Carol Alexander, ed. (January 2005). The Professional Risk Managers' Handbook. PRMIA Publications. ISBN 978-0976609704
- ^ Hagan, Patrick; et al. (2002). "Managing smile risk". Wilmott Magazine (Sep): 84–108.
- ^ See for example Pg 217 of: Jackson, Mary; Mike Staunton (2001). Advanced modelling in finance using Excel and VBA. New Jersey: Wiley. ISBN 0-471-49922-6.
- ^ These include: Jarrow and Rudd (1982); Corrado and Su (1996); Brown and Robinson (2002); Backus, Foresi, and Wu (2004). See, e.g.: E. Jurczenko, B. Maillet, and B. Negrea (2002). "Revisited multi-moment approximate option pricing models: a general comparison (Part 1)". Working paper, London School of Economics and Political Science.
- ^ The Risks of Financial Modeling: VAR and the Economic Meltdown, Hearing before the Subcommittee on Investigations and Oversight, Committee on Science and Technology, House of Representatives, One Hundred Eleventh Congress, first session, September 10, 2009
- ^ Pablo Fernandez (2019). "Common Sense and Illogical Models: Finance and Financial Economics". SSRN 2906887.
- ^ From The New Palgrave Dictionary of Economics, Online Editions, 2011, 2012, with abstract links:
• "regulatory responses to the financial crisis: an interim assessment" Archived 2013-05-29 at the Wayback Machine by Howard Davies
• "Credit Crunch Chronology: April 2007–September 2009" Archived 2013-05-29 at the Wayback Machine by The Statesman's Yearbook team
• "Minsky crisis" Archived 2013-05-29 at the Wayback Machine by L. Randall Wray
• "euro zone crisis 2010" Archived 2013-05-29 at the Wayback Machine by Daniel Gros and Cinzia Alcidi.
• Carmen M. Reinhart and Kenneth S. Rogoff, 2009. This Time Is Different: Eight Centuries of Financial Folly, Princeton. Description Archived 2013-01-18 at the Wayback Machine, ch. 1 ("Varieties of Crises and their Dates". pp. 3-20) Archived 2012-09-25 at the Wayback Machine, and chapter-preview links. - ^ William F. Sharpe (1991). "The Arithmetic of Active Management" Archived 2013-11-13 at the Wayback Machine. Financial Analysts Journal Vol. 47, No. 1, January/February
- ^ William F. Sharpe (2002). Indexed Investing: A Prosaic Way to Beat the Average Investor Archived 2013-11-14 at the Wayback Machine. Presentation: Monterey Institute of International Studies. Retrieved May 20, 2010.
Bibliography
[edit]Financial economics
- Roy E. Bailey (2005). The Economics of Financial Markets. Cambridge University Press. ISBN 978-0521612807.
- Marcelo Bianconi (2013). Financial Economics, Risk and Information (2nd ed.). World Scientific. ISBN 978-9814355131.
- Zvi Bodie, Robert C. Merton and David Cleeton (2008). Financial Economics (2nd ed.). Prentice Hall. ISBN 978-0131856158.
- James Bradfield (2007). Introduction to the Economics of Financial Markets. Oxford University Press. ISBN 978-0-19-531063-4.
- Satya R. Chakravarty (2014). An Outline of Financial Economics. Anthem Press. ISBN 978-1783083367.
- Jakša Cvitanić and Fernando Zapatero (2004). Introduction to the Economics and Mathematics of Financial Markets. MIT Press. ISBN 978-0262033206.
- George M. Constantinides; Milton Harris; René M. Stulz, eds. (2003). Handbook of the Economics of Finance. Elsevier. ISBN 978-0444513632.
- Keith Cuthbertson; Dirk Nitzsche (2004). Quantitative Financial Economics: Stocks, Bonds and Foreign Exchange. Wiley. ISBN 978-0470091715.
- Jean-Pierre Danthine, John B. Donaldson (2005). Intermediate Financial Theory (2nd ed.). Academic Press. ISBN 978-0123693808.
- Louis Eeckhoudt; Christian Gollier, Harris Schlesinger (2005). Economic and Financial Decisions Under Risk. Princeton University Press. ISBN 978-0-691-12215-1.
- Jürgen Eichberger; Ian R. Harper (1997). Financial Economics. Oxford University Press. ISBN 978-0198775409.
- Igor Evstigneev; Thorsten Hens; Klaus Reiner Schenk-Hoppé (2015). Mathematical Financial Economics: A Basic Introduction. Springer. ISBN 978-3319165707.
- Frank J. Fabozzi, Edwin H. Neave and Guofu Zhou (2011). Financial Economics. Wiley. ISBN 978-0470596203.
- Eugene F. Fama and Merton H. Miller (1972). The Theory of Finance (PDF). Holt, Rinehart and Winston. ISBN 0030867320.
- Christian Gollier (2004). The Economics of Risk and Time (2nd ed.). MIT Press. ISBN 978-0-262-57224-8.
- Thorsten Hens and Marc Oliver Rieger (2010). Financial Economics: A Concise Introduction to Classical and Behavioral Finance. Springer. ISBN 978-3540361466.
- Chi-fu Huang and Robert H. Litzenberger (1998). Foundations for Financial Economics. Prentice Hall. ISBN 978-0135006535.
- Jonathan E. Ingersoll (1987). Theory of Financial Decision Making. Rowman & Littlefield. ISBN 978-0847673599.
- Robert A. Jarrow (1988). Finance theory. Prentice Hall. ISBN 978-0133148657.
- Chris Jones (2008). Financial Economics. Routledge. ISBN 978-0415375856.
- Brian Kettell (2002). Economics for Financial Markets. Butterworth-Heinemann. ISBN 978-0-7506-5384-8.
