V1 Saliency Hypothesis
![]() | This article's use of external links may not follow Wikipedia's policies or guidelines. (October 2021) |
The V1 Saliency Hypothesis, or V1SH (pronounced ‘vish’) is a theory[1][2] about V1, the primary visual cortex (V1). It proposes that the V1 in primates creates a saliency map of the visual field to guide visual attention or gaze shifts exogenously.
Importance
[edit]V1SH is the only theory so far to not only endow V1 a very important cognitive function, but also to have provided multiple non-trivial theoretical predictions that have been experimentally confirmed subsequently.[2][3] According to V1SH, V1 creates a saliency map from retinal inputs to guide visual attention or gaze shifts.[1] Anatomically, V1 is the gate for retinal visual inputs to enter neocortex, and is also the largest cortical area devoted to vision. In the 1960s, David Hubel and Torsten Wiesel discovered that V1 neurons are activated by tiny image patches that are large enough to depict a small bar [4] but not a discernible face. This work led to a Nobel prize,[5] and V1 has since been seen as merely serving a back-office function (of image processing) for the subsequent cognitive processing in the brain beyond V1. However, research progress to understand the subsequent processing has been much more difficult or slower than expected (by, e.g., Hubel and Wiesel[6]). Outside the box of the traditional views, V1SH is catalyzing a change of framework[7] to enable fresh progresses on understanding vision.
See
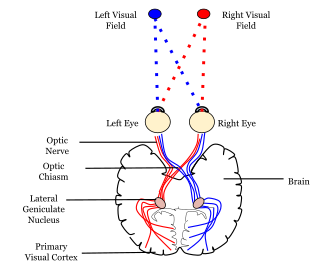
for where primary visual cortex is in the brain and relative to the eyes.
V1SH states that V1 transforms the visual inputs into a saliency map of the visual field to guide visual attention or direction of gaze.[2][1] Humans are essentially blind to visual inputs outside their window of attention. Therefore, attention gates visual perception and awareness, and theories of visual attention are cornerstones of theories of visual functions in the brain.
A saliency map is by definition computed from, or caused by, the external visual input rather than from internal factors such as animal’s expectations or goals (e.g., to read a book). Therefore, a saliency map is said to guide attention exogenously rather than endogenously. Accordingly, this saliency map is also called the bottom-up saliency map to guide reflexive or involuntary shifts of attention. For example, it guides our gaze shifts towards an insect flying in our peripheral visual field when we are reading a book. Note that this saliency map, which is constructed by a biological or natural brain, is not the same as the sort of saliency map that is engineered in artificial or computer vision, partly because the artificial saliency maps often include attentional guidance factors that are endogenous in nature.
In this (biological) saliency map of the visual field, each visual location has a saliency value. This value is defined as the strength of this location to attract attention exogenously.[2] So if location A has a higher saliency value than location B, then location A is more likely to attract visual attention or gaze shifts towards it than location B. In V1, each neuron can be activated only by visual inputs in a small region of the visual field. This region is called the receptive field of this neuron, and typically covers no more than the size of a coin at an arm’s length.[8] Neighbouring V1 neurons have neighbouring and overlapping receptive fields.[8] Hence, each visual location can simultaneously activate many V1 neurons. According to V1SH, the most activated neuron among these neurons signals the saliency value at this location by its neural activity.[1][2] A V1 neuron’s response to visual inputs within its receptive field is also influenced by visual inputs outside the receptive field.[9] Hence saliency value at each location depends on visual input context.[1][2] This is as it should be since saliency depends on context. For example, a vertical bar is salient in an image in which all the other visual items surrounding it are horizontal bars, but this same vertical bar is not salient if these other items are all vertical bars instead.
Neural mechanisms in V1 to generate the saliency map
[edit]
The figure above gives a schematics of the neural mechanisms in V1 to generate the saliency map. In this example, the retinal image has many purple bars, all uniformly oriented (right-tilted) except for one bar that is oriented uniquely (left-tilted). This orientation singleton is the most salient in this image, so it attracts attention or gaze, as observed in psychological experiments.[10] In V1, many neurons have their preferred orientations for visual inputs.[8] For example, a neuron's response to a bar in its receptive field is higher when this bar is oriented in its preferred orientation. Analogously, many V1 neurons have their preferred colours.[8] In this schematic, each input bar to the retina activates two (groups of) V1 neurons, one preferring its orientation and the other preferring its colour. The responses from neurons activated by their preferred orientations in their receptive fields are visualized in the schematics by the black dots in the plane representing the V1 neural responses. Similarly, responses from neurons activated by their preferred colours in their receptive fields are visualized by the purple dots. The sizes of the dots visualize the strengths of the V1 neural responses. In this example, the largest response comes from the neurons preferring and responding to the uniquely oriented bar. This is because of iso-orientation suppression: when two V1 neurons are near each other and have the same or similar preferred orientations, they tend to suppress each other’s activities.[9][11] Therefore, among the group of neurons that prefer and respond to the uniformly oriented background bars, each neuron receives iso-orientation suppression from other neurons of this group.[1][9] Meanwhile, the neuron responding to the orientation singleton does not belong to this group and thus escapes this suppression,[1] hence its response is higher than the other neural responses. Iso-colour suppression[12] is analogous to iso-orientation suppression, so all neurons preferring and responding to the purple colours of the input bars are under the iso-colour suppression. According to V1SH, the maximum response at each bar’s location represents the saliency value at each bar’s location.[1][2] This saliency value is thus highest at the location of the orientation singleton, and is represented by the response from neurons preferring and responding to the orientation of this singleton. These saliency values are sent to the superior colliculus,[13] a midbrain area, to execute gaze shifts to the receptive field of the most activated neuron responding to visual input space.[13] Hence, for this input image in the figure above, the orientation singleton, which evokes the highest V1 response to this image, attracts visual attention or gaze.
V1SH explains behavioral data on visual search/segmentation
[edit]V1SH can explain data on visual search, such as the short response times to find a uniquely red item among green items, or a uniquely vertical bar among horizontal bars, or an item uniquely moving to the right among items moving to the left. These kind of visual searches are called feature searches, when the search target is unique in a basic feature value like orientation, color, or motion direction.[10][14] The shortness of the search response time manifests a higher saliency value at the location of the search target to attract attention. V1SH also explains why it takes longer to find a unique red-vertical bar among red-horizontal bars and green-vertical bars. This is an example of conjunction searches when the search target is unique only by the conjunction of two features, each of which is present in the visual scene.[10]

Furthermore, V1SH explains data that are difficult to be explained by alternative frameworks.[10][15] The figure above illustrates an example: two neighboring textures in A, one made of uniformly left-tilted bars and another of uniformly right-tilted bars, are very easy to be segmented from each other by human vision. This is because the texture bars at the border between the two textures evoke the highest V1 neural responses (since they are least suppressed by iso-orientation suppression), therefore, the border bars are the most salient in the image to attract attention to the border. However, the segmentation becomes much more difficult if the texture in B is superposed on the original image in A (the result is depicted in C). This is because, at non-border texture locations, V1 neural responses to the horizontal and vertical bars (from B) are higher than those to the oblique bars (from A); these higher responses dictate and raise the saliency values at these non-border locations, making the border no longer as competitive for saliency.[16]
Impact
[edit]
V1SH was proposed in late 1990's[17][18] by Li Zhaoping. It was uninfluential initially since for decades it has been believed that attentional guidance is essentially or only controlled by higher-level brain areas. These higher-level brain areas include the frontal eye field and parietal cortical areas[19] in the frontal and more anterior part of the brain, and they are believed to be intelligent for attentional and executive control. In addition, the primary visual cortex, V1, located in occipital lobe in the back or posterior part of the brain, has traditionally been thought of as a low-level visual area that plays mainly a supporting role to other brain areas for their more important visual functions.[8] Opinions started to change by a surprising piece of behavioral data: an item uniquely shown to one eye --- an ocular singleton --- among similarly appearing items shown to the other eye (using e.g. a pair of glasses for watching 3D movies) can attract gaze or attention automatically.[20][21] An example is illustrated in this figure. Here, an image containing a single letter 'X' is shown to the right eye, and another image containing an array of the same 'X's and a letter 'O' is shown to the left eye. In such a situation, human observers normally perceive an image resembling a superposition of the two monocular images, such that they see an array of all the 'X's and the single 'O'. The 'X' arising from the right-eye image will not appear distinctive. Nevertheless, even when they are doing a task to search (in their perceived image) for the unique and perceptually distinctive 'O' as quickly as possible, their gaze automatically or involuntarily shifts to the 'X' arising from the right-eye image, often before their gaze shifts to the 'O'. Attention capture by such an ocular singleton occurs even when observers fail to guess whether this singleton is present (if it were absent in this example figure, all 'X's and the single 'O' would be shown to the left eye only).[20] This observation was counter-intuitive,[22] was easily reproduced by other vision researchers, and was uniquely predicted by V1SH. Since V1 is the only visual cortical area with neurons tuned to eye of origin of visual inputs,[4] this observation strongly supports V1's role in guiding attention.