- Yvan Lengwiler (2006). Microfoundations of Financial Economics: An Introduction to General Equilibrium Asset Pricing. Princeton University Press. ISBN 978-0691126319.
- Stephen F. LeRoy; Jan Werner (2000). Principles of Financial Economics. Cambridge University Press. ISBN 978-0521586054.
- Leonard C. MacLean; William T. Ziemba (2013). Handbook of the Fundamentals of Financial Decision Making. World Scientific. ISBN 978-9814417341.
- Antonio Mele (2022). Financial Economics. MIT Press. ISBN 9780262046848.
- Robert C. Merton (1992). Continuous-Time Finance. Blackwell. ISBN 978-0631185086.
- Frederic S. Mishkin (2012). The Economics of Money, Banking, and Financial Markets (3rd ed.). Prentice Hall. ISBN 978-0132961974.
- Harry H. Panjer, ed. (1998). Financial Economics with Applications. Actuarial Foundation. ISBN 978-0938959489.
- Geoffrey Poitras, ed. (2007). Pioneers of Financial Economics. Edward Elgar Publishing. Volume I ISBN 978-1845423810; Volume II ISBN 978-1845423827.
- Richard Roll, ed. (2006). The International Library of Critical Writings in Financial Economics. Cheltenham: Edward Elgar Publishing.
Asset pricing
- Kerry E. Back (2010). Asset Pricing and Portfolio Choice Theory. Oxford University Press. ISBN 978-0195380613.
- Tomas Björk (2009). Arbitrage Theory in Continuous Time (3rd ed.). Oxford University Press. ISBN 978-0199574742.
- John H. Cochrane (2005). Asset Pricing. Princeton University Press. ISBN 978-0691121376.
- Darrell Duffie (2001). Dynamic Asset Pricing Theory (3rd ed.). Princeton University Press. ISBN 978-0691090221.
- Edwin J. Elton; Martin J. Gruber; Stephen J. Brown; William N. Goetzmann (2014). Modern Portfolio Theory and Investment Analysis (9th ed.). Wiley. ISBN 978-1118469941.
- Robert A. Haugen (2000). Modern Investment Theory (5th ed.). Prentice Hall. ISBN 978-0130191700.
- Mark S. Joshi, Jane M. Paterson (2013). Introduction to Mathematical Portfolio Theory. Cambridge University Press. ISBN 978-1107042315.
- Lutz Kruschwitz, Andreas Loeffler (2005). Discounted Cash Flow: A Theory of the Valuation of Firms. Wiley. ISBN 978-0470870440.
- David G. Luenberger (2013). Investment Science (2nd ed.). Oxford University Press. ISBN 978-0199740086.
- Harry M. Markowitz (1991). Portfolio Selection: Efficient Diversification of Investments (PDF) (2nd ed.). Wiley. ISBN 978-1557861085.
- Frank Milne (2003). Finance Theory and Asset Pricing (2nd ed.). Oxford University Press. ISBN 978-0199261079.
- George Pennacchi (2007). Theory of Asset Pricing. Prentice Hall. ISBN 978-0321127204.
- Mark Rubinstein (2006). A History of the Theory of Investments. Wiley. ISBN 978-0471770565.
- William F. Sharpe (1999). Portfolio Theory and Capital Markets: The Original Edition. McGraw-Hill. ISBN 978-0071353205.
Corporate finance
- Jonathan Berk; Peter DeMarzo (2013). Corporate Finance (3rd ed.). Pearson. ISBN 978-0132992473.
- Peter Bossaerts; Bernt Arne Ødegaard (2006). Lectures on Corporate Finance (Second ed.). World Scientific. ISBN 978-981-256-899-1.
- Richard Brealey; Stewart Myers; Franklin Allen (2013). Principles of Corporate Finance. Mcgraw-Hill. ISBN 978-0078034763.
- CFA Institute (2022). Corporate Finance: Economic Foundations and Financial Modeling (3rd ed.). Wiley. ISBN 978-1119743767.
- Thomas E. Copeland; J. Fred Weston; Kuldeep Shastri (2004). Financial Theory and Corporate Policy (4th ed.). Pearson. ISBN 978-0321127211.
- Julie Dahlquist, Rainford Knight, Alan S. Adams (2022). Principles of Finance. OpenStax, Rice University. ISBN 9781951693541.
{{cite book}}
: CS1 maint: multiple names: authors list (link) - Aswath Damodaran (1996). Corporate Finance: Theory and Practice. Wiley. ISBN 978-0471076803.
- João Amaro de Matos (2001). Theoretical Foundations of Corporate Finance. Princeton University Press. ISBN 9780691087948.
- C. Krishnamurti; S. R. Vishwanath (2010). Advanced Corporate Finance. MediaMatics. ISBN 978-8120336117.
- Joseph Ogden; Frank C. Jen; Philip F. O'Connor (2002). Advanced Corporate Finance. Prentice Hall. ISBN 978-0130915689.
- Pascal Quiry; Yann Le Fur; Antonio Salvi; Maurizio Dallochio; Pierre Vernimmen (2011). Corporate Finance: Theory and Practice (3rd ed.). Wiley. ISBN 978-1119975588.
- Stephen Ross; Randolph Westerfield; Jeffrey Jaffe (2012). Corporate Finance (10th ed.). McGraw-Hill. ISBN 978-0078034770.
- Joel M. Stern, ed. (2003). The Revolution in Corporate Finance (4th ed.). Wiley-Blackwell. ISBN 9781405107815.
- Jean Tirole (2006). The Theory of Corporate Finance. Princeton University Press. ISBN 978-0691125565.
- Ivo Welch (2017). Corporate Finance (4th ed.). ISBN 978-0-9840049-2-8.