More experiments followed to further investigate V1SH,[2] and supporting data emerged from functional brain imaging,[23] visual psychophysics,[24][25] and from monkey electrophysiology[3][26][27][28] (although see some conflicting data[29]). V1SH has since become more popular.[30][31] V1 is now seen as one of the corner stones in the brain's network of attentional mechanisms,[32][33] and its functional role in guiding visual attention is appearing in handbooks[34][35] and textbooks.[36][37] Zhaoping argues that If V1SH is correct, the ideas[38][39] about how visual system works, and consequently questions to ask for future vision research, should be fundamentally changed.[7]
References
[edit]- ^ a b c d e f g h Li, Zhaoping (2002-01-01). "A saliency map in primary visual cortex". Trends in Cognitive Sciences. 6 (1): 9–16. doi:10.1016/S1364-6613(00)01817-9. ISSN 1364-6613. PMID 11849610. S2CID 13411369.
- ^ a b c d e f g h Zhaoping, Li (2014). The V1 hypothesis—creating a bottom-up saliency map for preattentive selection and segmentation. Oxford University Press. ISBN 978-0-19-177250-4.
- ^ a b Yan, Yin; Zhaoping, Li; Li, Wu (2018-10-09). "Bottom-up saliency and top-down learning in the primary visual cortex of monkeys". Proceedings of the National Academy of Sciences. 115 (41): 10499–10504. Bibcode:2018PNAS..11510499Y. doi:10.1073/pnas.1803854115. ISSN 0027-8424. PMC 6187116. PMID 30254154.
- ^ a b Hubel, D. H.; Wiesel, T. N. (Jan 1962). "Receptive fields, binocular interaction and functional architecture in the cat's visual cortex". The Journal of Physiology. 160 (1): 106–154.2. doi:10.1113/jphysiol.1962.sp006837. ISSN 0022-3751. PMC 1359523. PMID 14449617.
- ^ "The Nobel Prize in Physiology or Medicine 1981". NobelPrize.org. Retrieved 2021-10-10.
- ^ Hubel, David; Wiesel, Torsten (2012-07-26). "David Hubel and Torsten Wiesel". Neuron. 75 (2): 182–184. doi:10.1016/j.neuron.2012.07.002. ISSN 0896-6273. PMID 22841302. S2CID 12766897.
- ^ a b Zhaoping, Li (2019-10-01). "A new framework for understanding vision from the perspective of the primary visual cortex". Current Opinion in Neurobiology. Computational Neuroscience. 58: 1–10. doi:10.1016/j.conb.2019.06.001. ISSN 0959-4388. PMID 31271931. S2CID 195806018.
- ^ a b c d e "The Primary Visual Cortex by Matthew Schmolesky – Webvision". Retrieved 2020-07-05.
- ^ a b c Knierim, J. J.; van Essen, D. C. (April 1992). "Neuronal responses to static texture patterns in area V1 of the alert macaque monkey". Journal of Neurophysiology. 67 (4): 961–980. doi:10.1152/jn.1992.67.4.961. ISSN 0022-3077. PMID 1588394.
- ^ a b c d Treisman, Anne M.; Gelade, Garry (1980-01-01). "A feature-integration theory of attention". Cognitive Psychology. 12 (1): 97–136. doi:10.1016/0010-0285(80)90005-5. ISSN 0010-0285. PMID 7351125. S2CID 353246.
- ^ Allman, J.; Miezin, F.; McGuinness, E. (1985). "Stimulus specific responses from beyond the classical receptive field: neurophysiological mechanisms for local-global comparisons in visual neurons". Annual Review of Neuroscience. 8: 407–430. doi:10.1146/annurev.ne.08.030185.002203. ISSN 0147-006X. PMID 3885829.
- ^ Wachtler, Thomas; Sejnowski, Terrence J.; Albright, Thomas D. (2003-02-20). "Representation of color stimuli in awake macaque primary visual cortex". Neuron. 37 (4): 681–691. doi:10.1016/s0896-6273(03)00035-7. ISSN 0896-6273. PMC 2948212. PMID 12597864.
- ^ a b Schiller, Peter H. (1988), Held, Richard (ed.), "Colliculus, Superior", Sensory System I: Vision and Visual Systems, Readings from the Encyclopedia of Neuroscience, Boston, MA: Birkhäuser, p. 9, doi:10.1007/978-1-4899-6647-6_6, ISBN 978-1-4899-6647-6, retrieved 2020-07-05
- ^ Wolfe, Jeremy. "Visual Search". psycnet.apa.org. Retrieved 2020-07-11.
- ^ Itti, L.; Koch, C. (Mar 2001). "Computational modelling of visual attention" (PDF). Nature Reviews. Neuroscience. 2 (3): 194–203. doi:10.1038/35058500. ISSN 1471-003X. PMID 11256080. S2CID 2329233.
- ^ Zhaoping, Li; May, Keith A. (2007-04-06). "Psychophysical Tests of the Hypothesis of a Bottom-Up Saliency Map in Primary Visual Cortex". PLOS Computational Biology. 3 (4): e62. Bibcode:2007PLSCB...3...62Z. doi:10.1371/journal.pcbi.0030062. ISSN 1553-7358. PMC 1847698. PMID 17411335.
- ^ Li, Zhaoping (1999-08-31). "Contextual influences in V1 as a basis for pop out and asymmetry in visual search". Proceedings of the National Academy of Sciences. 96 (18): 10530–10535. Bibcode:1999PNAS...9610530L. doi:10.1073/pnas.96.18.10530. ISSN 0027-8424. PMC 17923. PMID 10468643.
- ^ Li, Zhaoping (1998). "Primary cortical dynamics for visual grouping " as a book chapter in "Theoretical Aspects of Neural Computation", Eds K.M. Wong, I. King, and D.Y. Yeung. Springer-verlag. pp. 155–164.
- ^ Desimone, Robert; Duncan, John (Mar 1995). "Neural Mechanisms of Selective Visual Attention". Annual Review of Neuroscience. 18 (1): 193–222. doi:10.1146/annurev.ne.18.030195.001205. ISSN 0147-006X. PMID 7605061.
- ^ a b Zhaoping, Li (2008-05-01). "Attention capture by eye of origin singletons even without awareness—A hallmark of a bottom-up saliency map in the primary visual cortex". Journal of Vision. 8 (5): 1.1–18. doi:10.1167/8.5.1. ISSN 1534-7362. PMID 18842072.
- ^ Zhaoping, Li (2012-02-01). "Gaze capture by eye-of-origin singletons: Interdependence with awareness". Journal of Vision. 12 (2): 17. doi:10.1167/12.2.17. ISSN 1534-7362. PMID 22344346.
- ^ Zhaoping, Li (2014-08-21). "Are we too "smart" to understand how we see?". OUPblog. Retrieved 2020-07-11.
- ^ Zhang, Xilin; Zhaoping, Li; Zhou, Tiangang; Fang, Fang (2012-01-12). "Neural Activities in V1 Create a Bottom-Up Saliency Map". Neuron. 73 (1): 183–192. doi:10.1016/j.neuron.2011.10.035. ISSN 0896-6273. PMID 22243756. S2CID 9767861.
- ^ Koene, Ansgar R.; Zhaoping, Li (2007-05-23). "Feature-specific interactions in salience from combined feature contrasts: evidence for a bottom-up saliency map in V1" (PDF). Journal of Vision. 7 (7): 6.1–14. doi:10.1167/7.7.6. ISSN 1534-7362. PMID 17685802.
- ^ Kennett, Matthew J.; Wallis, Guy (2019-07-01). "The face-in-the-crowd effect: Threat detection versus iso-feature suppression and collinear facilitation". Journal of Vision. 19 (7): 6. doi:10.1167/19.7.6. ISSN 1534-7362. PMID 31287860. S2CID 195871013.
- ^ Wagatsuma, Nobuhiko; Hidaka, Akinori; Tamura, Hiroshi (2021-01-12). "Correspondence between Monkey Visual Cortices and Layers of a Saliency Map Model Based on a Deep Convolutional Neural Network for Representations of Natural Images". eNeuro. 8 (1). doi:10.1523/ENEURO.0200-20.2020. ISSN 2373-2822. PMC 7890521. PMID 33234544.
- ^ Klink, P. Christiaan; Teeuwen, Rob R. M.; Lorteije, Jeannette A. M.; Roelfsema, Pieter R. (2023-02-28). "Inversion of pop-out for a distracting feature dimension in monkey visual cortex". Proceedings of the National Academy of Sciences. 120 (9). doi:10.1073/pnas.2210839120. ISSN 0027-8424. PMC 9992771. PMID 36812207.
- ^ Westerberg, Jacob A.; Schall, Jeffrey D.; Woodman, Geoffrey F.; Maier, Alexander (2023-09-26). "Feedforward attentional selection in sensory cortex". Nature Communications. 14 (1): 5993. doi:10.1038/s41467-023-41745-1. ISSN 2041-1723. PMC 10522696.
- ^ White, Brian J.; Kan, Janis Y.; Levy, Ron; Itti, Laurent; Munoz, Douglas P. (2017-08-29). "Superior colliculus encodes visual saliency before the primary visual cortex". Proceedings of the National Academy of Sciences of the United States of America. 114 (35): 9451–9456. doi:10.1073/pnas.1701003114. ISSN 1091-6490. PMC 5584409. PMID 28808026.
- ^ "Visual Perception meets Computational Neuroscience | www.ecvp.uni-bremen.de". www.ecvp.uni-bremen.de. Retrieved 2020-07-11.
- ^ "CNS 2020". www.cnsorg.org. Retrieved 2021-06-27.
- ^ Bisley, James W.; Goldberg, Michael E. (June 2010). "Attention, Intention, and Priority in the Parietal Lobe". Annual Review of Neuroscience. 33 (1): 1–21. doi:10.1146/annurev-neuro-060909-152823. ISSN 0147-006X. PMC 3683564. PMID 20192813.
- ^ Shipp, Stewart (2004-05-01). "The brain circuitry of attention". Trends in Cognitive Sciences. 8 (5): 223–230. doi:10.1016/j.tics.2004.03.004. ISSN 1364-6613. PMID 15120681. S2CID 459078.
- ^ Nobre, Anna C. (Kia); Kastner, Sabine, eds. (2014-01-01). The Oxford Handbook of Attention. Oxford University Press. doi:10.1093/oxfordhb/9780199675111.001.0001. ISBN 978-0-19-175301-5.
- ^ Wolfe, Jeremy M. (2018), "Visual Search", Stevens' Handbook of Experimental Psychology and Cognitive Neuroscience, American Cancer Society, pp. 1–55, doi:10.1002/9781119170174.epcn213, ISBN 978-1-119-17017-4, retrieved 2021-06-24
- ^ "Visual Attention and Consciousness". Routledge & CRC Press. Retrieved 2021-06-24.
- ^ Zhaoping, Li (2014-05-08). Understanding Vision: Theory, Models, and Data. Oxford, New York: Oxford University Press. ISBN 978-0-19-956466-8.
- ^ "Webvision – The Organization of the Retina and Visual System". Retrieved 2020-07-05.
- ^ Stone, James (14 September 2012). Vision and Brain. MIT Press. ISBN 9780262517737. Retrieved 2020-07-05.